
- Journal of Geographical Sciences
- Vol. 30, Issue 10, 1649 (2020)
Abstract
1 Introduction
Mapping floodplain inundation extent and depth is critical for the management of river systems, environmental assessment and disaster management (
Two widely used approaches for inundation mapping are hydrodynamic modelling based on water equilibrium equations and flood mapping using remote sensing images. Hydrodynamic modelling uses water equilibrium equations to simulate water movement along river channels and overbank flow (
Use of high-resolution airborne scanning laser altimetry (LiDAR) digital elevation model (DEM) data for flood inundation modelling has also received lots of attention for floodplain/wetland inundation mapping and the river network study since they are of high resolution (1 m or 2 m) and can capture the river network reasonably accurately (
Figure 1.
One limitation of the LiDAR-DEM is that it is only currently available for the small floodplain/wetland regions. For large area application, the inundation modelling framework needs the help of other relative coarse resolution DEM dataset, such as 1-sec SRTM-DEM dataset which is freely available globally (http://srtm.usgs.gov/).
This study develops a LiDAR-DEM based Rapid Inundation Modelling framework, named as LiDAR-RIM. It overcomes the issues associated with hydrodynamic modelling (e.g., slow computation) and remote sensing mapping (e.g., clouds, dense canopies and flood water mixed with water bodies). To take advantage of the high resolution LiDAR-DEM data, the LiDAR-RIM used the LiDAR-DEM together with gauging site information, loss/gain functions, flow data, climate and floodplain soil property to quickly model floodplain inundation extents, depths, volumes (for any given river stage and to simulate the relationship between river stage) and inundation area. The modelling framework was tested for two flood events and at two mid Murrumbidgee river reaches in the southeastern MDB (
2 Modelling framework and data
2.1 LiDAR-RIM
The LiDAR-RIM was developed for quick assessment of flood inundation extent, depth, volume and duration for any high flow event based on LiDAR-DEM data and simple hydraulic principles of mass balance (
1) Multiple LiDAR-DEM files are mosaiced along the mid Murrumbidgee River reaches.
2) River networks are digitized using the mosaiked LiDAR-DEM dataset.
Figure 2.
3) Using the LiDAR-DEM obtains river cross section for a given gauging site (
Figure 3.
4) The initial constrained inundation extent and inundation depth are calculated using mass balance (Equation (1)) and the corresponding inundation volume estimated from the difference between the cumulative flow at the two gauges minus the losses between the two gauges estimated from loss/gain functions in the simplified MDB daily river model (
5) Flood extent and flood depth at each grid cell change from the initial inundation (see step 4) by adding losses due to infiltration (estimated using soil properties) and open water evaporation (estimated from daily climate data after the flood events). For each grid cell, the flood depth gradually reduces, and it reduces to zero once the total depth of infiltration and water evaporation is as same as or larger than the initial flood depth.
6) Inundation extent obtained by LiDAR-RIM is compared to that obtained by Landsat TM/ETM+ images from which the normalised difference water index (NDWI) is mapped (
7) Inundation extents and inundation volumes are simulated at different flood level scenarios.
Step 4 is key, and the mass balance is expressed as:
where
Figure 4.
The LiDAR-FIM was developed in MATLAB by using high performance computation clusters to handle a large DEM matrix with a size up to about 20000×20000 grid cells (about 1600 km2 for 2-m resolution LiDAR-DEM). It took about 3-5 hours for the LiDAR-RIM to map the constrained inundation extent and depth for 20000×20000 grid cells.
