Sergey Krasikov, Aaron Tranter, Andrey Bogdanov, Yuri Kivshar. Intelligent metaphotonics empowered by machine learning[J]. Opto-Electronic Advances, 2022, 5(3): 210147

Search by keywords or author
- Opto-Electronic Advances
- Vol. 5, Issue 3, 210147 (2022)
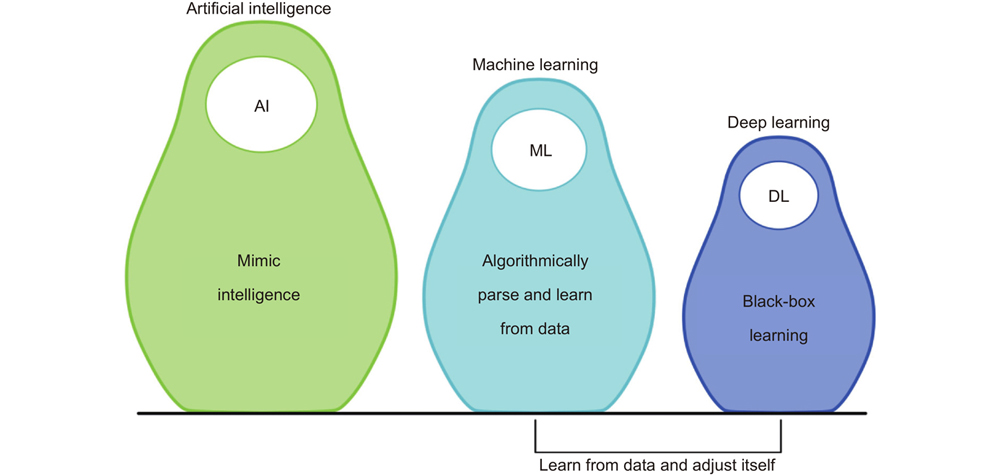
Fig. 1. Links of different ML-based concepts. Artificial intelligence (AI) is a part of computer science dedicated to development of ways to mimic general intelligence. Machine learning (ML) is a subset of AI, these are data-driven algorithms which learn from experience and have the capacity to improve their performance over time and adapt to new data. ML algorithms are varied in their approach, however deep learning (DL) is a subset of ML solely based on layered structures referred to as artificial neural networks. The main feature of DL is the capability to efficiently process raw unstructured data and automatically determine its features while classical ML involves the processing of data in a manner predefined by a human operator.
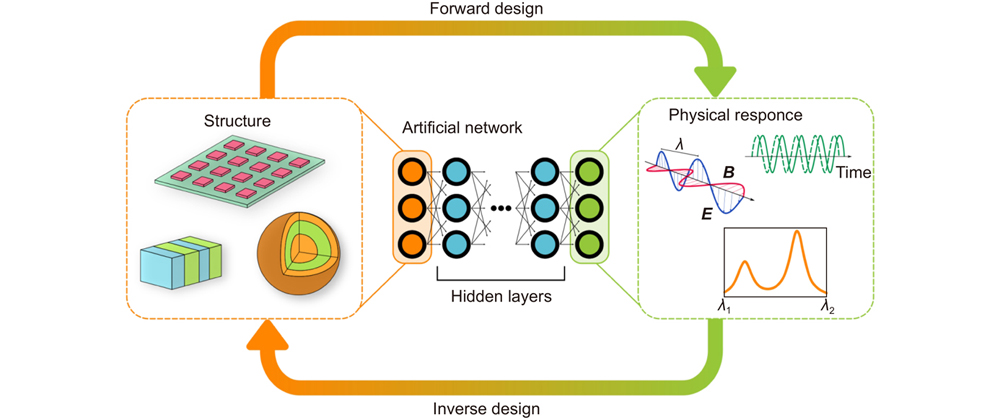
Fig. 2. Inverse and forward designs based on DL techniques. An artificial neural network is fed by parameters of a structure and the corresponding physical response is obtained with conventional numerical techniques or from an experiment. Structure parameters may come in a form of numerical arrays containing geometric or material parameters, or in a form of images or sets of pixels. During the training procedure the neural network determines the appropriate mapping to account for the relation between the parameters and the responses. Then, the network can predict a structure response which was not present in the learning set of data – this is a forward design, which is a prediction of a physical response for a given structure. Swapping the input and output data and applying the similar training procedure, the network may provide a reverse function, predicting parameters of a structure which allows achieving a given physical response, called an inverse design.
