Ai-chen WANG, Bin-jie GAO, Chun-jiang ZHAO, Yi-fei XU, Miao-lin WANG, Shu-gang YAN, Lin LI, Xin-hua WEI. Detecting Green Plants Based on Fluorescence Spectroscopy[J]. Spectroscopy and Spectral Analysis, 2022, 42(3): 788

Search by keywords or author
- Spectroscopy and Spectral Analysis
- Vol. 42, Issue 3, 788 (2022)
Abstract
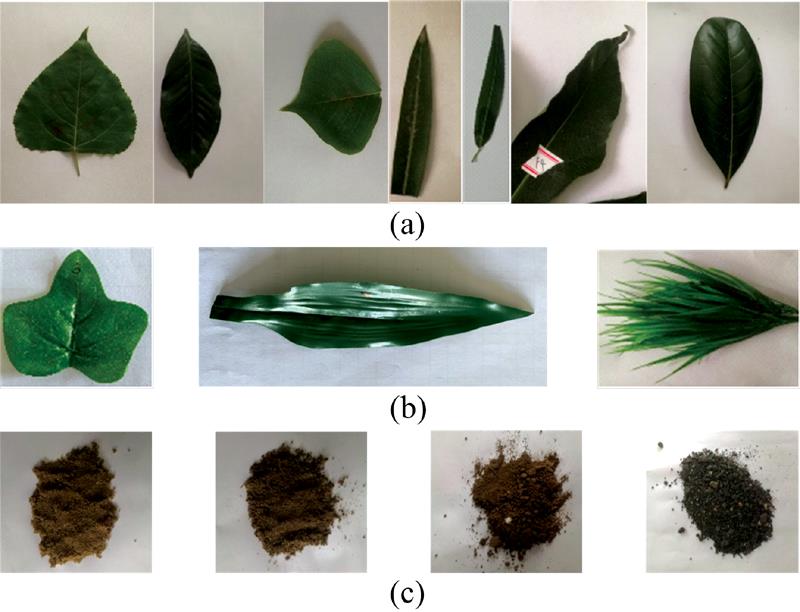
Set citation alerts for the article
Please enter your email address