
- Photonics Research
- Vol. 10, Issue 4, 939 (2022)
Abstract
1. INTRODUCTION
Over the past few years, the rapid development of artificial intelligence has revolutionized many aspects of our lives, with applications ranging from image recognition [1] to cancer diagnosis [2]. Meanwhile, machine learning itself has also posed great challenges to the traditional von Neumann computing architecture due to the massively distributed information processing fashion [3]. Taking inspiration from the extraordinary computational capacities of human brains [4], neuromorphic computing has emerged as a promising candidate for the next generation intelligent computing paradigm. Currently available electronic neuromorphic systems, e.g., TrueNorth [5], Loihi [6], Neurogrid [7], and SpiNNaker [8], have demonstrated significant performance enhancement in both power efficiency and computing speed, but still face intrinsic bottlenecks in terms of reduced bandwidth, limited speed, and large multicasting [9]. Benefitting from the unique advantages of optical devices, e.g., high parallelism, broad bandwidth, low cross talk, and large integration, photonic neuromorphic systems are capable of, in theory, outperforming state-of-the-art neuromorphic electronics with several orders-of-magnitude faster operation speed as well as far less power consumption [10]. Since the pioneering work in the early 1990s [11,12], considerable efforts have been aimed at hardware implementations of photonic neurons, either realizing the nonlinear activation functions or emulating the all-or-none dynamic response [3,9,13]. Notably, spiking neurons can process information in a more biologically computational fashion by exploring the sparse coding strategy and event-driven nature of neural systems, thus rendering an energy-efficient computing system that combines both the noise robustness of digital computation and the bandwidth efficiency of analog communication [14]. The past decade has witnessed a bloom of diverse photonic spiking neurons. Due to the similar underlying excitability mechanisms with biological neurons [15], semiconductor lasers of different types have been the most widely employed approaches, including microrings [16], microdisks [17,18], micropillars [19,20], quantum dots [21–23], two sections with saturable absorber regions [24,25], distributed feedback lasers [26], and vertical-cavity surface-emitting lasers (VCSELs) [27–33]. There are also other novel photonic demonstrations of spiking neural models, e.g., semiconductor optical amplifiers (SOAs) [34], microring modulators [35], and microring resonators with phase change materials [36,37].
Although impressive performance has been numerically and experimentally demonstrated with the aforementioned schemes, the necessary use of active components leads to poor compatibility with the mutual complementary metal–oxide–semiconductor (CMOS) fabrication process, making it difficult for large-scale integrated photonic neuromorphic systems. In our previous work [38], we have theoretically analyzed the nonlinearity of passive microresonators in the silicon-on-insulator (SOI) platform, which can be utilized to realize the spiking dynamics of photonic neurons. Here, we further provide systematic experimental studies of a microring spiking neuron. The discontinuous relation between oscillation frequency and optical pump power of self-pulsation offers direct evidence that the silicon microring belongs to the class II resonate-and-fire spiking neuron, which is further confirmed by subthreshold oscillations and the existence of a thin threshold region of excitability. Moreover, the all-optical microring spiking neuron does not exhibit a typical refractory period by engineering the perturbation power much higher than the pump power. As a result, its operation speed is limited only by the excited pulse width and can reach up to approximately sub-gigahertz (GHz), much faster than prior work [39]. More importantly, we report the first experimental demonstration of temporal integration and inhibitory dynamics in passive microresonators, to the best of our knowledge. These novel spiking regimes may potentially pave the way toward large-scale photonic neural networks.
2. DESIGN OF ALL-OPTICAL SILICON SPIKING NEURON AND EXPERIMENTAL SETUP
Biological neurons are capable of exhibiting a rich diversity of spiking activities, the simplest of which can be categorized into two classes. Table 1 provides a detailed summary of the most important neurocomputational properties [4]. In general, integrators feature a well-defined firing threshold and all-or-none spiking dynamics. When the perturbation strength is below the threshold, no spikes can be observed, whereas spike events with relatively constant amplitude will be generated beyond a certain value. Furthermore, the firing rate of integrators can be flexibly tailored by adjusting the input strength, indicating a rate coding scheme. On the other side, resonators feature fast subthreshold amplitude oscillations and typically exhibit a threshold area. Within a certain power range, external stimuli can excite resonators to produce spike events with varying amplitudes, depending on the perturbation strength. Distinct from the cases of integrators, the firing rate of resonators is limited to a specific frequency band, which is determined by the subthreshold oscillations. Moreover, resonators are able to present frequency preferences by responding to perturbations that are weak but resonant with the nature subthreshold oscillation frequency. It should be noted that integrators are ideal candidates for neuromorphic information processing. Resonators, however, have also attracted much research interest and may find important applications in selective communication among different neuron groups [40]. In fact, there has been evidence of both integrators and resonators in human brains.
