
- Journal of Geographical Sciences
- Vol. 30, Issue 8, 1363 (2020)
Abstract
Keywords
1 Introduction
The border areas of the Tibetan Plateau and the adjacent mountainous areas have a high incidence of earthquakes of magnitude greater than
At present, much research has been done in the area of post-disaster economic recovery. Most researchers have analyzed post-disaster economic shock and the recovery process by comparing the index changes before and after a disaster with the input-output coefficient, economic aggregate, economic growth rate, industrial structure, resident income, and the number of enterprises. The existing theoretical exploration of and empirical research on post-disaster economic recovery includes two main components.
(1) The methods of post-disaster economic loss assessment are first applied to the direct and indirect economic loss assessment of natural disasters such as earthquakes and severe meteorological events, e.g., transforming the traditional input-output coefficient into the productivity loss coefficient to reflect the economic impact (
(2) With the concept of economic resilience, the econometric model and index system are used to evaluate the resilience of the post-disaster economy on the basis of discussing the growth model of the regional economy following the disaster (
Existing studies, however, have paid more attention to the damaged state of the economy in the short term following a disaster, and there is a lack of quantitative analysis of the long-term economic recovery process and economic resilience. Research on the economic recovery effect and efficiency following natural disasters is also lacking. In addition, it is difficult to fully reflect the regional characteristics and influence of economic resilience and recovery efficiency by studying the economic recovery process in disaster areas as a whole. Consequently, this paper defines post-disaster economic resilience as the ability of the economic system in the disaster area to withstand and defuse the impact of earthquake disasters, which is characterized by both static and dynamic states. The static state emphasizes the robustness of the economic system during the earthquake impacts, while the dynamic state focuses on the rapidity and readability of long-term recovery from the impacts. Taking the areas most severely affected by the Wenchuan Earthquake as an example, according to the socioeconomic data of 10 disaster-hit counties and the post-disaster economic prediction results based on the differential autoregressive integrated moving average (ARIMA) model, the index of economic resilience of the border areas of the Tibetan Plateau impacted by the Wenchuan Earthquake was calculated. Then, an improved variable returns to scale (VRS) date envelopment analysis (DEA) model and the Malmquist productivity index were used to analyze the short-term and long-term economic recovery efficiency and its influence. This lays a scientific foundation for the assessment of post-disaster economic risks and the improvement in economic resilience in disaster-hit areas, as well as providing refined decision-making suggestions for the enhancement of natural disaster prevention and system response capabilities in the border areas of the Tibetan Plateau.
2 Research methods and data sources
2.1 Case study area and data sources
According to the assessment results for the scope of the disaster following the
Figure 1.
In terms of data collection and processing, taking the counties (cities) as the basic unit and 1994, the starting year with complete statistical data, as the base period, the constant prices of GDP, per capita GDP, the output values of the three strata of industry were converted by indices of GDP, the relative numbers reflecting the trend and degree of GDP change compared with the previous year at comparable prices. Since the capital stock data for the severely affected areas could not be directly obtained, in this paper the capital investment index of Sichuan Province was adopted to deflate the price of the total social fixed assets investment in the severely affected areas, and then the international perpetual inventory method was adopted to convert the flow of fixed assets into capital stock. The capital stock data calculated by the existing estimation methods were used as the base period capital stock, and the depreciation rate was set at a constant rate of 9.6% (
2.2 Research methods
2.2.1 ARIMA prediction model
The ARIMA model, whose principle function is to transform a nonstationary time series into a stationary one and then carry out a regression analysis of each lag period of the dependent variable, the present value of the random error term, and each lag period of the random error term (
where
2.2.2 Measurement model of post-disaster economic resilience
Based on the growth trajectory and a model of the regional economy following external shock events,
where the time period of
Figure 2.
