
- Chinese Optics Letters
- Vol. 20, Issue 2, 020601 (2022)
Abstract
1. Introduction
Nowadays, vortex beams (VBs), carrying orbital angular momentum (OAM), have been known as the hot spots and shown great potential in various fields such as optical micro-manipulation, optical sensors, optical transmission, optical lasers, and amplifiers[
Many schemes have been proposed to detect OAM modes. The most common schemes are interference and diffraction methods[
In this article, we proposed and designed an OAM mode recognition method based on an interferogram processed by a Gaussian smoothing filter (GSF) to identify the OAM modes of VBs. This method is called vortex interferogram recognition (VIR) based on GSF, which is abbreviated as VIR-GSF in this article. Performing simple image feature extraction on the VB light field, this scheme realizes mode recognition. Without a complex convolution and deconvolution process, the computational cost is greatly reduced. Since GSF can strengthen the stability of optical field distribution and reduce the variance of intensity fluctuations, by adding GSF to process the interferogram between the Gaussian beam and the VB, high identification accuracy for the distorted image disturbed by turbulence can be obtained. The calculation results show that this scheme has high accuracy for 1 km transmission in turbulent channels. Specifically, in no-turbulence, weak-turbulence (
Sign up for Chinese Optics Letters TOC. Get the latest issue of Chinese Optics Letters delivered right to you!Sign up now
2. Methods
At the receiver end of the OAM communication system, various OAM modes of the VB need to be demodulated. High-accuracy recognition of OAM modes is a necessary prerequisite for accurately distinguishing different modes. In the proposed scheme, mode recognition is accomplished based on the phase information carried by the VBs[
Figure 1.(a) and (c) are the interference principles between Gaussian beams and VBs with l = +4 and l = −4, respectively. (b) and (d) are obtained interferograms corresponding to l = +4 and l = −4.
Among them, the first item
The second item
In the above expressions,
After the realization of Eqs. (1) and (2), the intensity images
Figure 2.Recognition principle of OAM mode: OAM mode equals the number of intersections between the average value and the falling edge of the waveform, and the mode sign is determined by comparing the phase difference of the waveform at the radii r and r + Δr.
Equation (4) guarantees that the phase difference
Figure 3.Schematic diagram of OAM-FSO communication system with VIR-GSF demodulation technique under the free-space AT channel.
In order to reduce the influence of random scattering on the VBs, we added GSF in the recognition process to optimize the waveform jitter of the matrices
Figure 4.Filtering effect of GSF at different levels m, where m is set as 15 and 25. The comparison of waveforms after GSF operation and the original waveform is given.
Combining our proposed VIR scheme and using GSF can realize OAM mode recognition under AT environment. As a brief summary, we outline the proposed VIR-GSF scheme in Algorithm 1.
1: |
2: Convert F1(N×N), F2(N×N) to grayscale image as F′1(N×N), F′2(N×N) |
3: |
4: Extract elements on circle with radius R from F′1(N×N) as MR |
5: |
6: |
7: r = {R| |
8: Extract elements on circle with radius r from F′2(N×N) as M′r |
9: Extract elements on circle with radius (r + Δr) from F′2(N×N) as M′r+Δr |
10: |
11: |
12: Compare the phase difference |
13: |
14: s = +1 |
15: |
16: s = −1 |
17: |
18: Calculate |l| by intersections between curves |
19: l = s × |l| |
20: |
Table 1. The Proposed VIR-GSF Algorithm
3. Simulations and Results
In the numerical simulation, we used five levels of AT intensity: no turbulence, weak turbulence, medium turbulence, medium-strong turbulence (
Figure 5.Intensity distribution and interferogram of the VBs when the transmission distance is 1 km, where (a), (b), and (c) represent the weak, medium, and strong-turbulence levels, respectively.
When the transmission distance is 1 km, the recognition accuracy of the VIR-GSF scheme is shown in Fig. 6. Under the conditions of no turbulence, since the beam is stable, and its phase distribution is uniform, the mode identification accuracy can reach 100%. When the AT intensity is increased to weak and medium levels, recognition accuracy can be maintained at 100%, and the results are shown in Fig. 6(a). Further increasing the AT intensity to medium-strong and strong levels, the average accuracy can still reach 98.02% and 91.24%, as shown in Fig. 6(b).
Figure 6.Recognition accuracy of various turbulence levels when the transmission distance is 1 km. (a) shows the accuracy under no-turbulence, weak-turbulence, and medium-turbulence conditions. (b) shows that the recognition accuracy and average accuracy under the conditions of medium-strong and strong turbulence.
