Pavel S. Anisimov, Evgeny D. Tsyplakov, Viacheslav V. Zemlyakov, Jiexing Gao, "Speckle backpropagation for compensation of nonlinear effects in few-mode optical fibers," Chin. Opt. Lett. 21, 030601 (2023)

Search by keywords or author
- Chinese Optics Letters
- Vol. 21, Issue 3, 030601 (2023)
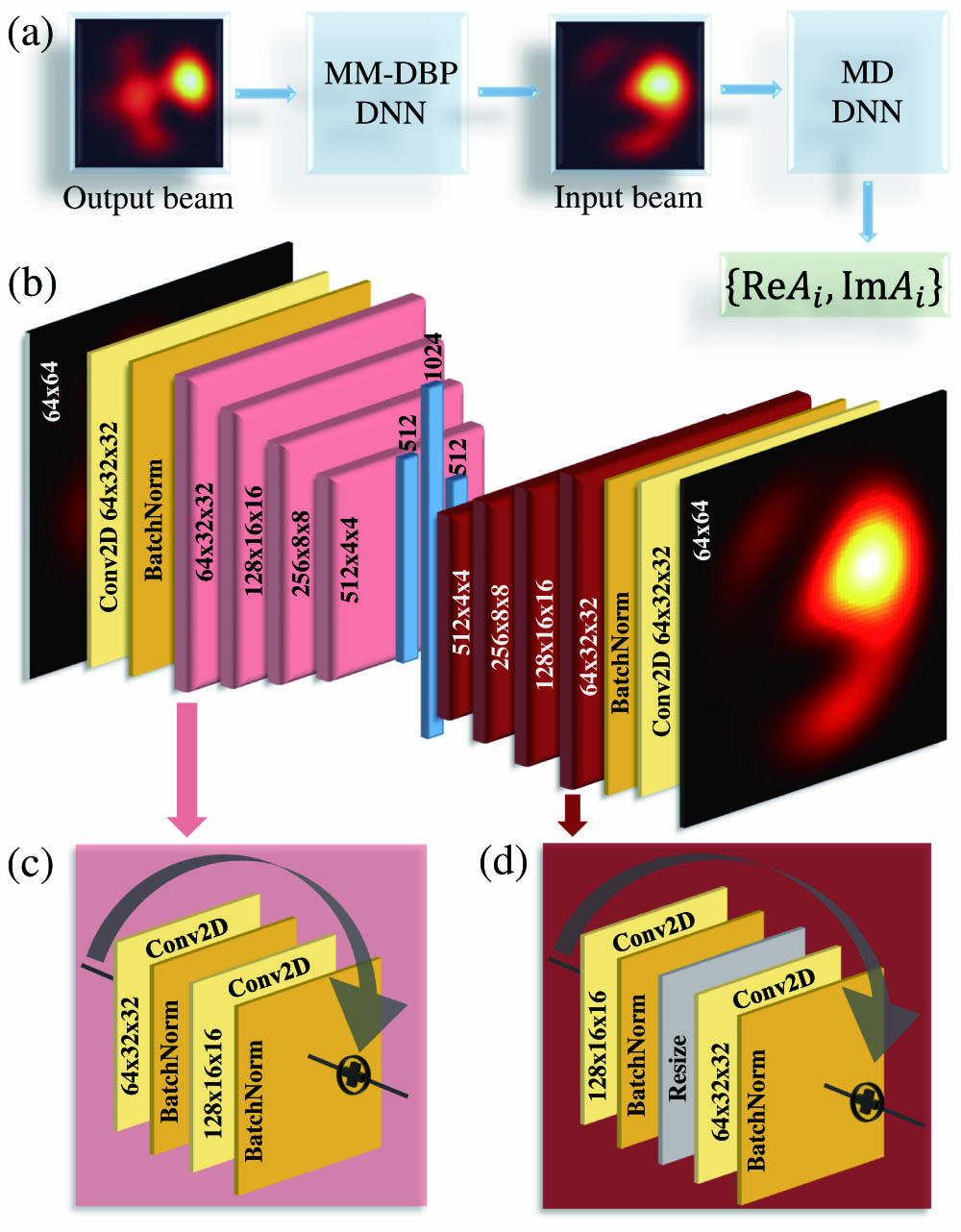
Fig. 1. (a) Flow chart of the proposed method. A near-field beam speckle, captured at the fiber output, is fed to MM-DBP DNN, resulting in the recovered input pattern. MD-DNN takes this input pattern and yields real and imaginary parts of complex mode coefficients. (b) Schematic of the proposed MM-DBP DNN. We use a residual neural network (ResNet) based autoencoder that compresses information acquired from the speckles and maps it onto a vector from the latent feature space. The decoder maps it back to the speckle space. Three middle blue blocks denote fully connected (FC) layers with 512, 1024, and 512 neurons, respectively. After each FC layer, we also place a dropout layer. BatchNorm stands for batch normalization. We use the rectified linear unit (ReLu) as the activation function. (c), (d) Detailed structure of the encoder and the decoder basic blocks, respectively. Those with other dimensions can be obtained analogously.