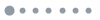
Fig. 3. ML-empowered designs of multilayer nanoantennas. (a ) Schematic of the design procedure based on the DL approaches. A DL algorithm (a black box) connects physical response with parameters of a structure. For example, hand-drawn scattering spectra can be processed with the black-box in order to define materials and thicknesses of the layers needed to achieve target spectra (Based on the results from ref.38). (b ) Demonstration of invisibility-to-superscattering transition of a multilayer sphere made of phase-change materials. Materials and thicknesses for this case are found similar to the scheme in (a)48. (c ) Transfer learning process. Layers of one trained ANN can be merged with another ANN to provide a training procedure for another type of the structure. This might be used for a design of multilayer films or multilayer sphere with using the ANN approach (Concept originates from the results of refs.52, 53). Figure reproduced with permission from: (b) ref.48, Optica Publishing Group.
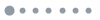
Fig. 4. ML-empowered designs of transformative metasurfaces. (a ) General scheme of the inverse design implemented for metasurfaces. Representation of metasurface elements can be done in several ways. For example, DL may process parameters of unit cells with a fixed geometry. At the same time, free-form design can be implemented, such as unit cells are represented as sets of pixelated images. DL-supplemented design of metasurfaces enable achieving of a great variety of devices, such as multi-resonant and broadband absorbers, metalenses with dual independent focal points, switchable reflectors, and structures with desired circular dichroism. (b ) Example of the LIDAR device consisting of two ITO electrodes containing the metastructures filled with the liquid crystal. Voltages applied to electrodes are controlled via FPGA processor. Metasurfaces deflects the transmitted beam at the desired angle via tuning of the refractive index. (c ) Configuration of binocular near-eye display utilizing beam splitters as in-coupling gratings. The optimised grating design is shown in the inset as well as RGB model of the beam-splitter and electric intensity above the grating. Figure reproduced with permission from: (a) refs.59, 60, under the Creative Commons Attribution 4.0 International License, refs.84, 108, Optica Publishing Group, ref.95, IEEE; (b) ref.109, under a Creative Commons Attribution 4.0 International license; (c) ref.110, Optica Publishing Group.
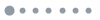
Fig. 5. DL-empowered metasensors. (a ) Schematic of sensing applications of metasurfaces empowered by the of DL methods. DL serves as a tool for designing metasurfaces used as sensing platforms or for analysing measured response spectra and its classification for the presence of specific molecules, etc. (b ) Example of a colorimetric sensor inversely designed with the DL approach. Design procedure implies achieving of a target dual-resonant spectra for a double-bar unit cell. As a result, the sensor is capable to distinguish refractive indexes differed in values by less than 0.01. (c ) DL-supported classification of SARS-CoV-2. Here, unit cell and Raman shift spectra are shown, where P.A. stands for the primary aptamer and S.A. is for the secondary aptamer. Confusion matrix is composed for DL-based classification of clinical samples. (d ) Example of a plasmonic sensor for monitoring biomolecule dynamics. Real-time analysis of absorbance spectra via ML methods allows to distinguish between dynamically changing biological samples. In particular, regression signal allows tracking dynamics of liposome nanoparticles loaded with sucrose and nucleotides. Introduction of melittin results in perforation of lipid membranes and cargo release followed by formation of lipid bilayer. This process can be tracked with the help of ML. Figure reproduced with permission from: (b) ref.138, under a Creative Commons Attribution 4.0 InternationalLicense; (c) ref.142, the authors; (d) ref.143, under a Creative Commons Attribution-NonCommercial-NoDerivatives 4.0 International License.