When the transmission distance is 2 km, the test results are shown in Figs. 7(a)–7(c). The results show that the accuracy can still be maintained at 100% under no-turbulence, weak-turbulence, and medium-turbulence conditions. Upgrading the turbulence level to medium-strong and strong degrees, the accuracy can reach 96.36% and 87.73%, respectively.
Figure 7.(a) Recognition accuracy of the VIR-GSF scheme at the conditions of no turbulence, weak turbulence, and medium turbulence. The recognition accuracy and average accuracy under (b) medium-strong and (c) strong-turbulence conditions. (d) Comparison of performance of CNN, CNN-GS, and VIR-GSF schemes in a turbulent environment for transmission of 2 km.
In addition, the proposed VIR-GSF scheme is compared with two reference methods of CNN and CNN-GS. The traditional CNN algorithm includes the input layer (
4. Conclusion
In this Letter, an OAM modes recognition method based on an interferogram was proposed. This method realizes the OAM modes recognition by computing the number of intersections between the average value and the falling edges of the waveform. Additionally, the GSF algorithm is adopted to reduce the influence of AT on OAM-FSO communication. The VIR-GSF scheme can achieve higher recognition accuracy in turbulent environments without using neural networks. This scheme can greatly save computational resources and reduce system complexity. It is anticipated that our work might be helpful for further improving the reliability of the OAM-FSO communication system.
References
[1] Y. Shen, X. Wang, Z. Xie, C. Min, Q. Liu, M. Gong, X. Yuan. Optical vortices 30 years on: OAM manipulation from topological charge to multiple singularities. Light Sci. Appl., 8, 90(2019).
[2] L. Allen, M. W. Beijersbergen, R. Spreeuw, R. J. C. Spreeuw, J. P. Woerdman. Orbital angular momentum of light and the transformation of Laguerre–Gaussian laser modes. Phys. Rev. A, 45, 8185(1992).
[3] L. Chen, R. K. Singh, A. Dogariu, Z. Chen, J. Pu. Estimating topological charge of propagating vortex from single-shot non-imaged speckle. Chin. Opt. Lett., 19, 022603(2021).
[4] Y. Shen, Z. Shen, G. Zhao, W. Hu. Photopatterned liquid crystal mediated terahertz Bessel vortex beam generator. Chin. Opt. Lett., 18, 080003(2020).
[5] A. E. Willner, H. Huang, Y. Yan, Y. Ren, N. Ahmed, G. Xie, C. Bao, L. Li, Y. Cao, Z. Zhao, J. Wang, M. Lavery, M. Tur, S. Ramachandran, A. Molisch, N. Ashrafi, S. Ashrafi. Optical communications using orbital angular momentum beams. Adv. Opt. Photon., 7, 66(2015).
[6] J. Wang, J. Yang, I. Fazal, N. Ahmed, Y. Yan, H. Huang, Y. Ren, Y. Yue, S. Dolinar, M. Tur, A. E. Willner. Terabit free-space data transmission employing orbital angular momentum multiplexing. Nat. Photon., 6, 488(2012).
[7] L. Zhao, T. Jiang, M. Mao, Y. Zhang, H. Liu, Z. Wei, D. Deng, A. Luo. Improve the capacity of data transmission in orbital angular momentum multiplexing by adjusting link structure. IEEE Photon. J., 12, 5501111(2020).
[8] J. Wang, S. Li, M. Luo, J. Liu, L. Zhu, C. Li, D. Xie, Q. Yang, S. Yu, J. Sun, X. Zhang, W. Shieh, A. WillnerEuropean Conference on Optical Communication (ECOC). N-dimentional multiplexing link with 1.036-Pbit/s transmission capacity and 112.6-bit/s/Hz spectral efficiency using OFDM- 8QAM signals over 368 WDM pol-muxed 26 OAM modes(2014).
[9] Q. Tian, L. Zhu, Y. Wang, Q. Zhang, B. Liu, X. Xin. The propagation properties of a longitudinal orbital angular momentum multiplexing system in atmospheric turbulence. IEEE Photon. J., 10, 7900416(2018).
[10] L. Chen, W. Zhang, Q. Lu, X. Lin. Making and identifying optical superposition of very high orbital angular momenta. Phys. Rev. A, 88, 4019(2013).
[11] D. Zibar, M. Piels, R. Jones, C. G. Schaeffer. Machine learning techniques in optical communication. J. Lightwave Technol., 34, 1442(2016).