Summary of Major Neurocomputational Properties [
Properties | Integrators | Resonators | ||
---|---|---|---|---|
Bifurcation | Saddle-node on invariant circle | Saddle-node | Subcritical Andronov–Hopf | Supercritical Andronov–Hopf |
Excitability | Class I | Class II | Class II | |
Bistability | No | Yes | Yes | No |
Threshold | Well defined | May not be defined | ||
Subthreshold oscillations | No | Yes | ||
All-or-none spiking | Yes | No | ||
Frequency preference | No | Yes |
Sign up for Photonics Research TOC. Get the latest issue of Photonics Research delivered right to you!Sign up now
There has been long-held research interest in the nonlinearity of microresonators to manipulate light with light. Due to the free carrier dispersion (FCD) effect, the free carriers generated by two-photon absorption (TPA) induce a blueshift to the resonance wavelength. Meanwhile, the absorbed optical energy by free carrier absorption (FCA) is mainly lost by heat, which leads to a redshift in the resonance wavelength owing to the thermo-optic (TO) effect. However, the relaxation process of heat is at least one order of magnitude slower than free carriers. As a result, the interplay between thermal and free carriers contributes to diverse nonlinearities in microresonators. To characterize this nonlinear process, we have proposed a universal coupled mode theory (CMT) model for all passive microresonators [38], which can output “negative” pulses when applying strong enough perturbations. Since information in spike processing is contained in the presence timing of spikes, regardless of their shapes, microresonators are still able to successfully emulate the spiking dynamics of photonic neurons, e.g., excitability, temporal integration, and inhibition. Moreover, the phase-plane analysis has revealed that the excitability of passive microresonators results from subcritical Andronov–Hopf bifurcation [39], indicating class II neural excitability. Although nonlinearities in a silicon microring have been extensively investigated in experiments [39,41,42], there has been little systematic research work concentrating on its characteristics from the perspective of a spiking neuron.
To fully explore the potential of a passive microring neuron, we have designed an all-pass silicon microring with a radius of 7 μm and a cross section of
Figure 1.(a) Microscope image of the silicon microring used in the experiment. (b) Schematic figure of the measurement setup for both characterization of the microring and investigation of its spiking dynamics. TLS, tunable laser source; PC, polarization controller; DUT, device under test; OSA, optical spectrum analyzer; CR, circulator; EDFA, erbium-doped fiber amplifier; BPF, bandpass filter; PD, photodetector; AWG, arbitrary waveform generator; OSC, oscilloscope; EOM, electro-optical modulator. (c) Non-normalized transmission of the microring. (d) Transmission of one single resonance and its Lorentz curve.
The experimental setup to further study the temporal dynamics of the silicon microring neuron is also presented in Fig. 1(b). The coupling loss between single mode fibers and grating couplers is optimized to be
3. RESONATE-AND-FIRE MICRORING SPIKING NEURON
A. Self-Pulsation
Considering that silicon microrings are able to exhibit self-pulsation at both negative and positive detunings from their resonance wavelengths, in our experiments, we detune the wavelength of pump light at
Figure 2.(a) Measured real-time output waveform of self-pulsation for different input powers. (b) Frequency of self-pulsation in relation to pump power.
B. Excitability
To investigate the excitability behavior of a microring spiking neuron, the optical pump power is fixed at
Figure 3.(a) Measured real-time output waveform of excitability in response to perturbation pulses of increasing power. (b) Normalized strength of the “negative” spike as a function of perturbation power. The threshold area is indicated by the shaded region.
