Yuan LI, Yao SHI, Shao-yuan LI, Ming-xing HE, Chen-mu ZHANG, Qiang LI, Hui-quan LI. Accurate Quantitative Analysis of Valuable Components in Zinc Leaching Residue Based on XRF and RBF Neural Network[J]. Spectroscopy and Spectral Analysis, 2022, 42(2): 490

Search by keywords or author
- Spectroscopy and Spectral Analysis
- Vol. 42, Issue 2, 490 (2022)
Abstract
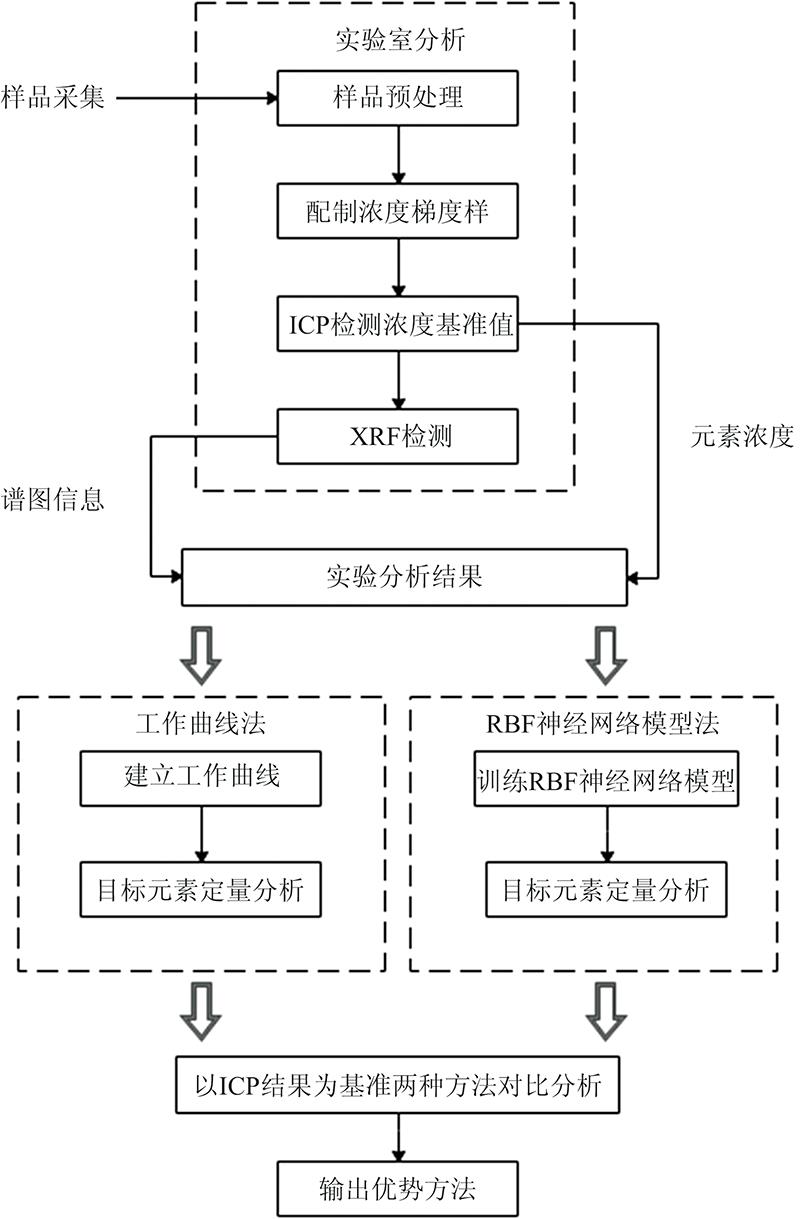
Set citation alerts for the article
Please enter your email address