2.2 Study area and data
The study area is located in the lower Murrumbidgee floodplain along the Murrumbidgee River (
The LiDAR-RIM was applied for inundation modelling in the study area. Two wetland reaches were selected: one from gauging site 410005 to 410036; another from gauging site 410036 to 410082 (
Some preliminary processes were conducted for the LiDAR-DEM before forcing the LiDAR-RIM. First, individual files were mosaiked (
The 1-sec (approximately 30-m) resolution SRTM-DEM product was provided by Geoscience Australia (
Two flood events were tested in December 2010 and March 2012. The December 2010 flood in the Murrumbidgee River has a return period of ~10 years. The flood started on December 11 and continued until December 22 in this region, with the peak flow occurring on December 18. The March 2012 flood has a return period of ~100 years. The flood started on March 7 and continued until March 14 at this region, with the peak flow occurring on March 9. The Landsat ETM+ image for 2012 flood inundation mapping was taken on March 20, 2012, 6 days after the flood event. The Landsat ETM+ image for the 2010 flood inundation mapping was taken on January 5, 2011, 12 days after the flood event. Both were obtained from Earth Resources Observation and Science Center (
The real-time streamflow data and gauging information at the three gauges of 410045, 410036 and 410082 were obtained from the NSW Government WaterInfo website (
To estimate open water evaporation and local precipitation input for inundation modelling, local climate data including daily precipitation and potential evaporation data was obtained from the Bureau of Meteorology through its free climate data online (
To estimate infiltration, the soil property data for the selected reaches were obtained from a subset of Australian soil property GIS databases which was produced by CSIRO (
3 Results and discussion
3.1 Inundation depth across gauging sites
Gauging site or digitized reach | Depth from zero gauge to LiDAR- DEM (m) | Maximum river stage (m) | Maximum inundation depth (m) | |||
---|---|---|---|---|---|---|
December 2010 flood | March 2012 flood | December 2010 flood | March 2012 flood | |||
Gauging site | 410005 | 1.9 | 7.99 | 8.95 | 6.09 | 7.05 |
410036 | 1.3 | 7.38 | 7.92 | 6.08 | 6.62 | |
410082 | 0.9 | 7.17 | 7.54 | 6.27 | 6.64 | |
Digitized reach | 410005-410036 | - | - | 6.08 | 6.84 | |
410036-410082 | - | - | 6.18 | 6.63 |
Table 1.
Inundation depth for the three gauging sites and for the two flood events. Maximum inundation depth is the difference between maximum river stage and the depth from zero gauge to the LiDAR-DEM water surface.
3.2 Flooding mapping using LiDAR-FIM
Figure 5.
The results shown in
Figure 6.
Figures 7a and 6c describe the relationships between the river stage (H) and the inundation volume (V) for the March 2012 flood and for the reaches 410005-410036 and 410036-410082, respectively. The inundation volume was obtained considering mass balance as described in step 4 in section 2.1. For the reach 410005-410036, inundation did not occur when the river stage was below 7.5 m, and inundation depth increased gradually from the river stage 7.6 m to 8.4 m, but increased sharply from the river stage 8.4 m to 8.9 m. The inundation volume for the stage below 8.4 m was about 1/3 to 1/10 of that for the stage above the 8.4 m. For the reach 410036-410082, the inundation volume was very small when the river stage was below 6.5 m. It increased gradually from the river stage of 6.5 m to 7.3 m, but increased sharply from the river stage of 7.3 m to 7.9 m. The stage-inundation relationship results shown here indicate that the inundation extent is extremely sensitive to certain stage heights, and most of the water spreads on the floodplain when the river stage is above a certain threshold (
Figure 7.
Figures 7b and 7d describe the relationships between H and the inundation area (A) for the two reaches, respectively. For the reach 410005-410036, the inundation area increased gradually with the river stage lifting from 7.6 m to 8.4 m, and increased sharply when the stage was above 8.4 m. For the reach 410036-410082, the inundation area increased gradually when river stage varied from 6.2 m to 7.3 m, and increased quickly when the stage was above 7.3 m. The H-A relationship is accordant to the H-V relationship.
It is noted that the H-V-A relationship derived in
The inundation extent shown in
Figure 8.
3.3 Comparing the flood extent obtaining using LiDAR-RIM and Landsat ETM+
The inundation extents extracted using the Landsat ETM+ images (see step 6 in section 2.1) are used to check the accuracy of those obtained using the LiDAR-RIM. Figures 6a and 6d show the inundation maps (black) obtained from Landsat ETM+ images, overlapped by red polygons that were obtained from the LiDAR-RIM inundation extent (after mass balance, infiltration and open water evaporation) (Figures 6c and 6f). Visual comparisons indicate that most inundated grid cells simulated by the LiDAR-RIM belong to water bodies identified from the Landsat ETM+ NDWI images.