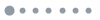
Fig. 6. Self-adapting metasurfaces. (a ) General schematic of DL-assisted self-adaptive metadevices. Programmable metasurfaces are controlled via a DL algorithm processing incoming signal. A change of environment leads to a change of coding sequences required to adjust the response of a metasurface. (b ) Example of a self-adapting cloaking device. An object is covered by a metacloak made of varactor diodes. Data from sensors perceiving changes in background is processed by a DL algorithm determining voltages required to apply to diodes to adjust the cloak. (c ) DL-assisted microwave imager based on a metasurface. Microwave data coming to a metasurface is processed via DL algorithm to reconstruct image of a human. Another DL algorithm can be used for recognition of specific regions within the reconstructed image, such as hands (gestures). Additionally, the collected data may be used for identification of human breath. (d ) Metasurface-based optical ANN with re-programmable functions. Digital metasurfaces are used as a physical layer of the network with programming sequences controlled by FPGA processor. The network can dynamically change its functions via re-training procedure. Figure reproduced with permission from: (b) ref.177, Springer Nature; (c) ref.180, (d) ref.182, under a Creative Commons Attribution 4.0 International License.
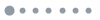
Fig. 7. Examples of DL-empowered metasystems. (a ) Forward and inverse design procedures for topological properties of 1D photonic crystal. Labels 0 and 1 indicate geometric Zak phase of bands (which is either 0 or π, correspondingly). (Adapted from refs.193, 197). (b ) Bound states in the continuum designed via DL algorithm, which allowed to predict reflection spectra with automatically labelled modes and find suitable geometric parameters of a unit cell. The results of the design procedure were confirmed experimentally by angle-resolved measurements. (Adapted from ref.202). (c ) Example of biology-inspired system incorporating DL algorithms. Here, spider-eye-like system is presented, where antenna is used to perceive incoming waves and process them to retrieve information about the environment. ANN in this case explicitly imitates the work of a biological neural network, realizing a system similar to a vision system of a spider. (Adapted from ref.206) Figure reproduced with permission from: (a) ref.193, Optica Publishing Group, ref.197, American Physical Society; (b) ref.202, the authors; (c) ref.206, under a Creative Commons Attribution 4.0 International License.
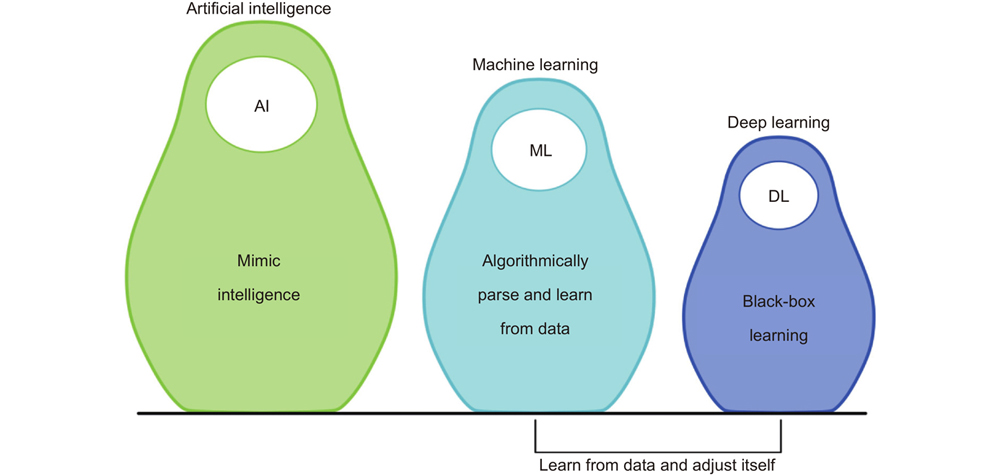
Set citation alerts for the article
Please enter your email address