The frequency detuning of the pump and perturbation light has little impact on the shape and power of microring neuron spikes. We first numerically study this with the CMT model [38], where the wavelengths of the pump and perturbation signals are set to be the same and detuned from the resonance
Figure 4.(a) Simulated and (b) measured microring neuron spikes for different wavelength detuning values of the pump and perturbation light.
C. Refractory Period
There are two types of refractory periods in excitable systems: the absolute refractory period, during which excitations are completely inhibited, and the relative refractive period, during which subthreshold spikes can be excited. The refractory period allows the spiking neuron to relax to its steady state, hence enabling repeatable spiking. However, its existence also sets the maximum operation speed of spike processing. Although the refractory period in a silicon microring has already been theoretically predicted [38] and experimentally demonstrated [39], the power of the perturbation light is typically limited to be lower than that of the pump light. In fact, the excitability threshold distribution of the microring neuron can be divided into three parts, as shown in Fig. 5(a). In region I, the microring neuron can be excited with perturbation power below the pump power but above the threshold; in region II, the microring neuron can be excited only when the perturbation power is much higher than the pump power. The microring neuron exhibits self-pulsation in region III. Previous studies have mainly focused on region I, where the operation speed of the microring neuron is constrained by its refractory period. Here, we numerically demonstrate that the refractory period can be suppressed by increasing perturbation power. In the simulation, two perturbation pulses with a time interval of 80 ns are used to trigger the microring neuron. As shown in Fig. 5(b), for
Figure 5.(a) Distribution of the excitability threshold for the microring neuron over different pump powers and wavelength detuning. (b) For
Figure 6.Measured real-time output waveform of refractory period with varying time intervals between two perturbation pulses. Each pulse is strong enough to excite the silicon microring neuron to emit a complete spike.
D. Temporal Integration
Temporal integration of a spiking neuron refers to the ability to integrate several closely spaced stimuli in time, and produce a complete output spike once the accumulated potential exceeds the excitability threshold. This property can be exploited to perform coincidence detection, which is the fundamental process in various spatial–temporal recognition tasks. To investigate this feature of the silicon microring neuron, we program a perturbation pattern consisting of a single rectangular pulse followed by two closely spaced pulses with varying time intervals. Each individual perturbation pulse is below the excitation threshold and yields only a subthreshold oscillation. As demonstrated in Fig. 7, two pulses with a center-to-center time duration of 18 ns can trigger a subthreshold spike with strength similar to a single pulse. When the time interval between two pulses shrinks to 10 ns, a deeper output spike can be excited with improved strength compared to a single pulse. Leaky integration is indicated by the recovery process between two dips. If the temporal separation further decreases to 5 ns, a single complete output spike with significant strength enhancement is produced on the arrival of two perturbation pulses. Obviously, the microring spiking neuron is able to exhibit temporal integration of multiple external stimuli.
Figure 7.Measured real-time output waveform of temporal integration with varying time intervals between two perturbation pulses. Only a subthreshold spike can be triggered by each single pulse.
E. Inhibitory Dynamics
Both excitatory and inhibitory dynamics play important roles in spike-time-dependent plasticity (STDP) [43], which offers an efficient learning mechanism for forming and modifying neuromorphic computing systems. Previously, inhibition has been experimentally demonstrated in VCSELs [25,29,31], a quantum-dot mode-locked laser [21], and a microring modulator neuron [35], but remains unexplored in the research field of passive microresonators. The experimental results of controllable inhibitory dynamics in a microring are shown in Fig. 8. We program the AWG to obtain a 15 ns excitatory pattern with a high level of 2.2 and a 15 ns inhibitory pattern with a low level of
Figure 8.Measured real-time output waveform of inhibitory dynamics with varying time intervals between the excitatory and inhibitory pattens.
4. CONCLUSION
In summary, we systematically investigate the fundamental spiking regimes of an all-optical microring neuron with both numerical and experimental results, i.e., self-pulsation, excitability, refractory period, temporal integration, and inhibitory dynamics. The fact that the passive microring behaves like a class II resonate-and-fire neuron has been verified by the discontinuous frequency–power relation of self-pulsation, subthreshold oscillations, and a narrow excitation threshold region. The refractory period of the microring neuron has been successfully suppressed by engineering perturbation power much higher than pump power, thus promising an operation speed up to roughly sub-GHz. Furthermore, temporal integration and inhibitory dynamics are experimentally demonstrated in a passive microresonator for the first time. Last but not least, our silicon microring spiking neuron is fully compatible with the standard CMOS process, thus paving the way for large-scale integrated spiking neural networks.
