Yalin Song, Yanwei Pang. Backbone Network for Object Detection Task[J]. Laser & Optoelectronics Progress, 2020, 57(4): 041021

Search by keywords or author
- Laser & Optoelectronics Progress
- Vol. 57, Issue 4, 041021 (2020)
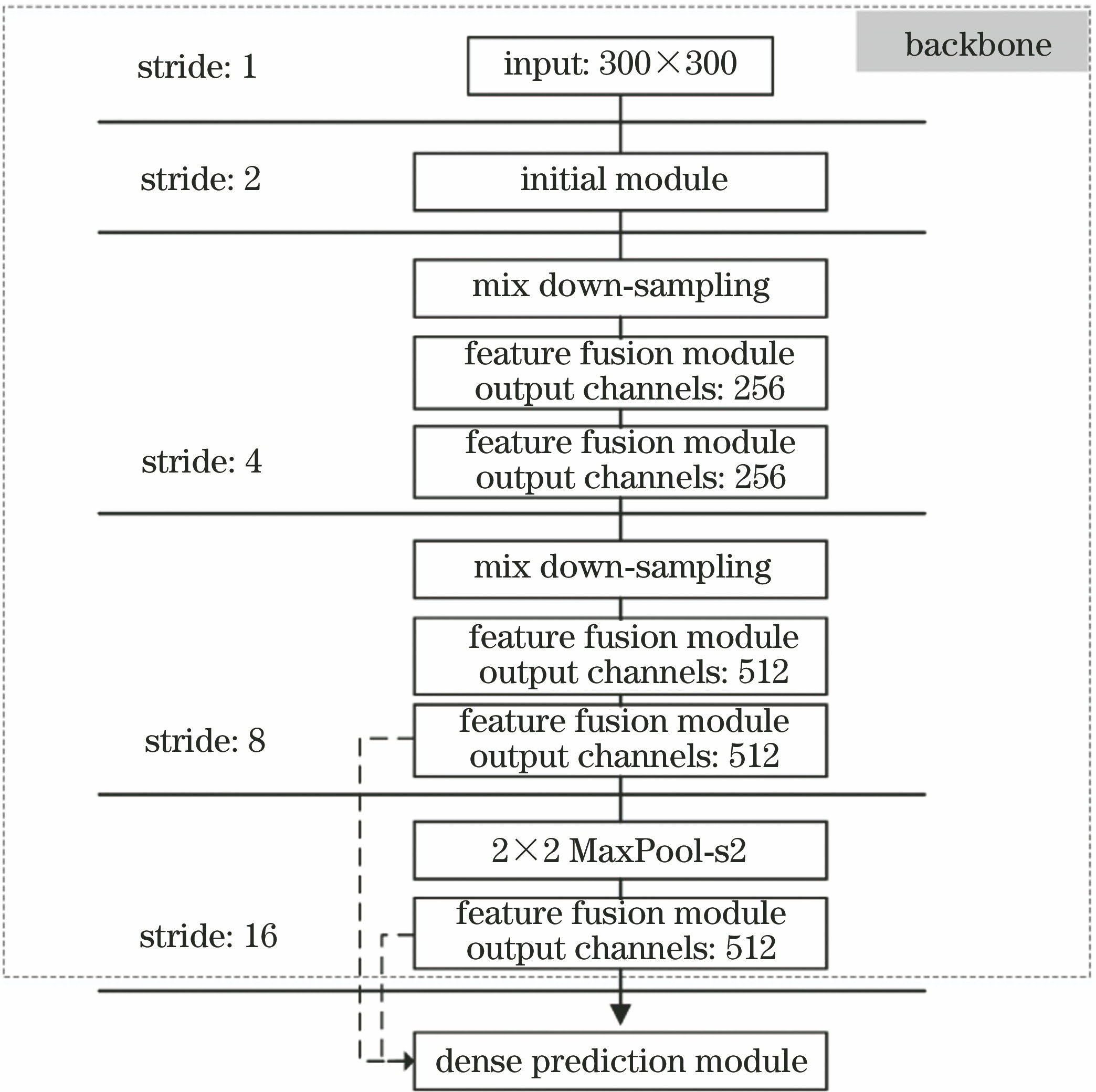
Fig. 1. Network architecture
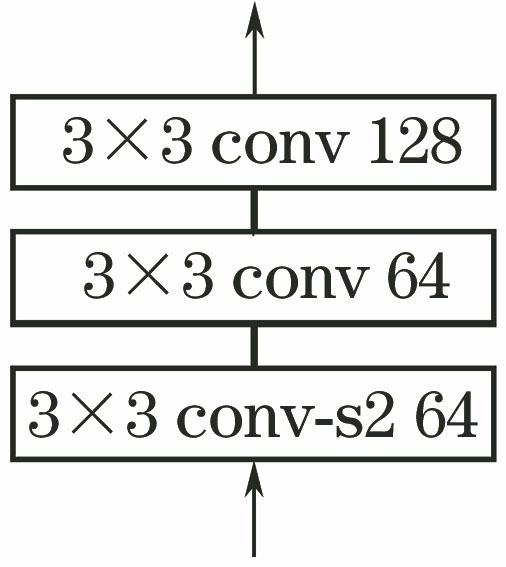
Fig. 2. Initial module
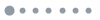
Fig. 3. Feature fusion module
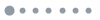
Fig. 4. Mix down-sampling module
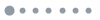
Fig. 5. Prediction modules. (a) Plain prediction module; (b) dense prediction module
