Dong-xu SHEN, Ming-jian HONG, Jia-lin DONG. Raman Spectrum Wavelength Selection Method Based on Neural Network[J]. Spectroscopy and Spectral Analysis, 2020, 40(11): 3457

Search by keywords or author
- Spectroscopy and Spectral Analysis
- Vol. 40, Issue 11, 3457 (2020)
Abstract
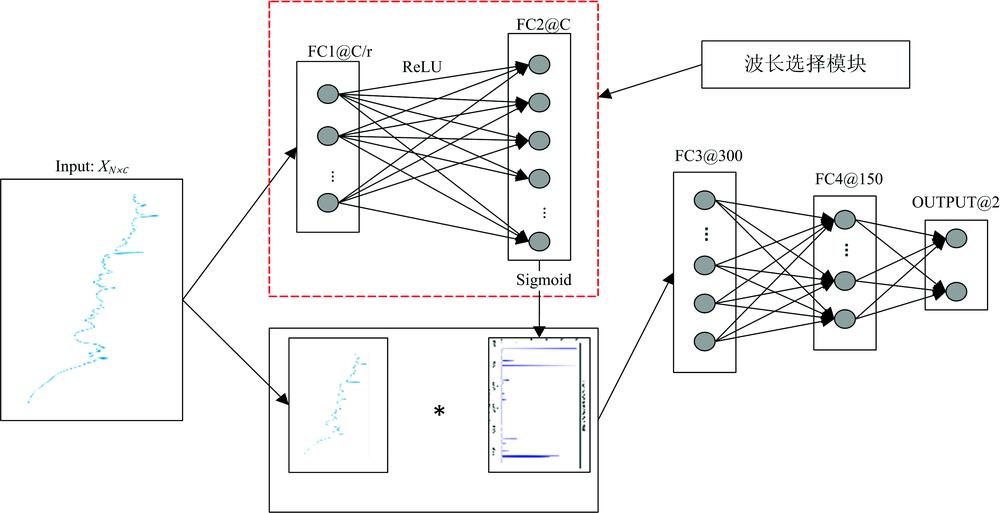
Set citation alerts for the article
Please enter your email address