Shengjie Du, Xiaofen Jia, Yourui Huang, Yongcun Guo, Baiting Zhao. High efficient activation function design for CNN model image classification task[J]. Infrared and Laser Engineering, 2022, 51(3): 20210253

Search by keywords or author
- Infrared and Laser Engineering
- Vol. 51, Issue 3, 20210253 (2022)
Abstract
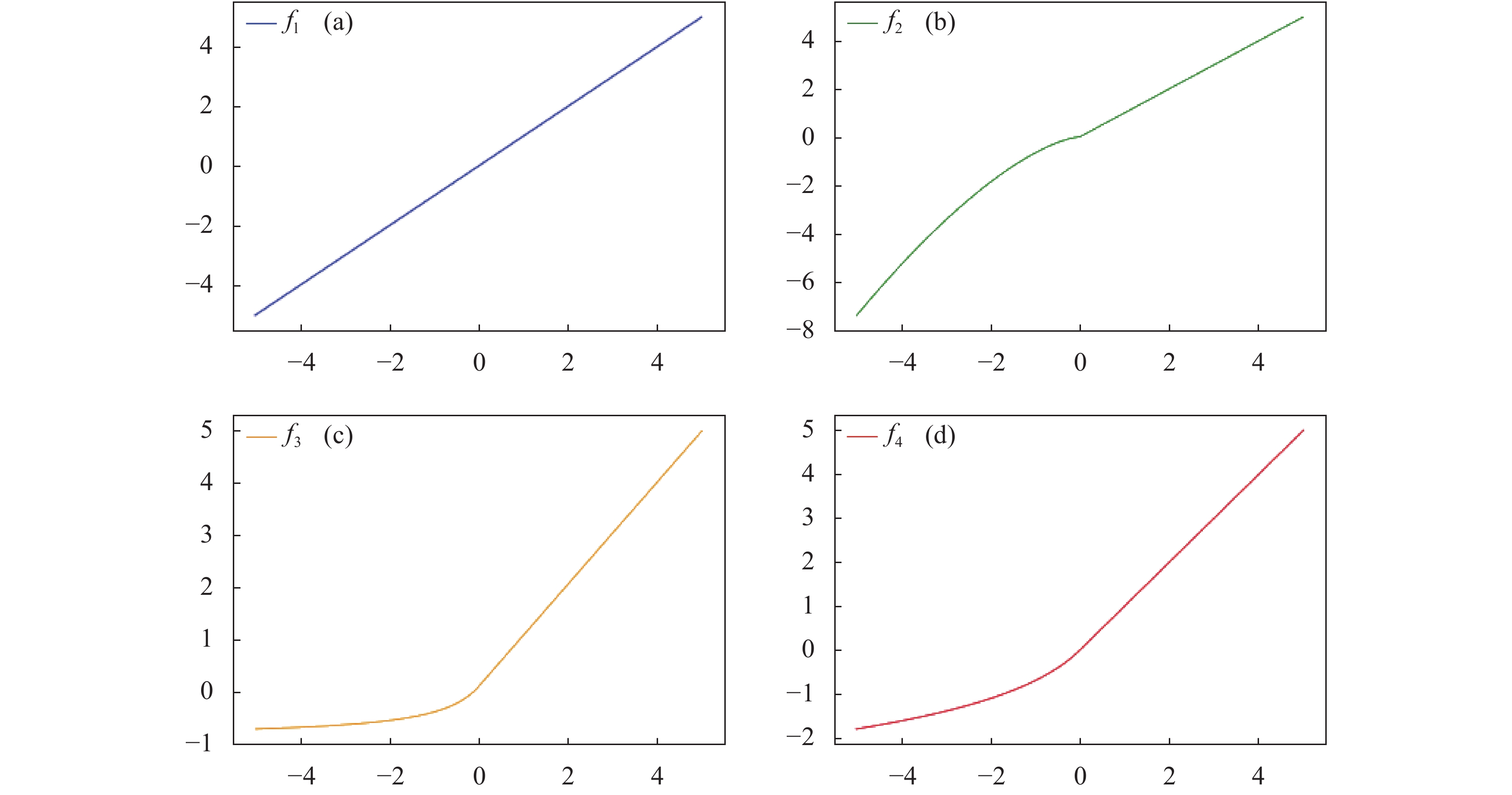
Set citation alerts for the article
Please enter your email address