Umut Hasan, Mamat Sawut, Nijat Kasim, Nigela Taxipulati, WANG Jing-zhe, Irxat Ablat. Hyperspectral Estimation Model of Leaf Water Content in Spring Wheat Based on Grey Relational Analysis[J]. Spectroscopy and Spectral Analysis, 2018, 38(12): 3905

Search by keywords or author
- Spectroscopy and Spectral Analysis
- Vol. 38, Issue 12, 3905 (2018)
Abstract
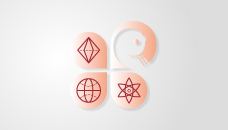
Set citation alerts for the article
Please enter your email address