SU Wei, ZHAO Xiao-feng, SUN Zhong-ping, ZHANG Ming-zheng, ZOU Zai-chao, WANG Wei, SHI Yuan-li. Estimating the Corn Canopy Chlorophyll Content Using the Sentinel-2A Image[J]. Spectroscopy and Spectral Analysis, 2019, 39(5): 1535

Search by keywords or author
- Spectroscopy and Spectral Analysis
- Vol. 39, Issue 5, 1535 (2019)
Abstract
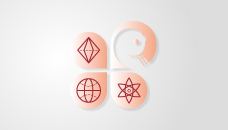
Set citation alerts for the article
Please enter your email address