References
[1] K. He, X. Zhang, S. Ren, J. Sun. Deep residual learning for image recognition. IEEE Conference on Computer Vision and Pattern Recognition, 770-778(2016).
[2] R. Fakoor, F. Ladhak, A. Nazi, M. Huber. Using deep learning to enhance cancer diagnosis and classification. International Conference on Machine Learning, 3937-3949(2013).
[3] B. J. Shastri, A. N. Tait, T. F. de Lima, W. H. Pernice, H. Bhaskaran, C. D. Wright, P. R. Prucnal. Photonics for artificial intelligence and neuromorphic computing. Nat. Photonics, 15, 102-114(2021).
[4] E. M. Izhikevich. Dynamical Systems in Neuroscience(2007).
[5] F. Akopyan, J. Sawada, A. Cassidy, R. Alvarez-Icaza, J. Arthur, P. Merolla, N. Imam, Y. Nakamura, P. Datta, G.-J. Nam, B. Taba, M. Beakes, B. Brezzo, J. B. Kuang, R. Manohar, W. P. Risk, B. Jackson, D. S. Modha. TrueNorth: design and tool flow of a 65 mW 1 million neuron programmable neurosynaptic chip. IEEE Trans. Computer-Aided Design Integr. Circuits Syst., 34, 1537-1557(2015).
[6] M. Davies, N. Srinivasa, T.-H. Lin, G. Chinya, Y. Cao, S. H. Choday, G. Dimou, P. Joshi, N. Imam, S. Jain, Y. Liao, C.-K. Lin, A. Lines, R. Liu, D. Mathaikutty, S. McCoy, A. Paul, J. Tse, G. Venkataramanan, Y.-H. Weng, A. Wild, Y. Yang, H. Wang. Loihi: a neuromorphic manycore processor with on-chip learning. IEEE Micro, 38, 82-99(2018).
[7] D. Khodagholy, J. N. Gelinas, T. Thesen, W. Doyle, O. Devinsky, G. G. Malliaras, G. Buzsáki. NeuroGrid: recording action potentials from the surface of the brain. Nat. Neurosci., 18, 310-315(2015).
[8] S. B. Furber, F. Galluppi, S. Temple, L. A. Plana. The spiNNaker project. Proc. IEEE, 102, 652-665(2014).
[9] P. R. Prucnal, B. J. Shastri, T. F. de Lima, M. A. Nahmias, A. N. Tait. Recent progress in semiconductor excitable lasers for photonic spike processing. Adv. Opt. Photon., 8, 228-299(2016).
[10] P. R. Prucnal, B. J. Shastri, M. C. Teich. Neuromorphic Photonics(2017).
[11] C.-H. Wang, B. K. Jenkins. Subtracting incoherent optical neuron model: analysis, experiment, and applications. Appl. Opt., 29, 2171-2186(1990).
[12] S. Tariq, M. K. Habib, H. A. Helmy. Opto-electronic neuron-type operation via stimulated Raman scattering in optical fiber. J. Lightwave Technol., 15, 938-947(1997).
[13] X. Guo, J. Xiang, Y. Zhang, Y. Su. Integrated neuromorphic photonics: synapses, neurons, and neural networks. Adv. Photon. Res., 2, 2000212(2021).
[14] R. Sarpeshkar. Analog versus digital: extrapolating from electronics to neurobiology. Neural Comput., 10, 1601-1638(1998).
[15] M. A. Nahmias, B. J. Shastri, A. N. Tait, P. R. Prucnal. A leaky integrate-and-fire laser neuron for ultrafast cognitive computing. IEEE J. Sel. Top. Quantum Electron., 19, 1800212(2013).
[16] W. Coomans, L. Gelens, S. Beri, J. Danckaert, G. Van der Sande. Solitary and coupled semiconductor ring lasers as optical spiking neurons. Phys. Rev. E, 84, 036209(2011).
[17] K. Alexander, T. Van Vaerenbergh, M. Fiers, P. Mechet, J. Dambre, P. Bienstman. Excitability in optically injected microdisk lasers with phase controlled excitatory and inhibitory response. Opt. Express, 21, 26182-26191(2013).