2.2.3 Improved DEA model with VRS
The DEA model with VRS was selected to evaluate the relative efficiency of economic recovery in the short term following the earthquake. Taking a severely affected county (city) as the decision-making unit (DMU), the number of units is
Based on the DEA model of constant returns to scale (CRS), the VRS model was established by introducing the constraints:$\underset{n=1}{\overset{N}{\mathop \sum }}\,=1$, where
2.2.4 Malmquist productivity index model
In order to further understand the interannual variation in the long-term economic recovery efficiency in disaster areas, the Malmquist productivity index model was adopted. The total factor productivity was decomposed into changes in technological progress, pure technical efficiency, and scale efficiency.
where ${D}'({x}',{y}')$ and ${D}'({{x}^{t+1}},{{y}^{t+1}})$ refer to the distance function of the DMU in period
When the Malmquist productivity index is greater than 1, the total factor level in the
3 Results
3.1 Post-disaster economic resilience
3.1.1 Economic recovery cycle
Before the Wenchuan Earthquake, the 10 counties and cities in the most severely affected areas had maintained an average annual growth rate of more than 10%. Following the 2008 earthquake, the total GDP decreased from 52.787 billion yuan in 2007 to 38.546 billion yuan, a decrease of 26.98%. Of the 10 counties, Wenchuan County, where the epicenter was located, had the highest degree of recession (
Figure 3.
3.1.2 Overall and sectoral economic resilience
Based on the value-added data for the three strata of industry from 1994 to 2007, the ARIMA model was adopted to predict the GDP and growth trajectory of these three strata under the condition of no earthquake (
Figure 4.
As shown in
Figure 5.
3.1.3 Economic readjustment process
A comparison of the model predictions for the post-earthquake economy indicates that the post-earthquake economy was able to undergo structural readjustment and was relatively resilient to the crisis (
Figure 6.
At the same time, the post-earthquake economic transformation has significantly boosted the level of economic development in the most severely affected areas. In the three years following 2009, the per capita GDP growth rates in the disaster areas were 22.28%, 20.05%, and 19.29%, respectively. By 2010, the levels of per capita GDP in the most severely affected areas had recovered to the absolute levels in the year before the earthquake, and by 2015, it had doubled compared with that before the earthquake. Moreover, post-earthquake development opportunities and industrial restructuring have narrowed the gaps between the different levels of economic development of the different counties. From the coefficient of variation (CV value) of per capita GDP over the years, it was found that the trend for widening of the economic gaps between the post-earthquake disaster areas has been reversed. The CV value remained above 0.7 in the five years before the earthquake, but decreased to 0.65 in the five years following the earthquake (
Figure 7.
3.2 Efficiency of post-disaster economic recovery
To measure the efficiency of post-disaster economic recovery from the perspective of input and output, it should be pointed out that when the DEA model is used for evaluation, it is generally stipulated that the sum of input and output indicators is less than or equal to one-third (⅓) of the number of evaluation units (
3.2.1 Short-term economic recovery efficiency based on the VRS model
Based on the input and output indicators of the most severely affected areas in 2008, the VRS model was adopted for evaluation, and the results are shown in
Area | Comprehensive | Pure technical | Scale efficiency | Returns to scale |
---|---|---|---|---|
Wenchuan | 0.505 | 1.000 | 0.505 | Increase |
Beichuan | 0.293 | 1.000 | 0.293 | Increase |
Mianzhu | 1.000 | 1.000 | 1.000 | Constant |
Shifang | 1.000 | 1.000 | 1.000 | Constant |
Qingchuan | 0.321 | 1.000 | 0.321 | Increase |
Maoxian | 0.269 | 1.000 | 0.269 | Increase |
Dujiangyan | 0.912 | 0.939 | 0.971 | Decrease |
Pingwu | 0.299 | 0.580 | 0.515 | Increase |
Pengzhou | 0.637 | 1.000 | 0.637 | Decrease |
Anxian | 0.798 | 0.981 | 0.813 | Increase |
Average value | 0.603 | 0.950 | 0.632 | — |
Table 1.
