
- Chinese Optics Letters
- Vol. 20, Issue 3, 033401 (2022)
Abstract
1. Introduction
Ghost imaging (GI), referred to as correlated imaging, is a non-localized imaging method that has attracted extensive attention over the past few decades[
Up to now, X-ray GI (XGI) has attracted extensive attention and has been investigated theoretically and experimentally in recent years with pseudo-thermal[
Reconstruction of megapixel and high-fidelity images with few measurements is a major challenge for XGI. As is well known, the number of measurements needed for image reconstruction with acceptable SNR is proportional to the number of pixels in the image. For the reconstruction of a megapixel image based on the classic ensemble average algorithm, typically many more measurements than the Nyquist–Shannon criterion are needed to achieve sufficient fidelity, which implies tedious data acquisition and long acquisition time. Reducing the number of measurements significantly is apparently the critical path for the realization of practical XGI. The compressed sensing algorithm (CSA) is proposed for the reconstruction of XGI, which can reduce the acquisition time dramatically[
Sign up for Chinese Optics Letters TOC. Get the latest issue of Chinese Optics Letters delivered right to you!Sign up now
Inspired by the concept of synthetic aperture radar (SAR), a method called synthetic aperture XGI (SAXGI) is proposed in this study to reconstruct megapixel XGI images with high fidelity using few measurements.
2. Principle
2.1. Theory for synthetic aperture X-ray ghost imaging
The experimental setup for SAXGI is shown in Fig. 1. The pseudo-thermal X-ray source is artificially created by sandpaper sheets acting as masks illuminated by a collimated monochromatic X-ray beam. The stochastic intensity fluctuation at different sampling times is achieved by scanning the sandpaper transversely by a motor stage to illuminate different parts of the sandpaper. The object is placed a distance
Figure 1.Scheme of experimental setup for synthetic aperture X-ray ghost imaging (SAXGI), showing the reference arm and object arm.
With the object out of the optical path (reference arm), the X-ray beam propagates in free space and then impinges on
To attain a good fidelity, TXGI based on ensemble averaging requires many more measurements than pixels of the reconstructed image. The large number of measurements makes TXGI unsuitable for practical applications concerning data acquisition efficiency. Thus, the concept of SAR[
The CSA is introduced to further reduce the number of measurements while retaining sufficient image quality. Combined with total variation (TV) regularization[
As is well known, for a larger block, more measurements are required to maintain an acceptable block sampling rate and achieve a successful reconstruction of the block image. On the other hand, according to the principle of the CSA, an image block with small size generally leads to the loss of sparse characteristics. This means that the block size selected for SAXGI should be optimized. Simulations were carried out to investigate the effect of block size on the SAXGI image quality, and the results are presented in Section 2.2.
Finally, the large-size image is synthesized from a sequence of image blocks. The quality of the reconstructed images is evaluated by the structural similarity (SSIM) index[
2.2. Effect of block size on the restored image
When the block size is too small, it is difficult for the CSA to accurately describe the sparse characteristics of each block in natural images, which makes it invalid to exactly restore the images. Accordingly, the peak SNR (PSNR) of the restored images tends to decrease with reduced block size. A simulation was carried out to verify this property. In the simulation with 1% Gaussian white noise added, to achieve the efficient reconstruction of large images, the cameraman image with
Simulation results for the effect of block size on the restored image at different sampling rates are shown in Fig. 2. Figure 2(a) presents the target image for comparison. Figure 2(b) corresponds to the square block size of 20. Due to the limited block size, deteriorated image quality can be observed directly, especially at the areas denoted with yellow and green boxes. With the block size increased to 40, the corresponding image quality was visibly improved, as shown in Fig. 2(c). When the block size was 60, the improvement of image quality in Fig. 2(d) relative to that in Fig. 2(c) was not significant. To analyze the effect of block size on the image quality quantitatively, image reconstruction with a series of block sizes of SAXGI was carried out. As shown in Fig. 2(e), the PSNR of the restored images rises sharply with the block size until the block size reaches 20. At a block size of 30, the rising rate of the PSNR begins to decline. After a block size of 40, the increase of the PSNR tends to gentle. As shown in Fig. 2(e), for sampling rates of 20%, 30%, and 40%, the higher the sampling rate, the larger the PSNR. The trend of the PSNR with block size is similar for all measured sampling rates. By appropriately compromising the number of measurements and PSNR in actual SAXGI for radiology, a block size of 40 and sampling rate of 30% are generally preferred. To demonstrate the ability of SAXGI for megapixel imaging, a simulation using an image of SSRF scenery with
Figure 2.Simulation results for the effect of block size on the restored images by SAXGI. (a) Target image. (b), (c), (d) Restored images at square block sizes of 20, 40, and 60, respectively, using a sampling rate of 30%. (e) Peak signal-to-noise ratio (PSNR) of the restored images versus block size at different sampling rates.
