YANG Yong, YUE Jian-hua, LI Jing, ZHANG He-rui. Online Discrimination Model for Mine Water Inrush Source Based CNN and Fluorescence Spectrum[J]. Spectroscopy and Spectral Analysis, 2019, 39(8): 2425

Search by keywords or author
- Spectroscopy and Spectral Analysis
- Vol. 39, Issue 8, 2425 (2019)
Abstract
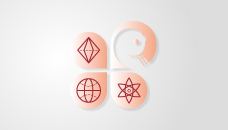
Set citation alerts for the article
Please enter your email address