XU Kai-jian, TIAN Qing-jiu, XU Nian-xu, YUE Ji-bo, TANG Shao-fei. Classifying Forest Dominant Trees Species Based on High Dimensional Time-Series NDVI Data and Differential Transform Methods[J]. Spectroscopy and Spectral Analysis, 2019, 39(12): 3794

Search by keywords or author
- Spectroscopy and Spectral Analysis
- Vol. 39, Issue 12, 3794 (2019)
Abstract
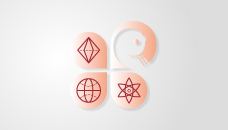
Set citation alerts for the article
Please enter your email address