Relative efficiency of economic recovery and its decomposition results for 2008
The comprehensive efficiency of economic recovery in the most severely affected areas in 2008 was 0.603, which was only 60.3% of the optimal level. Only two cities in the most severely affected areas, Mianzhu City and Shifang City, achieved DEA effectiveness, and both the technology efficiency and the scale efficiency were 100% of the optimal level, indicating that the economic input factors were reasonably combined and allocated and that the post-earthquake economic recovery efficiency was at a relatively advanced level in the most severely affected areas. The other eight counties and cities did not achieve DEA effectiveness, and their economic input has not been fully utilized because of the disaster. According to the decomposition of pure technical efficiency and scale efficiency, the pure technical efficiency of economic recovery in the most severely affected areas was 0.950, 95.0% of the optimal level. Six counties and cities, including Mianzhu, Shifang, Pengzhou, Wenchuan, Maoxian, and Beichuan, achieved technical efficiency, while the other four counties and cities were in an inefficient state of pure technical efficiency. The scale efficiency of economic recovery in the most severely affected areas was 0.632, 63.2% of the optimal level. Of these areas, only Mianzhu and Shifang were effective in scale efficiency, and remained unchanged, while the other counties and cities were ineffective in scale efficiency, and mainly increased in scale efficiency.
The comprehensive efficiency, pure technical efficiency, and scale efficiency of short-term economic recovery in the plain and hilly areas were significantly higher than those in the plateau and mountain areas. The average values for the former areas were 0.869, 0.984, and 0.884 respectively, while those for the latter areas were 0.337, 0.916, and 0.381 respectively. This indicates that the strong earthquake had an impact on the production efficiency affected by management, technology, and input scale factors in the plateau and mountain areas, which led to weak economic resilience in the short term following the earthquake. Among the counties, the comprehensive efficiencies of Beichuan, Qingchuan, Maoxian, and Pingwu counties were less than 40% of the optimal level. A correlation test was conducted of comprehensive efficiency index, per capita GDP, and industrialization level. The results show that there was a significant positive correlation between per capita GDP, industrialization level, and economic recovery efficiency (confidence level was 0.05). The Pearson correlation coefficients were 0.884 and 0.537, respectively, indicating that regions with higher levels of economic development and industrialization had greater economic resilience, while poor counties were less resilient to earthquake disasters.
3.2.2 Medium- and long-term economic recovery efficiency based on the Malmquist TFP
The Malmquist total factor productivity (TFP) index of post-disaster economic recovery from 2000 to 2015, along with its decomposition results, were calculated using DEAP2.1 software. The interannual trend of TFP (
Figure 8.
(1) In the two years following the earthquake, the TFP fluctuated considerably, and the economic recovery efficiency decreased significantly, resulting in short-term economic recession.
In the two years following the earthquake, the TFP index values in the most severely affected areas were 0.663 and 0.848, respectively, and TFP declined by 33.7% and 15.2%, respectively. In the five years since 2010, TFP in the most severely affected areas rebounded and returned to a steady state. The TFP index values were 1.041, 1.015, 1.032, 1.03, and 1.036, respectively, maintaining an average annual growth rate of 3%. In general, the Wenchuan Earthquake caused severe fluctuations in TFP in the most severely affected areas, and the significant decline in economic output efficiency following the earthquake resulted in a short-term economic recession. However, following the two-year recession, the most severely affected areas gradually shifted from high-speed rebound to stable growth.
(2) The rate of technical change was strongly affected by the earthquake, and the renewal of the production system was the leading factor in determining economic recovery following the earthquake.
From the TFP decomposition results (
Year | Comprehensive efficiency | Rate of technological change | Pure technical efficiency | Scale efficiency | Total factor growth rate |
---|---|---|---|---|---|
2007 | 0.924 | 1.131 | 0.974 | 0.948 | 1.044 |
2008 | 1.027 | 0.646 | 1.053 | 0.975 | 0.663 |
2009 | 0.957 | 0.886 | 0.854 | 1.121 | 0.848 |
2010 | 1.011 | 1.030 | 0.988 | 1.023 | 1.041 |
2011 | 0.930 | 1.092 | 1.012 | 0.919 | 1.015 |
2012 | 0.993 | 1.039 | 1.060 | 0.937 | 1.032 |
2013 | 1.019 | 1.011 | 1.069 | 0.953 | 1.030 |
2014 | 1.037 | 0.999 | 1.029 | 1.007 | 1.036 |
2015 | 1.037 | 0.977 | 0.991 | 1.047 | 1.014 |
Table 2.
