Tang Yi, Nian Yongjian, He Mi, Wang Qiannan, Xu Ke. Successive spectral unmixing for hyperspectral images based on L1/2 regularization[J]. Infrared and Laser Engineering, 2019, 48(7): 726003

Search by keywords or author
- Infrared and Laser Engineering
- Vol. 48, Issue 7, 726003 (2019)
Abstract
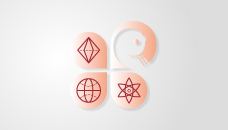
Set citation alerts for the article
Please enter your email address