WANG Wen-jun, LI Zhi-wei, WANG Can, ZHENG De-cong, DU Hui-ling. Prediction of Available Potassium Content in Cinnamon Soil Using Hyperspectral Imaging Technology[J]. Spectroscopy and Spectral Analysis, 2019, 39(5): 1579

Search by keywords or author
- Spectroscopy and Spectral Analysis
- Vol. 39, Issue 5, 1579 (2019)
Abstract
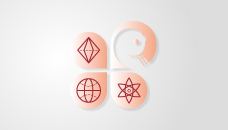
Set citation alerts for the article
Please enter your email address