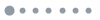
Fig. 6. Qualitative detection results
|
Table 1. Comparison of different initial modules
|
Table 2. Comparison of different feature fusion methods
|
Table 3. Comparison of different down-sampling modules
|
Table 4. Comparison of different prediction modules
|
Table 5. Detection resultsof different backbone networks in SSD, DSOD, and RFBNet models
|
Table 6. Detection results of different detectors on the PASCAL VOC dataset
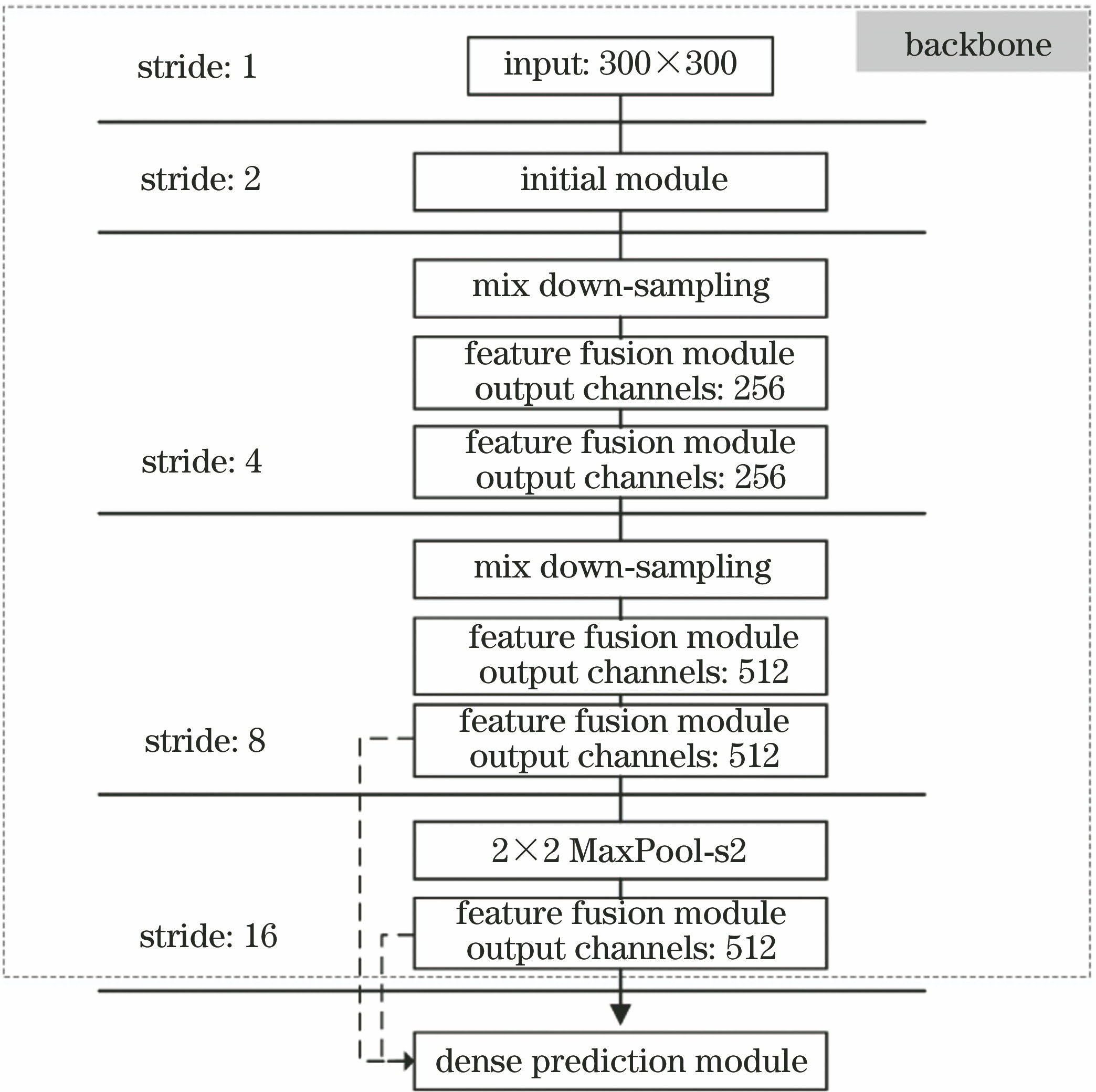
Set citation alerts for the article
Please enter your email address