3. Results and Discussion
3.1. Simulations
To demonstrate the ability of SAXGI for megapixel imaging, a simulation was carried out prior to experiments. In simulation, the experimental setup was conceived, as shown in Fig. 1. The simulated speckle field was generated by the method mentioned above. The maximum value of its autocorrelation was far below the theoretical maximum of two, because the refractive index of the simulated speckle modulated the phase of X-rays within a range of much less than
The target image used in the numerical simulation was a photograph of the exterior scenery of SSRF with an image size of
Figure 3.Numerical simulation on the megapixel SAXGI. (a) Target image. (b) Signals in the object arm with binning of 64 × 64. (c) Image reconstructed by TXGI with 1000 measurements. (d) Image reconstructed by SAXGI with 1000 measurements. (e) Line profiles of the images marked at the red lines of (a) and (d). (f) Line profiles of the images marked at the yellow lines of (a) and (d).
Figure 3(d) shows the result of SAXGI, in which details of the target image, including the fuzzy branches of the bush and the ripples on the water surface, were clearly reconstructed. The block sampling rate of 24.4% was applied to meet the demand of the CSA, which contributed to a high-fidelity and high-resolution image restored by SAXGI. To compare the image reconstructed by SAXGI with the target image quantitatively, line profiles of the branches marked with red lines in Figs. 3(a) and 3(d) are presented in Fig. 3(e), which confirms the accurate reconstruction of the target image, especially for branches with larger size. Figure 3(f) shows the line profiles of ripples on the water surface of the pool marked with yellow lines in Figs. 3(a) and 3(d), respectively. Due to the low contrast induced by the ripples and reflection of the pool floor, the consistency of line profiles in Fig. 3(f) is poorer than that in Fig. 3(e). This demonstrates that objects with high contrast tend to be recovered with higher fidelity with the CSA based on TV regularization.
To further compare Figs. 3(d) and 3(a) quantitatively, the SSIM index is employed, and, in this case, its value is 0.982, a value quite close to one, which indicates that the image reconstructed by SAXGI maintains high fidelity with the target image. Meanwhile, the SSIM value for the TXGI image is 0.009, which agrees well with the fact that no effective spatial information of the target image can be retrieved through TXGI, as shown in Fig. 3(c). As verified by the numerical simulation, SAXGI is able to deal with megapixel image reconstruction with high fidelity under very limited measurements, while TXGI is ineffective under these conditions.
3.2. Experiments
A typical sample, especially a tangled strand of tungsten fiber, was chosen to test the feasibility of SAXGI for a binary object. The SAXGI experiments were carried out at beamlines of BL09B and BL13W at SSRF, a 3.5 GeV third-generation synchrotron radiation facility[
Figure 4.Speckle pattern and corresponding autocorrelation function in the experiment. (a) Speckle pattern taken by the detector DR with the pixel size of 3.25 µm. (b) Autocorrelation function over the whole speckle pattern, which has the maximum value of 1.26 and full width at half-maximum (FWHM) of 72 µm.
The sample was mounted 45 cm downstream of the sandpaper, followed by the Hamamatsu detector (model: ORCA-flash 4.0 C11440), which was less than 1 cm away from the object. The basic pixel size of the Hamamatsu detector is 6.5 µm. The X-ray beam impinged on a scintillator to obtain a projected image of visible light and was then magnified with a microscope objective. The effective pixel size depends on the optical magnification. The decline of the correlation strength caused by the defocused distance
Tungsten fiber is a true binary object due to the strong absorption of tungsten at the photon energy of 15 keV. The tangled strand of tungsten fiber was taken as the test sample with high contrast and complex structure for SAXGI. The target image shown in Fig. 5(a) was obtained by projection imaging with a field of view of
Figure 5.SAXGI experiments for tungsten fiber. (a) Target image taken by DR, where the edge of the cross is smooth in the inset. (b) Signals in object arm with a binning of 40 × 40. (c) Image reconstructed by TXGI with 660 measurements. (d) Image reconstructed by SAXGI with the same measurements, where the edge of the cross is uneven in the inset.
