Chonghui Zheng, Tianshu Wang, Zheqi Liu, Qiaochu Yang, Xianzhu Liu. Deep transfer learning method to identify orbital angular momentum beams[J]. Opto-Electronic Engineering, 2022, 49(6): 210409

Search by keywords or author
- Opto-Electronic Engineering
- Vol. 49, Issue 6, 210409 (2022)
Abstract
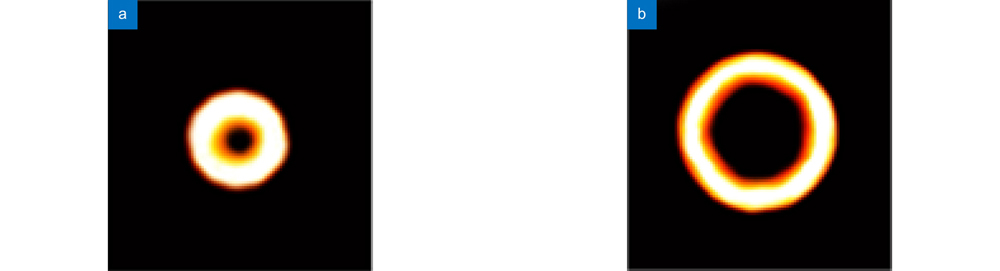
Set citation alerts for the article
Please enter your email address