XU Tiantian, HAN Guangjie, ZOU Yan, ZHU Hongbo, WANG Min, LIN Chuan. Electromagnetic Power Spectrum Density prediction model based on hybrid machine learning[J]. Journal of Terahertz Science and Electronic Information Technology , 2021, 19(4): 623

Search by keywords or author
Journals >Journal of Terahertz Science and Electronic Information Technology >Volume 19 >Issue 4 >Page 623 > Article
- Journal of Terahertz Science and Electronic Information Technology
- Vol. 19, Issue 4, 623 (2021)
Abstract
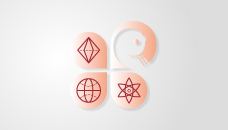
Set citation alerts for the article
Please enter your email address