Bao-hua YANG, Zhi-wei GAO, Lin QI, Yue ZHU, Yuan GAO. Prediction Model of Soluble Solid Content in Peaches Based on Hyperspectral Images[J]. Spectroscopy and Spectral Analysis, 2021, 41(11): 3559

Search by keywords or author
- Spectroscopy and Spectral Analysis
- Vol. 41, Issue 11, 3559 (2021)
Abstract
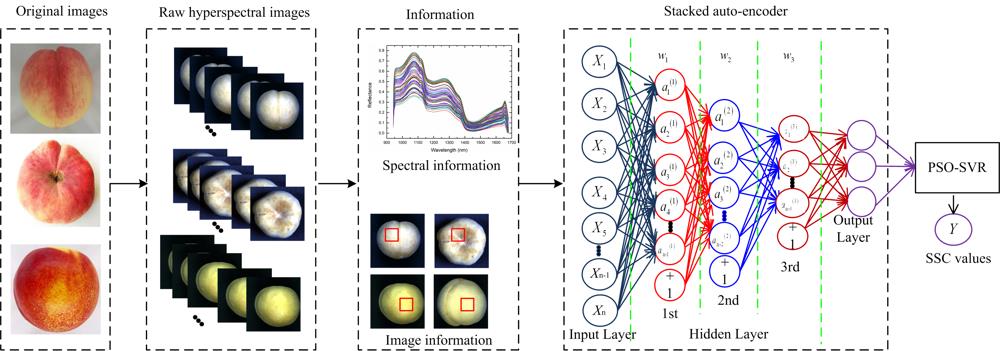
Set citation alerts for the article
Please enter your email address