WANG Ya-sheng, YANG Meng, LUO Zhi-yuan, WANG You, LI Guang, HU Rui-fen. Rapid Coal Classification Based on Confidence Machine and Near Infrared Spectroscopy[J]. Spectroscopy and Spectral Analysis, 2016, 36(6): 1685

Search by keywords or author
- Spectroscopy and Spectral Analysis
- Vol. 36, Issue 6, 1685 (2016)
Abstract
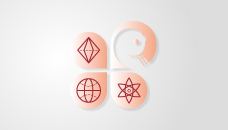
Set citation alerts for the article
Please enter your email address