Then, the reconstructed image by SAXGI is shown in Fig. 5(d). Obviously, the complex structure of the tungsten fiber was successfully retrieved, and the quality of the image restored by SAXGI was significantly improved compared with that by TXGI. Both the background noise and the complexity generated by the overlapping of the tungsten fiber may aggravate the difficulty of high-quality reconstruction. Therefore, the block sampling rate of 41.25% was employed to ensure accurate reconstruction for SAXGI. The similarity of the morphology of the crosses in the insets of Figs. 5(a) and 5(d) proved the reconstruction accuracy, although the edge of the tungsten fiber got slightly blurred in the reconstructed image. Results shown in Fig. 6 indicate that high consistency of the line profiles was achieved.
Figure 6.Comparison between the line profiles of different positions in the target image and the reconstructed image. (a) Line profiles of the sparse tungsten fiber marked with red lines in Figs.
To evaluate the precision of the image reconstruction by SAXGI, line profiles of the sparse fibers denoted with red lines in Figs. 5(a) and 5(d) are presented in Fig. 6(a). High consistency between the SAXGI image and the target image can be observed, especially at positions with high contrast. As shown in Fig. 6(a), the flat top of the profile depicts the grayscale of the flat background without absorption. Moreover, line profiles of the stacked fibers denoted with green lines in Figs. 5(a) and 5(d) also match the positions and heights of the peaks of the curves quite well, as shown in Fig. 6(b). However, the consistency of the profiles at the lower and narrower peaks was obviously reduced, which confirms that simpler structure and higher contrast of objects would lead to higher fidelity of restoration. The SSIM value of 0.976 confirms the high fidelity of the tungsten fiber image reconstructed by SAXGI compared to the target image. Meanwhile, the SSIM value of 0.044 verifies the inability of TXGI to reconstruct the tungsten fiber from so few measurements.
Theoretically, the resolution limit of real-space XGI through the CSA can surpass the Rayleigh diffraction limit[
Image reconstruction efficiency is also important for applicability of the proposed method. The practical computing time for the reconstruction of ghost images depends on many factors including size of the block image, number of blocks, number of measurements, and image complexity. In the simulation, the time for the reconstruction of one block with a size of
As a result, experiments with a high absorption sample demonstrate the excellence of SAXGI for retrieving the image with high contrast and structure complexity from very few measurements.
4. Conclusion
As a powerful non-localized radiology, XGI has attracted extensive attention in recent years. However, reconstruction of megapixel and high-fidelity images with fewer measurements is a major challenge for XGI. Inspired by the concept of SAR, SAXGI is proposed in this study for the realization of megapixel and high-fidelity XGI using very few measurements. Both simulations and experiments were carried out to demonstrate the superiority of the proposed method. In the simulation, the SSIM up to 0.982 indicated that SAXGI almost perfectly retrieved the fine detail of the scenery image with
The ability of SAXGI for large field of view, high-fidelity XGI using few measurements implies that the proposed method may find applications in in-situ imaging of a variety of samples, especially when data acquisition efficiency is crucial. Moreover, when combined with computational XGI and a direct recording X-ray detector in the signal arm, the data acquisition efficiency of SAXGI can be further improved, and a dynamic XGI is also predictable.
References
[1] A.-X. Zhang, Y.-H. He, L.-A. Wu, L.-M. Chen, B.-B. Wang. Tabletop X-ray ghost imaging with ultra-low radiation. Optica, 5, 374(2018).
[2] H. Yu, R. Lu, S. Han, H. Xie, G. Du, T. Xiao, D. Zhu. Fourier-transform ghost imaging with hard X rays. Phys. Rev. Lett., 117, 113901(2016).
[3] Z. Bo, W. Gong, S. Han. Motion de-blurring by second-order intensity-correlated imaging. Chin. Opt. Lett., 14, 070301(2016).
[4] A. Valencia, G. Scarcelli, M. D’Angelo, Y. Shih. Two-photon imaging with thermal light. Phys. Rev. Lett., 94, 063601(2005).
[5] J.-E. Oh, Y.-W. Cho, G. Scarcelli, Y.-H. Kim. Sub-Rayleigh imaging via speckle illumination. Opt. Lett., 38, 682(2013).
[6] W.-K. Yu, X.-F. Liu, X.-R. Yao, C. Wang, G.-J. Zhai, Q. Zhao. Single-photon compressive imaging with some performance benefits over raster scanning. Phys. Lett. A, 378, 3406(2014).
[7] J. Cheng. Ghost imaging through turbulent atmosphere. Opt. Express, 17, 7916(2009).
[8] C. Zhao, W. Gong, M. Chen, E. Li, H. Wang, X. Wendong, A. Han. Ghost imaging lidar via sparsity constraints. Appl. Phys. Lett., 101, 141123(2012).
[9] Y. Zhu, J. Shi, H. Li, G. Zeng. Three-dimensional ghost imaging based on periodic diffraction correlation imaging. Chin. Opt. Lett., 12, 071101(2014).
