LIU Zhong-bao, LEI Yu-fei, SONG Wen-ai, ZHANG Jing, WANG Jie, TU Liang-ping. Stellar Spectra Classification by Support Vector Machine with Unlabeled Data[J]. Spectroscopy and Spectral Analysis, 2019, 39(3): 948

Search by keywords or author
- Spectroscopy and Spectral Analysis
- Vol. 39, Issue 3, 948 (2019)
Abstract
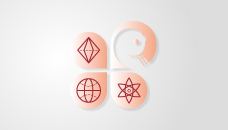
Set citation alerts for the article
Please enter your email address