Yan-de LIU, Shun WANG. Research on Non-Destructive Testing of Navel Orange Shelf Life Imaging Based on Hyperspectral Image and Spectrum Fusion[J]. Spectroscopy and Spectral Analysis, 2022, 42(6): 1792

Search by keywords or author
- Spectroscopy and Spectral Analysis
- Vol. 42, Issue 6, 1792 (2022)
Abstract
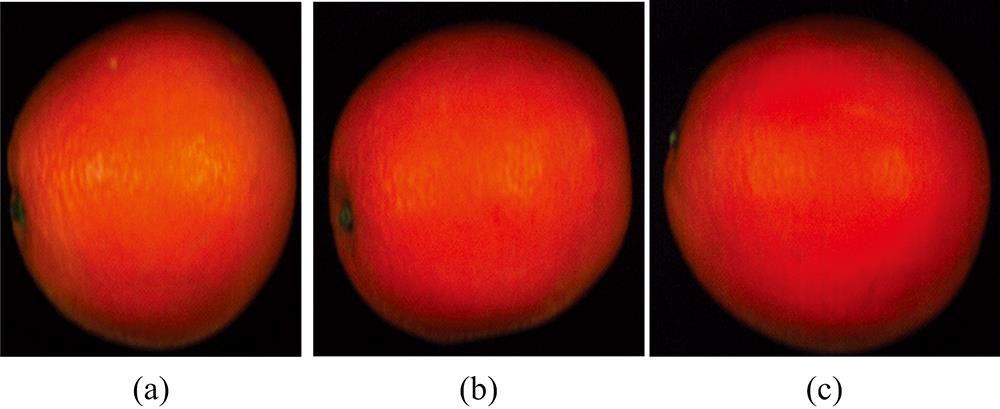
Set citation alerts for the article
Please enter your email address