
Search by keywords or author
- Spectroscopy and Spectral Analysis
- Vol. 42, Issue 9, 2726 (2022)
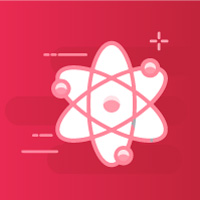
Fig. 1. Location of geographical area of Shunchang County, Nanping City, Fujian Province, remote sensing of experimental areas and measuring points distribution (2D) (b) and (3D) (c)
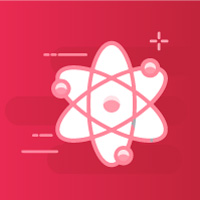
Fig. 2. Random Forest Structure
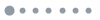
Fig. 3. SPAD variation trend of phyllostachys pubescens leaf samples under different conditions
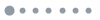
Fig. 4. Leaf samples of phyllostachys pubescens (a) under different conditions and their spectral information (b)
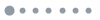
Fig. 5. Pearson correlation analysis of vegetation Index with different pest levels
(1)—(35) represent characteristic Indexes respectively: CIgreen, CIred, NDVI705, DVI, SAVI, OSAVI, MCARI, TCARI, RVI, ARVI, GNDVI, PRI, VARI, NPCI, PRI*CI,R 515/R 570, mSR, VOG1, VOG2, VOG3, 469 nm, 702 nm, 760 nm, 469FDR, 469SDR, 702FDR, 702SDR, 760FDR, 760SDR, CARI, RES, REA, REDVI, RERVI, RENDVI, similarly hereinafter
(1)—(35) represent characteristic Indexes respectively: CIgreen, CIred, NDVI705, DVI, SAVI, OSAVI, MCARI, TCARI, RVI, ARVI, GNDVI, PRI, VARI, NPCI, PRI*CI,
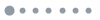
Fig. 6. Pearson correlation analysis of leaf spectral characteristics of leaf samples under different hazard levels
(a): Healthy leaf sample; (b): Mild leaf sample; (c); Moderate leaf sample; (d): Severe leaf sample; (e): Off year leaf sample
(a): Healthy leaf sample; (b): Mild leaf sample; (c); Moderate leaf sample; (d): Severe leaf sample; (e): Off year leaf sample
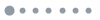
Fig. 7. SPAD detection results of phyllostachys pubescens leaves by four models(R 2 and RMSE are model fitting degree and root mean square error, respectively, similarly hereinafter)
(a): Multiple linear regression; (b): Ridge regression; (c): Random forest regression; (d): XGBoost regression
(a): Multiple linear regression; (b): Ridge regression; (c): Random forest regression; (d): XGBoost regression
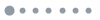
Fig. 8. SPAD detection results of phyllostachys pubescens leaves under different damage conditions by four models
(a): Healthy leaf—Multiple linear regression; (b): Healthy leaf—Ridge regression; (c): Healthy leaf—Random forest regression;(d): Healthy leaf—XGBoost regression; (e): Mild leaf—Multiple linear regression; (f): Mild leaf—Ridge regression;(g): Mild leaf—Random forest regression; (h): Mild leaf—XGBoost regression; (i): Moderate leaf —Multiple linear regression;(j): Moderate leaf —Ridge regression; (k): Moderate leaf —Random forest regression; (l): Moderate leaf —XGBoost regression;(m): Severe leaf —Multiple linear regression; (n): Severe leaf —Ridge regression; (o): Severe leaf —Random forest regression;(p): Severe leaf —XGBoost regression; (q): Off year leaf—Multiple linear regression; (r): Off year leaf —Ridge regression;(s): Off year leaf —Random forest regression; (t): Off year leaf —XGBoost regression;
(a): Healthy leaf—Multiple linear regression; (b): Healthy leaf—Ridge regression; (c): Healthy leaf—Random forest regression;(d): Healthy leaf—XGBoost regression; (e): Mild leaf—Multiple linear regression; (f): Mild leaf—Ridge regression;(g): Mild leaf—Random forest regression; (h): Mild leaf—XGBoost regression; (i): Moderate leaf —Multiple linear regression;(j): Moderate leaf —Ridge regression; (k): Moderate leaf —Random forest regression; (l): Moderate leaf —XGBoost regression;(m): Severe leaf —Multiple linear regression; (n): Severe leaf —Ridge regression; (o): Severe leaf —Random forest regression;(p): Severe leaf —XGBoost regression; (q): Off year leaf—Multiple linear regression; (r): Off year leaf —Ridge regression;(s): Off year leaf —Random forest regression; (t): Off year leaf —XGBoost regression;
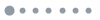
Fig. 9. Characteristic space of leaf spectral Index of all leaf samples and leaf samples under different pest grades
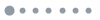
Fig. 10. Changes of model R 2 and RMSE under different regularization parameters
|
Table 1. SPAD-related vegetation Index
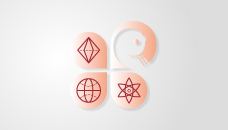
Download Citation
Set citation alerts for the article
Please enter your email address