Pan Huang, Peng He, Xing Yang, Jiayang Luo, Hualiang Xiao, Sukun Tian, Peng Feng. Breast tumor grading network based on adaptive fusion and microscopic imaging[J]. Opto-Electronic Engineering, 2023, 50(1): 220158

Search by keywords or author
- Opto-Electronic Engineering
- Vol. 50, Issue 1, 220158 (2023)
Abstract
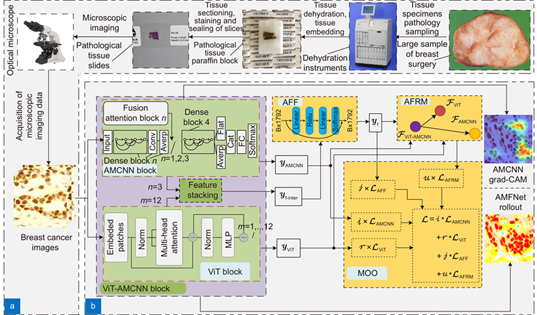
Set citation alerts for the article
Please enter your email address