Malmquist index and its decomposition results since 2007
(3) Following the earthquake, the comprehensive economic efficiency improved steadily, and the pure technical efficiency and scale efficiency improved alternately.
The comprehensive efficiency did not show the same strong fluctuations as TFP and the technology change index. In 2008 and 2009, the values of the index of comprehensive efficiency change for the most severely affected areas were 1.027 and 0.957, respectively, which then maintained a stable increasing trend of less than 1% annually. It is worth noting that with the progress of recovery time, the improvement in comprehensive efficiency has pulled on TFP, which was an important driving force for long-term economic recovery and development following the earthquake. Then, the comprehensive efficiency was decomposed into pure technical efficiency and scale efficiency under VRS. As shown in
(4) Following the earthquake, the economic efficiency of the plateau and mountain areas decreased significantly, and resilience was lower in those areas than in the plain and hilly areas in the short term.
According to the post-earthquake changes in different regions, in 2008 the Malmquist index values of the plateau and mountain areas and the plain and hilly areas were 0.598 and 0.747, respectively, that is, decreases of 40.18% and 25.26%, respectively, compared with 2007. The results show that the economic efficiency of plateau and mountain areas decreased significantly and their economic resilience was lower than that of the plain and hilly areas. Further analysis shows that following the earthquake, the technical change index values of the plateau and mountain areas and the plain and hilly areas decreased by 35.18% and 35.64%, respectively. However, due to the difference in the comprehensive economic efficiency, that is, the plain and hilly areas were significantly better (1.161) than the plateau and mountain areas (0.924) in terms of their comprehensive economic efficiency, resulting in the relatively high resilience of the plain and hilly areas in the short term. It can be seen that when post-earthquake technology and production systems are severely damaged, the plain and hilly areas have obvious advantages in terms of economic management, resource allocation, and other aspects. Therefore, the economic efficiency of those areas is higher than that of the plateau and mountain areas, which can have technical benefits and economies of scale in the short term, and the total factor growth rate can increase rapidly as a result.
4 Conclusions and implications
Based on the predictions of the ARIMA model, this paper has calculated the index of economic resilience in the most severely affected areas following the Wenchuan Earthquake of 2008, and has revealed the robustness, rapidity, and adaptability of economic recovery in the border areas of the Tibetan Plateau following the disaster. In addition, using the VRS model and the Malmquist productivity index evaluation, the short-term and long-term economic recovery efficiencies and impact effects have been analyzed. The four main conclusions and policy implications are described below.
(1) The economy in the areas most severely affected by the Wenchuan Earthquake is relatively resilient. The earthquake led to a short-term economic recession, but the economy recovered to the pre-earthquake level within two years. The economic resilience indexes of GDP and the three strata of industry were 0.877, 0.903, 0.781, and 1.07, respectively, among which the economic resilience index values for agriculture and the service industry were higher than that of manufacturing industry. The economy of the region showed a certain resilience following the quake. The capital, human resources, and material inputs for construction assistance have stimulated the structural readjustment of industries in the most severely affected areas, have brought about a significant boost to regional economic development, and have narrowed the gap in the level of economic development within the most severely affected areas. By 2010, per capita GDP had recovered to the pre-earthquake level, and by 2015 it had basically doubled from that before the earthquake. The coefficient of variation of per capita GDP in the most severely affected areas also decreased, from above 0.7 in the five years before the quake to 0.65 in the five years following the quake.
