YU Lei, HONG Yong-sheng, ZHOU Yong, ZHU Qiang. Inversion of Soil Organic Matter Content Using Hyperspectral Data Based on Continuous Wavelet Transformation[J]. Spectroscopy and Spectral Analysis, 2016, 36(5): 1428

Search by keywords or author
- Spectroscopy and Spectral Analysis
- Vol. 36, Issue 5, 1428 (2016)
Abstract
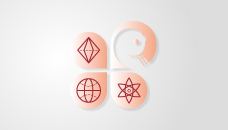
Set citation alerts for the article
Please enter your email address