Jin-qiang CHANG, Ruo-yu ZHANG, Yu-jie PANG, Meng-yun ZHANG, Ya ZHA. Classification of Impurities in Machine-Harvested Seed Cotton Using Hyperspectral Imaging[J]. Spectroscopy and Spectral Analysis, 2021, 41(11): 3552

Search by keywords or author
- Spectroscopy and Spectral Analysis
- Vol. 41, Issue 11, 3552 (2021)
Abstract
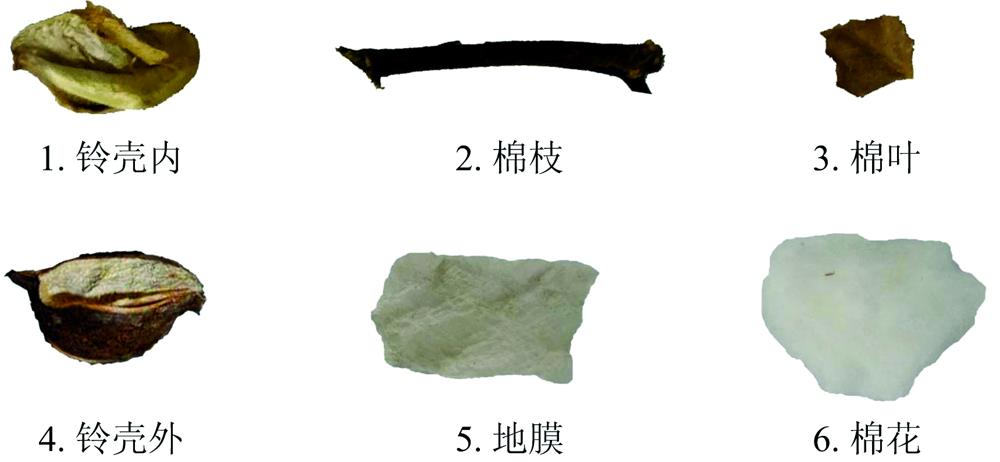
Set citation alerts for the article
Please enter your email address