(2) In 2008, the comprehensive efficiency of economic recovery in the disaster areas was only 60.3% of the optimal level. The comprehensive efficiency, pure technical efficiency, and scale efficiency of short-term economic recovery in the plain and hilly areas were significantly higher than those in the plateau and mountain areas. The powerful earthquake had an impact on production efficiency, and was affected by factors such as management and technology, as well as by factors of input scale, resulting in weak economic resilience in the short term following the earthquake. From the perspective of interannual changes, TFP fluctuated strongly following the earthquake. The TFP index in the disaster areas decreased by 33.7% and 15.2%, respectively, in 2009 and 2010, and then maintained an average annual growth rate of 3%. The significant decline in the post-earthquake TFP index is due mainly to technological changes, and the updating of the production system was the leading factor in determining post-earthquake economic resilience, which is also the important reason why the post-earthquake economic resilience of the plateau and mountain areas was weaker than that of the plain and hilly areas. It was also found that, with the decline in economic scale, long-term economic recovery in the disaster areas depends mainly on pure technical efficiency.
(3) The study shows that although government financial input and external counterpart assistance, including emergency and transitional assistance, are the keys to the rapid recovery of the post-disaster economic order in the border areas of the Tibetan Plateau, it is endogenous technological progress that plays the decisive role in the resilience and recovery efficiency of the post-disaster economy. To achieve a better recovery effect, on the one hand, the difference between natural conditions and the stage of economic development should be taken into account. The disaster areas themselves need to actively adjust and reform the economic structure. On the other hand, regarding top-down support and government financial assistance, it is necessary to pay attention to the upgrading of the production system in the disaster areas to improve the level of technological progress. Only in this way can the scale effect of large-scale capital, new facilities, human resources, and other inputs be exerted in order to enhance the economic resilience and recovery efficiency in responding to the impact of natural disasters. Therefore, the enlightened policies that would enhance the resilience of the post-disaster economy can be summarized as follows: (i) full play should be given to the initiative of self-reliance of people who live in the disaster areas, enhance the self-development capacity of economic recovery in the long run, and take the post-disaster economic recovery as an opportunity to optimize the industrial structure and adjust the industrial layout. Guided by the market, and based on the carrying capacity of resources and the environment, industrial policies, and employment needs, scientific guidance should be given to disaster-hit enterprises to rebuild, relocate, close down, or turn around and support the recovery and development of industries with distinctive strengths; (ii) while restoring the hardware systems in areas of urban and rural housing, infrastructure, and public service facilities, a focus should also be maintained on the re-engineering of software systems such as skills training, employment assistance, investment attraction, and park operation and management. Through the co-construction of industrial parks, work-for-relief, industrial cooperation projects, and other forms of industrial counterpart assistance and cooperation, the ability of workers in disaster-hit areas to find new jobs and transfer jobs would be increased, thereby simultaneously improving the hardware- and software-supporting capabilities for post-disaster economic development; (iii) new ways should be developed to invest both government funds and market capital, a disaster prevention and reduction reserve fund, a special fund for post-disaster reconstruction, a counterpart support fund, a natural disaster risk investment fund, government bonds, disaster relief lottery, etc. should all be established. A government-led, multi-source investment and risk-sharing investment guarantee system and risk-sharing mechanism should be established to make society more resilient as a whole, and, particular, resilient to natural disasters.
(4) This paper explores the post-earthquake economic recovery process and its efficiency in the border areas of the Tibetan Plateau. Limited by difficulties in obtaining data, however, only the total economic volume and the three strata of industry were selected for comparative analysis. In fact, the preliminary research conclusions of this paper have shown that there were significant differences in the recovery efficiency and change trends of different industries following the earthquake. Therefore, supported by large-scale funds, new facilities, human resources, and other assistance, more thorough studies of the post-disaster recovery process and differences in the input-output efficiency of various industries need to be undertaken. Moreover, this study also found that the degree of mixing of old and new capital affects economic recovery efficiency, and the share of old capital stock was significantly correlated with pure technical efficiency and scale efficiency (correlation coefficients of 0.643 and -0.695, respectively); thus, further study is of great practical importance for optimizing the investment mechanism of economic recovery funds. In addition, for the border areas of the Tibetan Plateau, it is particularly important to realize a balanced and coordinated development of the economy and ecological environment following natural disasters. Whether the choice of industrial development path in disaster areas is compatible with the carrying capacity of resources and the environment there is an important direction for future research.
References
[3] Towards a theory of economic recovery from disasters. International Journal of Mass Emergencies & Disasters, 32, 171-181(2012).