![(a) Example of an output, initial, and recovered speckle, respectively, in the absence of noise [see Eq. (6)]; (b) same patterns with receiver noise included (SNR = 10 dB); (c) energy redistribution for each mode after having propagated the fiber.](/richHtml/col/2023/21/3/030601/img_002.jpg)
Fig. 2. (a) Example of an output, initial, and recovered speckle, respectively, in the absence of noise [see Eq. (6 )]; (b) same patterns with receiver noise included (SNR = 10 dB); (c) energy redistribution for each mode after having propagated the fiber.
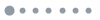
Fig. 3. (a) MSE and (b) C versus SNR. Each point represents averaging over 1000 samples from the validation data set.
|
Table 1. Parameters Used in Simulations of Nonlinear Light Propagation in FMFs
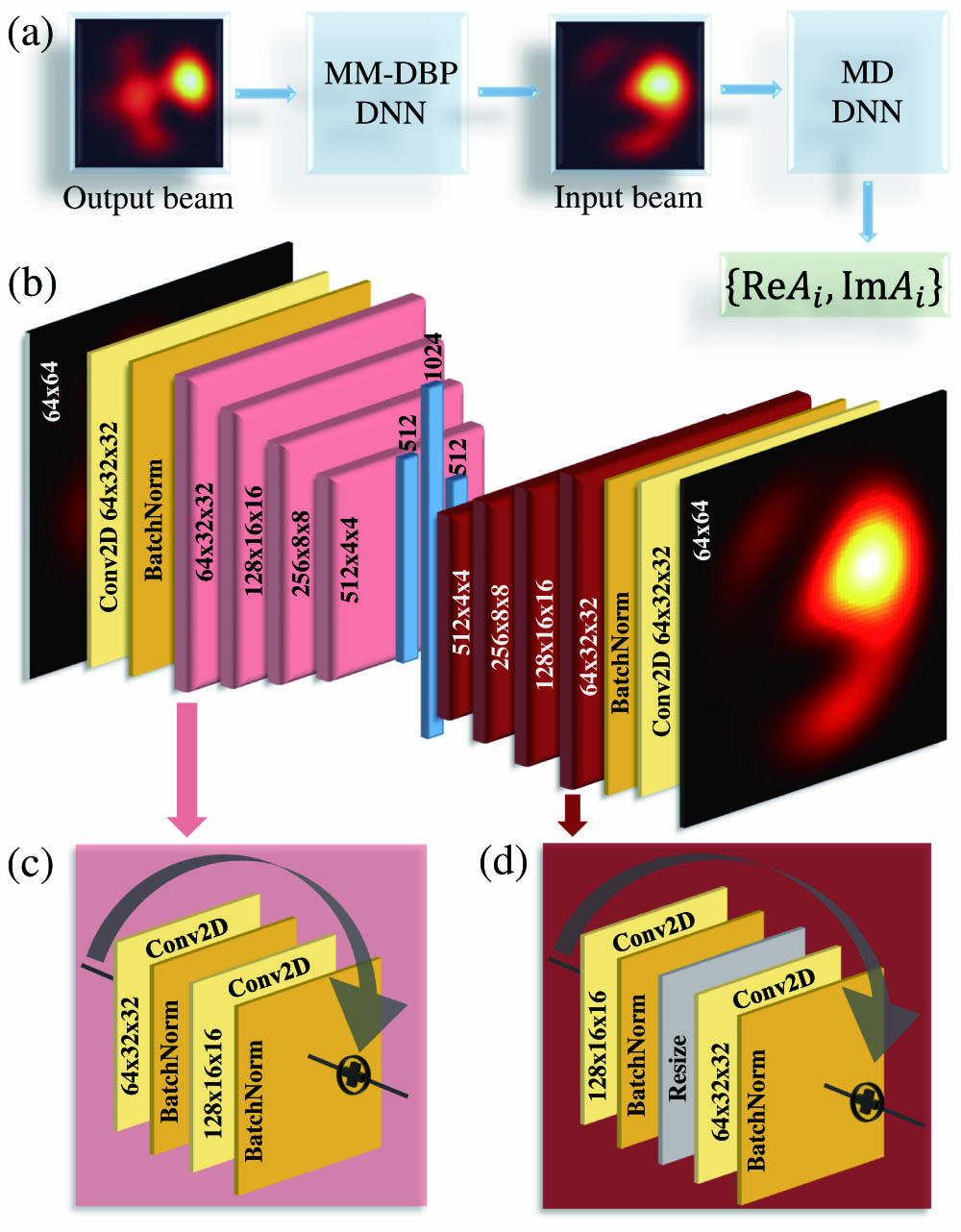
Set citation alerts for the article
Please enter your email address