[18] L. Gelens, L. Mashal, S. Beri, W. Coomans, G. Van der Sande, J. Danckaert, G. Verschaffelt. Excitability in semiconductor microring lasers: experimental and theoretical pulse characterization. Phys. Rev. A, 82, 063841(2010).
[19] F. Selmi, R. Braive, G. Beaudoin, I. Sagnes, R. Kuszelewicz, S. Barbay. Relative refractory period in an excitable semiconductor laser. Phys. Rev. Lett., 112, 183902(2014).
[20] F. Selmi, R. Braive, G. Beaudoin, I. Sagnes, R. Kuszelewicz, S. Barbay. Temporal summation in a neuromimetic micropillar laser. Opt. Lett., 40, 5690-5693(2015).
[21] J. Robertson, T. Ackemann, L. F. Lester, A. Hurtado. Externally-triggered activation and inhibition of optical pulsating regimes in quantum-dot mode-locked lasers. Sci. Rep., 8, 12515(2018).
[22] G. Sarantoglou, M. Skontranis, C. Mesaritakis. All optical integrate and fire neuromorphic node based on single section quantum dot laser. IEEE J. Sel. Top. Quantum Electron., 26, 1900310(2019).
[23] G. Sarantoglou, M. Skontranis, A. Bogris, C. Mesaritakis. Experimental study of neuromorphic node based on a multiwaveband emitting two-section quantum dot laser. Photon. Res., 9, B87-B95(2021).
[24] B. J. Shastri, M. A. Nahmias, A. N. Tait, A. W. Rodriguez, B. Wu, P. R. Prucnal. Spike processing with a graphene excitable laser. Sci. Rep., 6, 19126(2016).
[25] P. Y. Ma, B. J. Shastri, T. F. De Lima, C. Huang, A. N. Tait, M. A. Nahmias, H.-T. Peng, P. R. Prucnal. Simultaneous excitatory and inhibitory dynamics in an excitable laser. Opt. Lett., 43, 3802-3805(2018).
[26] H.-T. Peng, G. Angelatos, T. F. de Lima, M. A. Nahmias, A. N. Tait, S. Abbaslou, B. J. Shastri, P. R. Prucnal. Temporal information processing with an integrated laser neuron. IEEE J. Sel. Top. Quantum Electron., 26, 5100209(2019).
[27] A. Hurtado, K. Schires, I. Henning, M. Adams. Investigation of vertical cavity surface emitting laser dynamics for neuromorphic photonic systems. Appl. Phys. Lett., 100, 103703(2012).
[28] J. Robertson, E. Wade, Y. Kopp, J. Bueno, A. Hurtado. Toward neuromorphic photonic networks of ultrafast spiking laser neurons. IEEE J. Sel. Top. Quantum Electron., 26, 7700715(2019).
[29] J. Robertson, T. Deng, J. Javaloyes, A. Hurtado. Controlled inhibition of spiking dynamics in VCSELs for neuromorphic photonics: theory and experiments. Opt. Lett., 42, 1560-1563(2017).
[30] M. Hejda, J. Robertson, J. Bueno, J. A. Alanis, A. Hurtado. Neuromorphic encoding of image pixel data into rate-coded optical spike trains with a photonic VCSEL-neuron. APL Photon., 6, 060802(2021).
[31] Y. Zhang, S. Xiang, X. Guo, A. Wen, Y. Hao. All-optical inhibitory dynamics in photonic neuron based on polarization mode competition in a VCSEL with an embedded saturable absorber. Opt. Lett., 44, 1548-1551(2019).
[32] S. Xiang, Z. Ren, Y. Zhang, Z. Song, Y. Hao. All-optical neuromorphic XOR operation with inhibitory dynamics of a single photonic spiking neuron based on a VCSEL-SA. Opt. Lett., 45, 1104-1107(2020).
[33] Y. Zhang, J. Robertson, S. Xiang, M. Hejda, J. Bueno, A. Hurtado. All-optical neuromorphic binary convolution with a spiking VCSEL neuron for image gradient magnitudes. Photon. Res., 9, B201-B209(2021).
