Yichao Wang, Zheng Zhang, Haizhou Huang, Wenxiong Lin. Particle auto-statistics and measurement of the spherical powder for 3D printing based on deep learning[J]. Infrared and Laser Engineering, 2021, 50(10): 2021G004

Search by keywords or author
- Infrared and Laser Engineering
- Vol. 50, Issue 10, 2021G004 (2021)

Fig. 1. Flowchart of the powder microscopy image automatic analysis system
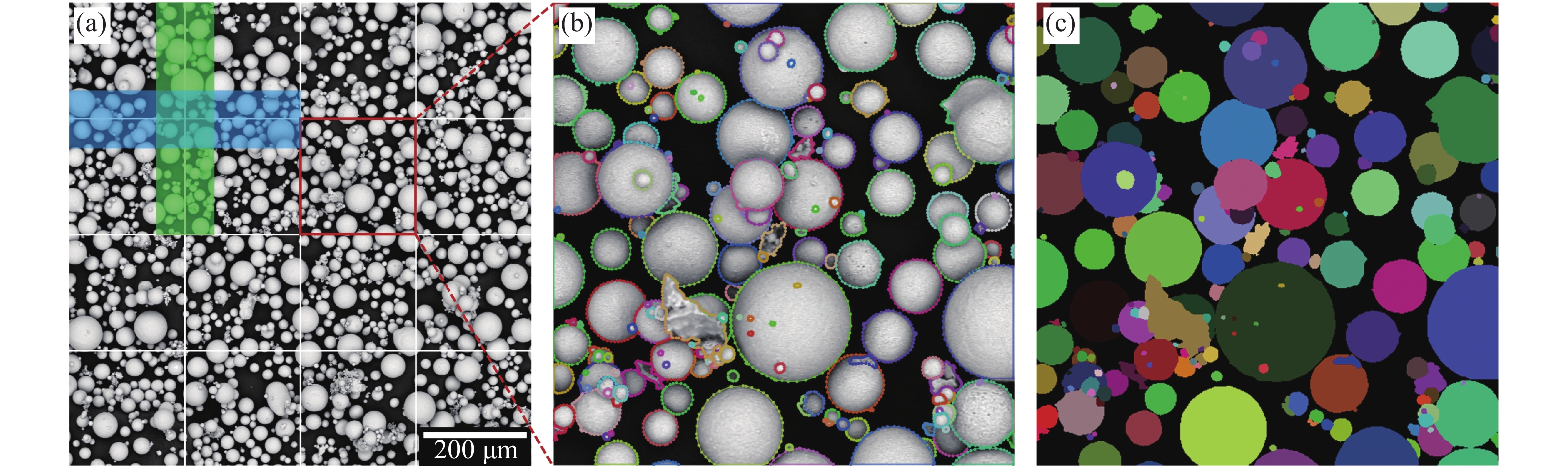
Fig. 2. (a) Original SEM image (2 048×2 048 pixel), which is cropped into 16 parts; (b) Characteristic image labeled with LabelMe(512×512 pixel); (c) The corresponding image mask of (b)
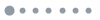
Fig. 3. Loss-epoch curve during train process
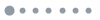
Fig. 4. Flowchart of transferring and rough merging process of one sub-image
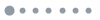
Fig. 5. Illustration of two kinds of IoU & IoS in rough merging and precise merging processes, respectively. (a) IoU & IoS of two circumscribed rectangles; (b) IoU & IoS of two masks; (c) One example of the usage of IoS
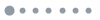
Fig. 6. (a) Illustration of particle boundary smoothing and error compensation; (b) Fitted perimeter and area residual function based on scattered deviation values of standard circles
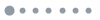
Fig. 7. Predicted results and comparation with the Phenom ProSuite Software Particlemetric. (a) Raw image; (b) Output segmentation result of Particlemetric; (c) Four enlarged details region of (b); (d) Output result of proposed method; (e) Four enlarged details region of (d)
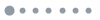
Fig. 8. Statistical analysis results and comparation. (a) PSD results measured by the Particlemetric, our method and laser diffraction technique, respectively; (b) Degree of sphericity distribution (DSD) results measured by Particlemetric and proposed method

Set citation alerts for the article
Please enter your email address