[10] A. N. Boto, P. Kok, D. S. Abrams, S. L. Braunstein, C. P. Williams, J. P. Dowling. Quantum interferometric optical lithography: exploiting entanglement to beat the diffraction limit. Phys. Rev. Lett., 85, 2733(2000).
[11] M. D’Angelo, M. V. Chekhova, Y. Shih. Two-photon diffraction and quantum lithography. Phys. Rev. Lett., 87, 013602(2001).
[12] X.-H. Chen, I. N. Agafonov, K.-H. Luo, Q. Liu, R. Xian, M. V. Chekhova, L.-A. Wu. High-visibility, high-order lensless ghost imaging with thermal light. Opt. Lett., 35, 1166(2010).
[13] X.-H. Chen, Q. Liu, K.-H. Luo, L.-A. Wu. Lensless ghost imaging with true thermal light. Opt. Lett., 34, 695(2009).
[14] M. Zhang, Q. Wei, X. Shen, Y. Liu, H. Liu, J. Cheng, S. Han. Lensless Fourier-transform ghost imaging with classical incoherent light. Phys. Rev. A, 75, 021803(2007).
[15] Y.-H. He, A.-X. Zhang, M.-F. Li, Y.-Y. Huang, B.-G. Quan, D.-Z. Li, L.-A. Wu, L.-M. Chen. High-resolution sub-sampling incoherent X-ray imaging with a single-pixel detector. APL Photonics, 5, 056102(2020).
[16] A. M. Kingston, D. Pelliccia, A. Rack, M. P. Olbinado, Y. Cheng, G. R. Myers, D. M. Paganin. Ghost tomography. Optica, 5, 1516(2018).
[17] D. Pelliccia, M. P. Olbinado, A. Rack, A. M. Kingston, G. R. Myers, D. M. Paganin. Towards a practical implementation of X-ray ghost imaging with synchrotron light. IUCrJ, 5, 428(2018).
[18] D. Pelliccia, A. Rack, M. Scheel, V. Cantelli, D. M. Paganin. Experimental X-ray ghost imaging. Phys. Rev. Lett., 117, 113902(2016).
[19] A. Schori, D. Borodin, K. Tamasaku, S. Shwartz. Ghost imaging with paired X-ray photons. Phys. Rev. A, 97, 063804(2018).
[20] A. Schori, S. Shwartz. X-ray ghost imaging with a laboratory source. Opt. Express, 25, 14822(2017).
[21] S. Li, F. Cropp, K. Kabra, T. J. Lane, G. Wetzstein, P. Musumeci, D. Ratner. Electron ghost imaging. Phys. Rev. Lett., 121, 114801(2018).
[22] M. Cao, X. Yang, J. Wang, S. Qiu, D. Wei, H. Gao, F. Li. Resolution enhancement of ghost imaging in atom vapor. Opt. Lett., 41, 5349(2016).
[23] A. M. Kingston, G. R. Myers, D. Pelliccia, F. Salvemini, J. J. Bevitt, U. Garbe, D. M. Paganin. Neutron ghost imaging. Phys. Rev. A, 101, 053844(2020).
[24] T. Pittman, Y. Shih, D. Strekalov, A. Sergienko. Optical imaging by means of two-photon quantum entanglement. Phys. Rev. A, 52, R3429(1995).
[25] D. Zhang, Y.-H. Zhai, L.-A. Wu, X.-H. Chen. Correlated two-photon imaging with true thermal light. Opt. Lett., 30, 2354(2005).
[26] H.-C. Liu, S. Zhang. Computational ghost imaging of hot objects in long-wave infrared range. Appl. Phys. Lett., 111, 031110(2017).
[27] D. Ceddia, D. M. Paganin. Random-matrix bases, ghost imaging, and X-ray phase contrast computational ghost imaging. Phys. Rev. A, 97, 062119(2018).
[28] D. Donoho. Compressed sensing. IEEE Trans. Inf. Theory, 52, 1289(2006).
[29] W. Gong, S. Han. High-resolution far-field ghost imaging via sparsity constraint. Sci. Rep., 5, 9280(2015).
[30] O. Katz, Y. Bromberg, Y. Silberberg. Compressive ghost imaging. Appl. Phys. Lett., 95, 131110(2009).
[31] G. Wu, T. Li, J. Li, B. Luo, H. Guo. Ghost imaging under low-rank constraint. Opt. Lett., 44, 4311(2019).
[32] R. Zhu, G.-S. Li, Y. Guo. Block-compressed-sensing-based reconstruction algorithm for ghost imaging. OSA Continuum, 2, 2834(2019).