To further check the agreement between the LiDAR-RIM inundation extents and Landsat ETM+ inundation extents, the 2-m LiDAR-RIM inundation extent was re-sampled to 30-m resolution to match the Landsat ETM+ data. The consistency checks show that for the March 2012 flood, the two approaches are consistent at 73.2% of the LiDAR-RIM inundation grid cells (Figures 6a and 6c). For the December 2010 flood, the two approaches are consistent at 70.1% of grid cells. These results indicate that the inundation extents estimated using the LiDAR-RIM considering mass balance, infiltration and open water evaporation agree well with the flood inundation maps generated from the Landsat ETM+ images.
Figure 9.
It is noted that the inundation extents obtained using the Landsat ETM+ images cover many pixels that are not hydrologically connected to the river system. Therefore, flooding in those pixels are not caused by river overbank flow (Figures 6a and 6d). Another weak point for the remote sensing inundation mapping is that it is impossible to directly get inundation depth and volume from the Landsat TM/ETM+ images or other remote sensing images. However, these issues are resolved when using the LiDAR- RIM.
3.4 Comparing the flood extent/area obtained using LiDAR-DEM and SRTM-DEM
The LiDAR-DEM dataset is normally available in the small floodplain/wetland regions, which limit the application of the LiDAR-RIM. For large area application, the modelling framework needs helps from other relative coarse resolution DEM dataset, such as the 30 m resolution SRTM-DEM dataset which is globally available. The SRTM-DEM data has been hydrologically corrected in Australia (
Figures 9a and 9b show constrained inundation extents obtained using the 30-m resolution SRTM-DEM for the March 2012 and December 2010 floods, respectively. The inundation extents shown in Figures 9a and 9b are noticeably smaller than those shown in Figures 6b and 6e and the inundation depth shown in Figures 9a and 9b are noticeably deeper than those shown in Figures 6b and 6e.
Figure 10.
3.5 Strength and limitations
The LiDAR-RIM is relatively simple since it does not consider flow hydraulic characteristics (except mass balance) that are necessary in hydrodynamic modelling. The hydrodynamic modelling approach is considered to be the most suitable method for generating comprehensive flood hazard maps at high spatial and temporal resolutions. However, such a method is a high computational demand. The LiDAR-RIM can be considered as an intermediate approach for rapid assessment of flood inundation due to its small run time. For example, for the selected reaches in the Murrumbidgee, it took less than 5 hours to map the constraint inundation extents and depths shown in Figures 6b, 6c, 6e and 6f. Compared to this, the 2D hydrodynamic modelling at 2-m resolution for the selected reaches would require several days to get simulation results (
It is noted that the proposed inundation mapping approach requires necessary auxiliary data, such as streamflow, precipitation, potential evaporation, soil property, and so on. It is not appliable to the ungauged catchments. However, these are routine data and easily available for gauged catchments, where it is suitable for floodplain inundation mapping. Additionally, it has a high requirement for the cross-section data of a given gauge. Therefore, it is necessary to use high resolution DEM, such as LiDAR-DEM to accurately delineate cross sections and flood water surfaces.
4 Conclusions
This study develops a rapid inundation modelling framework using LiDAR-DEM (LiDAR-RIM), which requires observed streamflow data together with gauge station information and loss/gain functions. It can be effectively used for rapid assessment of flood inundation, including inundation extents, depths, and volume. The LiDAR-RIM has been successfully applied to simulate flood inundation for the March 2012 and the December 2010 flood events in two wetland reaches in mid Murrumbidge River. The inundation extents estimated by the LiDAR-RIM considering mass balance, infiltration and open water evaporation compare well with the water bodies identified by the LANDSAT ETM+ images. This study also tests the applicability of the coarse resolution SRTM-DEM which is globally available. The inundation extents obtained by using the SRTM-DEM is smaller than those obtained using the LiDAR-DEM. The main reason for this is that the river cross sections obtained from the SRTM-DEM are not accurate enough for inundation modelling. It is unrealistic to use the SRTM-DEM for inundation modelling of large flood events. The H-V-A relationships derived using the LiDAR-RIM are also very useful for river system modelling. The LiDAR-RIM is integrated into a simplified river system model for the estimation of exchange of flux between the river and floodplain. It is expected that the LiDAR-RIM will be widely used for floodplain inundation mapping and scenario modelling for flood risk assessment. Therefore, more researches are required to test its accuracy and potentially improve its model structure for better simulations and predictions.
References
[1] Bates PD, De Roo A PJ. A simple raster-based model for flood inundation simulation. Journal of Hydrology, 236, 54-77(2000).
