LU De-jun, CUAN Kai-xuan, ZHANG Wei-feng. Research on Spectral Reflectance Estimation Using Locally Weighted Linear Regression within k-Nearest Neighbors[J]. Spectroscopy and Spectral Analysis, 2018, 38(12): 3708

Search by keywords or author
- Spectroscopy and Spectral Analysis
- Vol. 38, Issue 12, 3708 (2018)
Abstract
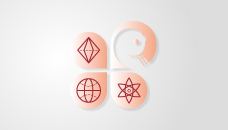
Set citation alerts for the article
Please enter your email address