Wenjun Chen, Chao Cong, Liwen Huang. Convolutional Neural Network Image Feature Measurement Based on Information Entropy[J]. Laser & Optoelectronics Progress, 2021, 58(22): 2211004

Search by keywords or author
- Laser & Optoelectronics Progress
- Vol. 58, Issue 22, 2211004 (2021)
Abstract
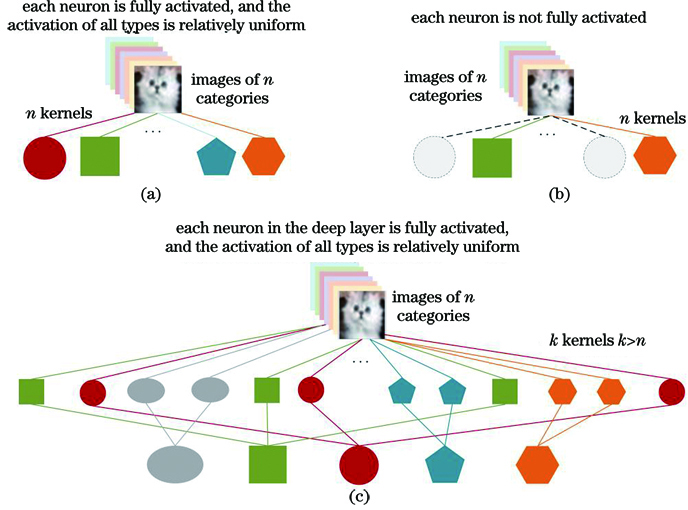
Set citation alerts for the article
Please enter your email address