[2] Bates PD, Horritt MS, Smith CN et al. Integrating remote sensing observations of flood hydrology and hydraulic modelling. Hydrological Processes, 11, 1777-1795(1997).
[3] BuskerT, de RooA, GelatiE et al. A global lake and reservoir volume analysis using a surface water dataset and satellite altimetry. Hydrology and Earth System Sciences, 23, 669-690(2019).
[4] ChenB, Krajewski WF, GoskaR et al. Using LiDAR surveys to document floods: A case study of the 2008 Iowa flood. Journal of Hydrology, 553, 338-349(2017).
[5] CookA, MerwadeV. Effect of topographic data, geometric configuration and modelling approach on flood inundation mapping. Journal of Hydrology, 377, 131-142(2009).
[6] Doody TM, Colloff MJ, DaviesM et al. Quantifying water requirements of riparian river red gum (Eucalyptus camaldulensis) in the Murray-Darling Basin, Australia: Implications for the management of environmental flows. Ecohydrology, 8, 1471-1487(2015).
[7] DuttaD. Flood hazard mapping using hydrodynamic modelling approach. In: Wong T. Flood Risk and Flood Management(2012).
[8] AlamJ, UmedaK et al. A two-dimensional hydrodynamic model for flood inundation simulation: A case study in the lower Mekong River Basin. Hydrological Processes, 21, 1223-1237(2007).
[9] A Directory of Important Wetlands in Australia. 3rd ed. Environment Australia, Canberra. Commonwealth of Australia, 137pp.(2001).
[11] Horritt MS, Bates PD. Evaluation of 1D and 2D numerical models for predicting river flood inundation. Journal of Hydrology, 268, 87-99(2002).
[12] Huang CQ, PengY, Lang MG et al. Wetland inundation mapping and change monitoring using Landsat and airborne LiDAR data. Remote Sensing of Environment, 141, 231-242(2014).
[13] HughesJ, DuttaD, Kim S et al. An automated calibration procedure for a river system model. In: Proceedings of the National Conference on Water and Climate: Policy Implementation Challenges, Engineers Australia, 1-3 May 2012, Canberra, CD-ROM version (8 pages).(2012).
[14] Hughes JD, DuttaD, VazeJ et al. An automated multi-step calibration procedure for a river system model. Environmental Modelling & Software, 51, 173-183(2014).
[16] JiangL, SchneiderR, Andersen OB et al. CryoSat-2 altimetry applications over rivers and lakes. Water, 9, 211(2017).
[17] Johnson JM, MunasingheD, EyeladeD et al. An integrated evaluation of the National Water Model (NWM)-Height Above Nearest Drainage (HAND) flood mapping methodology. Natural Hazards and Earth System Sciences, 19, 2405-2420(2019).
[18] LiY, GaoH, Jasinski MF et al. Deriving High-Resolution Reservoir Bathymetry From ICESat-2 Prototype Photon-Counting Lidar and Landsat Imagery. IEEE Transactions on Geoscience and Remote Sensing, 57, 7883-7893(2019).
[19] McFeeters SK. The use of the normalized difference water index (NDWI) in the delineation of open water features. International Journal of Remote Sensing, 17, 1425-1432(1996).
[20] McKenzie NJ, Jacquier DW, Ashton LJ et al. Estimation of soil properties using the atlas of Australian soils, CSIRO Land and Water Technical Report 11/00, February 2000(2000).
[21] Negishi JN, SagawaS, SanadaS et al. Using airborne scanning laser altimetry (LiDAR) to estimate surface connectivity of floodplain water bodies. River Research and Applications, 28, 258-267(2012).
[22] PappenbergerF, BevenK, HorrittM et al. Uncertainty in the calibration of effective roughness parameters in HEC-RAS using inundation and downstream level observations. Journal of Hydrology, 302, 46-69(2005).
[23] Penton DJ, Overton IC. Spatial Modelling of Floodplain Inundation Combining Satellite Imagery and Elevation Models, Modsim 2007: International Congress on Modelling and Simulation: Land,. Water and Environmental Management: Integrated Systems for Sustainability, 1464-1470(2009).
[24] SaksenaS, MerwadeV. Incorporating the effect of DEM resolution and accuracy for improved flood inundation mapping. Journal of Hydrology, 530, 180-194(2015).