[33] F. Ferri, D. Magatti, L. A. Lugiato, A. Gatti. Differential ghost imaging. Phys. Rev. Lett., 104, 253603(2010).
[34] X.-R. Yao, W.-K. Yu, X.-F. Liu, L.-Z. Li, M.-F. Li, L.-A. Wu, G.-J. Zhai. Iterative denoising of ghost imaging. Opt. Express, 22, 24268(2014).
[35] K. W. C. Chan, M. N. O’Sullivan, R. W. Boyd. High-order thermal ghost imaging. Opt. Lett., 34, 3343(2009).
[36] L.-H. Ou, L.-M. Kuang. Ghost imaging with third-order correlated thermal light. J. Phys. B, 40, 1833(2007).
[37] W. Gong. High-resolution pseudo-inverse ghost imaging. Photonics Res., 3, 234(2015).
[38] C. Zhang, S. Guo, J. Cao, J. Guan, F. Gao. Object reconstitution using pseudo-inverse for ghost imaging. Opt. Express, 22, 30063(2014).
[39] W. M. Brown. Synthetic aperture radar. IEEE Trans. Aerosp. Electron. Syst., AES-3, 217(1967).
[40] Y. Kang, Y.-P. Yao, Z.-H. Kang, L. Ma, T.-Y. Zhang. Performance analysis of compressive ghost imaging based on different signal reconstruction techniques. J. Opt. Soc. Am. A, 32, 1063(2015).
[41] C. Li. An Efficient Algorithm for Total Variation Regularization with Applications to the Single Pixel Camera and Compressive Sensing(2011).
[42] Z. Wang, A. Bovik, H. Sheikh, E. Simoncelli. Image quality assessment: from error visibility to structural similarity. IEEE Trans. Image Process., 13, 600(2004).
[43] X.-F. Liu, X.-R. Yao, M.-F. Li, W.-K. Yu, X.-H. Chen, Z.-B. Sun, L.-A. Wu, G.-J. Zhai. The role of intensity fluctuations in thermal ghost imaging. Acta Phys. Sin., 62, 184205(2013).
[44] H.-L. Xie, B. Deng, G.-H. Du, Y.-N. Fu, H. Guo, Y.-L. Xue, G.-Y. Peng, F. Tao, L. Zhang, T.-Q. Xiao. Methodology development and application of X-ray imaging beamline at SSRF. Nucl. Sci. Tech., 31, 102(2020).
[45] L. Pan, C. Deng, C. Yu, S. Yue, W. Gong, S. Han. Influence of the sparsity of random speckle illumination on ghost imaging in a noise environment. Chin. Opt. Lett., 19, 041103(2021).
[46] X. Xu, E. Li, X. Shen, S. Han. Optimization of speckle patterns in ghost imaging via sparse constraints by mutual coherence minimization. Chin. Opt. Lett., 13, 071101(2015).
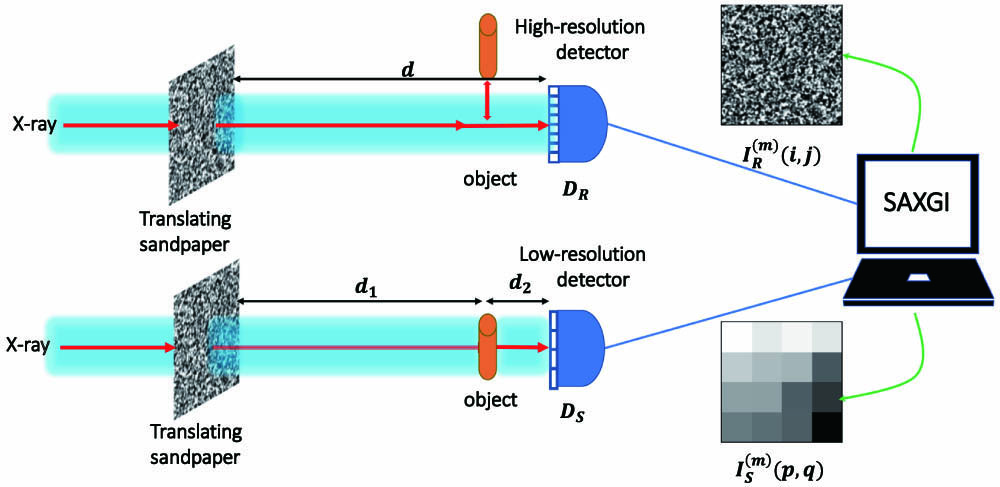
Set citation alerts for the article
Please enter your email address