WANG Hong-bo, ZHAO Zi-qi, LIN Yi, FENG Rui, LI Li-guang, ZHAO Xian-li, WEN Ri-hong, WEI Nan, YAO Xin, ZHANG Yu-shu. Leaf Area Index Estimation of Spring Maize with Canopy Hyperspectral Data Based on Linear Regression Algorithm[J]. Spectroscopy and Spectral Analysis, 2017, 37(5): 1489

Search by keywords or author
- Spectroscopy and Spectral Analysis
- Vol. 37, Issue 5, 1489 (2017)
Abstract
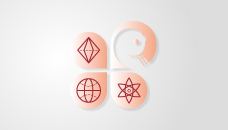
Set citation alerts for the article
Please enter your email address