WANG Cai-ling, WANG Hong-wei, HU Bing-liang, WEN Jia, XU Jun, LI Xiang-juan. A Novel Spatial-Spectral Sparse Representation for Hyperspectral Image Classification Based on Neighborhood Segmentation[J]. Spectroscopy and Spectral Analysis, 2016, 36(9): 2919

Search by keywords or author
- Spectroscopy and Spectral Analysis
- Vol. 36, Issue 9, 2919 (2016)
Abstract
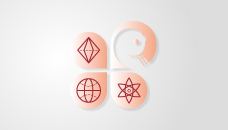
Set citation alerts for the article
Please enter your email address