Jie-hong CHENG, Zheng-guang CHEN. Wavelength Selection of Near-Infrared Spectra Based on Improved SiPLS-Random Frog Algorithm[J]. Spectroscopy and Spectral Analysis, 2020, 40(11): 3451

Search by keywords or author
- Spectroscopy and Spectral Analysis
- Vol. 40, Issue 11, 3451 (2020)
Abstract
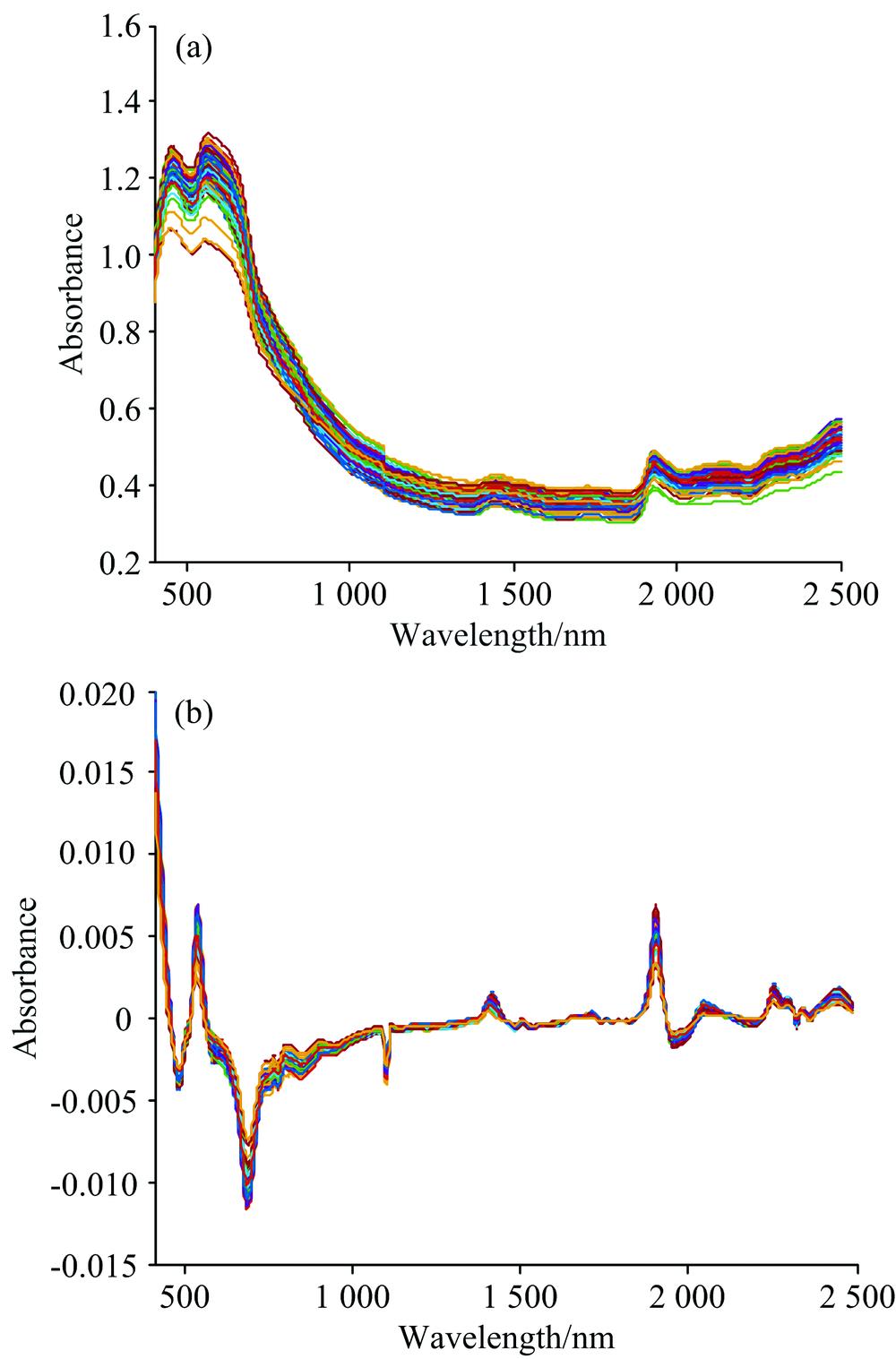
Set citation alerts for the article
Please enter your email address