[4] et alData Envelopment Analysis: Theory, Methodology, and Applications. Netherlands:Springer.(1994).
[6] Social accounting for disaster preparedness and recovery planning. Technical Report NCEER-93-0002, National Centre for Earthquake Engineering Research.(1993).
[8] . The Economics of Natural Disasters: Implications for Federal Policy, 43-63(1969).
[9] A method based on input-output model to evaluate economic impact of disasters. Journal of Natural Disasters, 19, 113-118(2010).
[10] et al. Planning of Post-Disaster Reconstruction of Wenchuan: Resources and Environmental Carrying Capacity Evaluation(2009).
[11] Productivity growth, technical progress, and efficiency change in industrialized countries: Reply. American Economic Review, 87, 1040-1044(1997).
[14] . Climate Change 2013: The Physical Science Basis(2013).
[17] Post-Ike economic resilience along the Texas coast. Disasters, 39, 493-521(2015).
[18] Damage assessment in tourism caused by an earthquake disaster. Infrastructure Planning Review, 3, 56-74(2013).
[19] et al. China’s Climate Extreme and Disaster Risk Management and Adapt to The National Assessment Report.(2015).
[21] An economic framework for the development of a resilience index for business recovery. International Journal of Disaster Risk Reduction, 73-83(2013).
[24] et alResearch review and prospects of natural disasters regionalization in China. Geographical Research, 36, 1401-1414(2017).
[26] Do natural disasters promote long-run growth?. Economic Inquiry, 40, 664-687(2002).
[27] Post-natural disaster economic recovery: Recent progress. Journal of Beijing Normal University, 52, 196-201(2016).
[29] et alTheoretical and empirical study of the impact of Wenchuan earthquake on economic growth in Aba Prefecture. Journal of Natural Disasters, 23, 90-97(2014).
[32] et alCost-benefit analysis of China’s state-run natural disaster relief system: Methods and application. Journal of Beijing Normal University (Natural Science), 50, 100-104(2014).
[33] et alDisaster recovery measurement framework: An application case of disaster recovery after hurricane Katrina. Journal of Natural Disasters, 22, 58-64(2013).
[34] Structural reform and industrial growth in China. Economic Research Journal, 4-20(2009).
[35] The estimation of China’s provincial capital stock: 1952-2000. Economic Research Journal, 35-44(2004).
[36] The analysis of total factor productivity in China: A review and application of Malmquist index approach. Journal of Quantitative & Technical Economics, 25, 111-122(2008).
[37] Analysis of the impact of Wenchuan earthquake on national and regional economic growth and countermeasures. Journal of Southwest Minzu University (Humanities & Social Sciences Edition), 29, 75-79(2008).
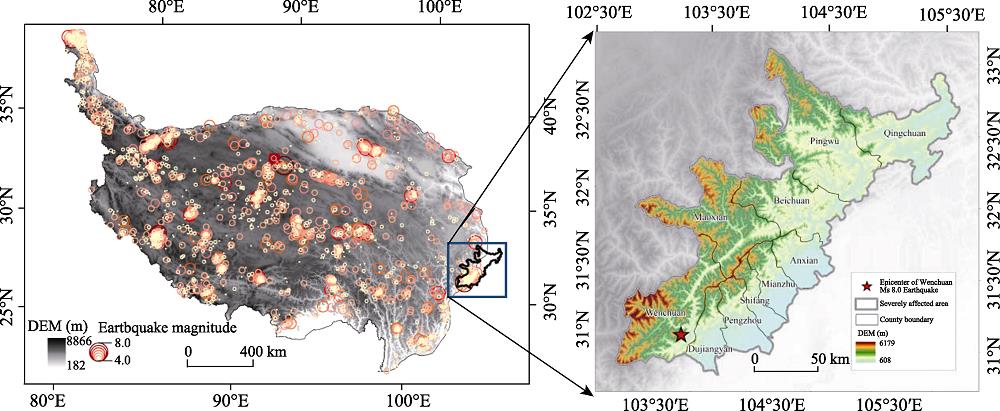
Set citation alerts for the article
Please enter your email address