[34] K. Kravtsov, M. P. Fok, D. Rosenbluth, P. R. Prucnal. Ultrafast all-optical implementation of a leaky integrate-and-fire neuron. Opt. Express, 19, 2133-2147(2011).
[35] A. N. Tait, T. F. De Lima, M. A. Nahmias, H. B. Miller, H.-T. Peng, B. J. Shastri, P. R. Prucnal. Silicon photonic modulator neuron. Phys. Rev. Appl., 11, 064043(2019).
[36] I. Chakraborty, G. Saha, K. Roy. Photonic in-memory computing primitive for spiking neural networks using phase-change materials. Phys. Rev. Appl., 11, 014063(2019).
[37] J. Feldmann, N. Youngblood, C. D. Wright, H. Bhaskaran, W. H. Pernice. All-optical spiking neurosynaptic networks with self-learning capabilities. Nature, 569, 208-214(2019).
[38] J. Xiang, A. Torchy, X. Guo, Y. Su. All-optical spiking neuron based on passive microresonator. J. Lightwave Technol., 38, 4019-4029(2020).
[39] T. Van Vaerenbergh, M. Fiers, P. Mechet, T. Spuesens, R. Kumar, G. Morthier, B. Schrauwen, J. Dambre, P. Bienstman. Cascadable excitability in microrings. Opt. Express, 20, 20292-20308(2012).
[40] F. C. Hoppensteadt, E. M. Izhikevich. Weakly Connected Neural Networks, 126(2012).
[41] L. Zhang, Y. Fei, Y. Cao, X. Lei, S. Chen. Experimental observations of thermo-optical bistability and self-pulsation in silicon microring resonators. J. Opt. Soc. Am. B, 31, 201-206(2014).
[42] M. Borghi, D. Bazzanella, M. Mancinelli, L. Pavesi. On the modeling of thermal and free carrier nonlinearities in silicon-on-insulator microring resonators. Opt. Express, 29, 4363-4377(2021).
[43] G.-Q. Bi, M.-M. Poo. Synaptic modifications in cultured hippocampal neurons: dependence on spike timing, synaptic strength, and postsynaptic cell type. J. Neurosci., 18, 10464-10472(1998).
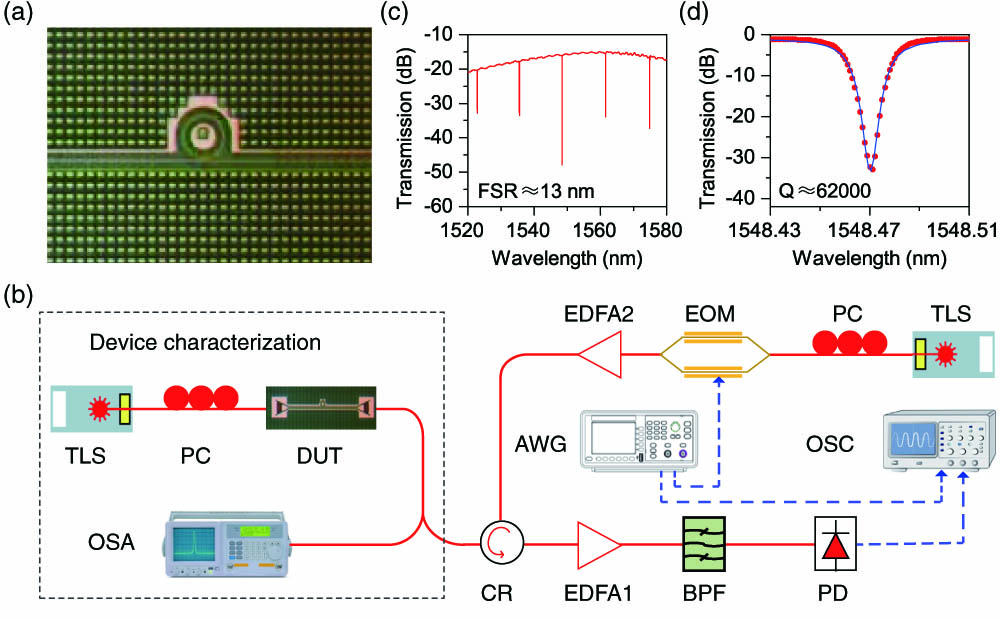
Set citation alerts for the article
Please enter your email address