[25] Sanders BF. Evaluation of on-line DEMs for flood inundation modelling. Advances in Water Resources, 30, 1831-1843(2007).
[26] SanyalJ, Lu XX. Application of remote sensing in flood management with special reference to monsoon Asia: A review. Natural Hazards, 33, 283-301(2004).
[27] ShaikhM, GreenD, CrossH. A remote sensing approach to determine environmental flows for wetlands of the Lower Darling River, New South Wales, Australia. International Journal of Remote Sensing, 22, 1737-1751(2001).
[28] Smith LC. Satellite remote sensing of river inundation area, stage, and discharge: A review. Hydrological Processes, 11, 1427-1439(1997).
[29] Smith R AE, Bates PD, HayesC. Evaluation of a coastal flood inundation model using hard and soft data. Environmental Modelling & Software, 30, 35-46(2012).
[30] TengJ, VazeJ, DuttaD et al. Rapid inundation modelling in large floodplains using LiDAR DEM. Water Resources Management, 29, 2619-2636(2015).
[31] Thompson JR, Sorenson HR, GavinH et al. Application of the coupled MIKE SHE/MIKE 11 modelling system to a lowland wet grassland in southeast England. Journal of Hydrology, 293, 151-179(2004).
[32] Tseng KH, Shum CK, KimJ et al. Integrating Landsat imageries and digital elevation models to infer water level change in Hoover Dam. IEEE Journal of Selected Topics in Applied Earth Observations and Remote Sensing, 9, 1696-1709(2016).
[33] TsubakiR, KawaharaY. The uncertainty of local flow parameters during inundation flow over complex topographies with elevation errors. Journal of Hydrology, 486, 71-87(2013).
[34] VazeJ, TengJ, SpencerG. Impact of DEM accuracy and resolution on topographic indices. Environmental Modelling & Software, 25, 1086-1098(2010).
[35] WangY, Colby JD, Mulcahy KA. An efficient method for mapping flood extent in a coastal floodplain using Landsat TM and DEM data. International Journal of Remote Sensing, 23, 3681-3696(2002).
[36] Wu XS, Wang ZL, Guo SL et al. Scenario-based projections of future urban inundation within a coupled hydrodynamic model framework: A case study in Dongguan City, China. Journal of Hydrology, 547, 428-442(2017).
[37] ZengL, SchmittM, LiL et al. Analysing changes of the Poyang Lake water area using Sentinel-1 synthetic aperture radar imagery. International Journal of Remote Sensing, 38, 7041-7069(2017).
[38] ZwenznerH, VoigtS. Improved estimation of flood parameters by combining space based SAR data with very high resolution digital elevation data. Hydrology and Earth System Sciences, 13, 567-576(2009).
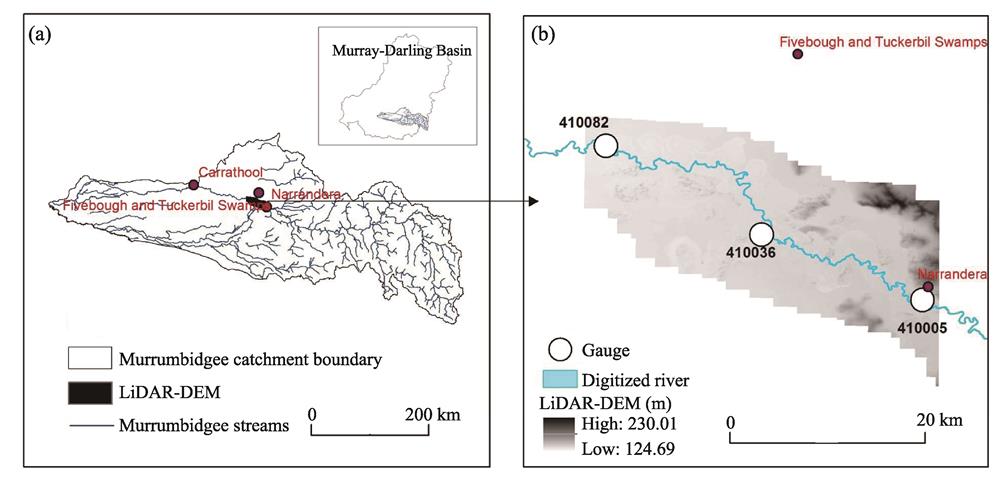
Set citation alerts for the article
Please enter your email address