LI Zhi-hao, SHEN Jun, BIAN Rui-hua, ZHENG Jian. Accuracy Comparison of the Machine Learning Algorithm Used to Raman Real Sample Collection in the Front Line of Public Security[J]. Spectroscopy and Spectral Analysis, 2019, 39(7): 2171

Search by keywords or author
- Spectroscopy and Spectral Analysis
- Vol. 39, Issue 7, 2171 (2019)
Abstract
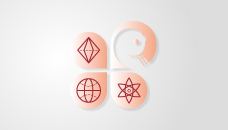
Set citation alerts for the article
Please enter your email address