[12] T. Doster, A. T. Watnik. Machine learning approach to OAM beam demultiplexing via convolutional neural networks. Appl. Opt., 56, 3386(2017).
[13] M. I. Dedo, Z. Wang, K. Guo, Z. Guo. OAM mode recognition based on joint scheme of combining the Gerchberg–Saxton (GS) algorithm and convolutional neural network (CNN). Opt. Commun., 456, 124696(2020).
[14] P. Li, B. Wang, X. Song, X. Zhang. Non-destructive identification of twisted light. Opt. Lett., 41, 1574(2016).
[15] S. Zhao, J. Leach, L. Gong, J. Ding, B. Zheng. Aberration corrections for free-space optical communications in atmosphere turbulence using orbital angular momentum states. Opt. Express, 20, 452(2012).
[16] J. Vickers, M. J. Burch, R. Vyas, S. Singh. Phase and interference properties of optical vortex beams. J. Opt. Soc. Am. A, 25, 823(2008).
[17] T. Doster, A. T. Watnik. Laguerre–Gauss and Bessel–Gauss beams propagation through turbulence: analysis of channel efficiency. Appl. Opt., 55, 10239(2016).
[18] L. C. Andrews, R. L. Phillips. Laser Beam Propagation through Random Media(2005).
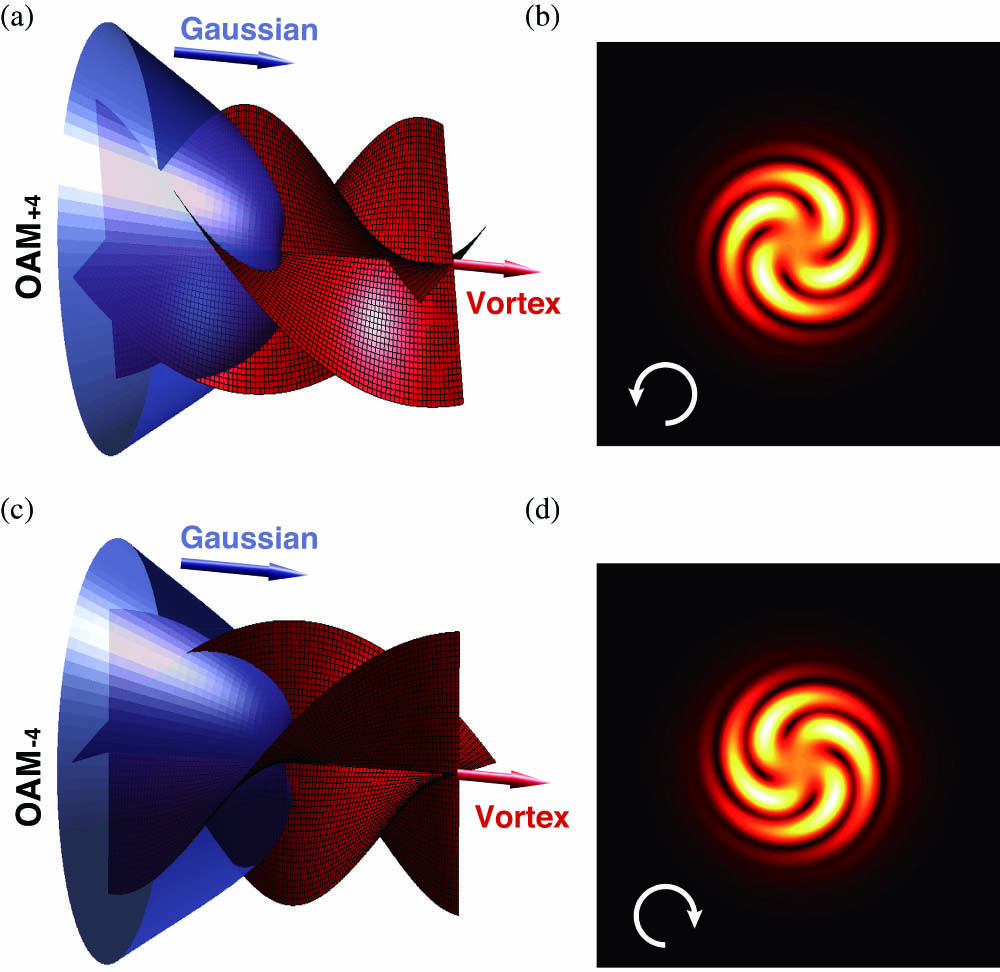
Set citation alerts for the article
Please enter your email address