LUO Long-qiang, YAO Xin-li, HE Sai-ling. Study on the Method of Determining the Survival Rate of Rice Seeds Based on Visible-Near Infrared Multispectral Data[J]. Spectroscopy and Spectral Analysis, 2020, 40(1): 221

Search by keywords or author
- Spectroscopy and Spectral Analysis
- Vol. 40, Issue 1, 221 (2020)
Abstract
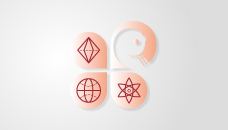
Set citation alerts for the article
Please enter your email address