De-ming KONG, Hong-jie CHEN, Xiao-yu CHEN, Rui DONG, Shu-tao WANG. Research on Oil Identification Method Based on Three-Dimensional Fluorescence Spectroscopy Combined With Sparse Principal Component Analysis and Support Vector Machine[J]. Spectroscopy and Spectral Analysis, 2021, 41(11): 3474

Search by keywords or author
- Spectroscopy and Spectral Analysis
- Vol. 41, Issue 11, 3474 (2021)
Abstract
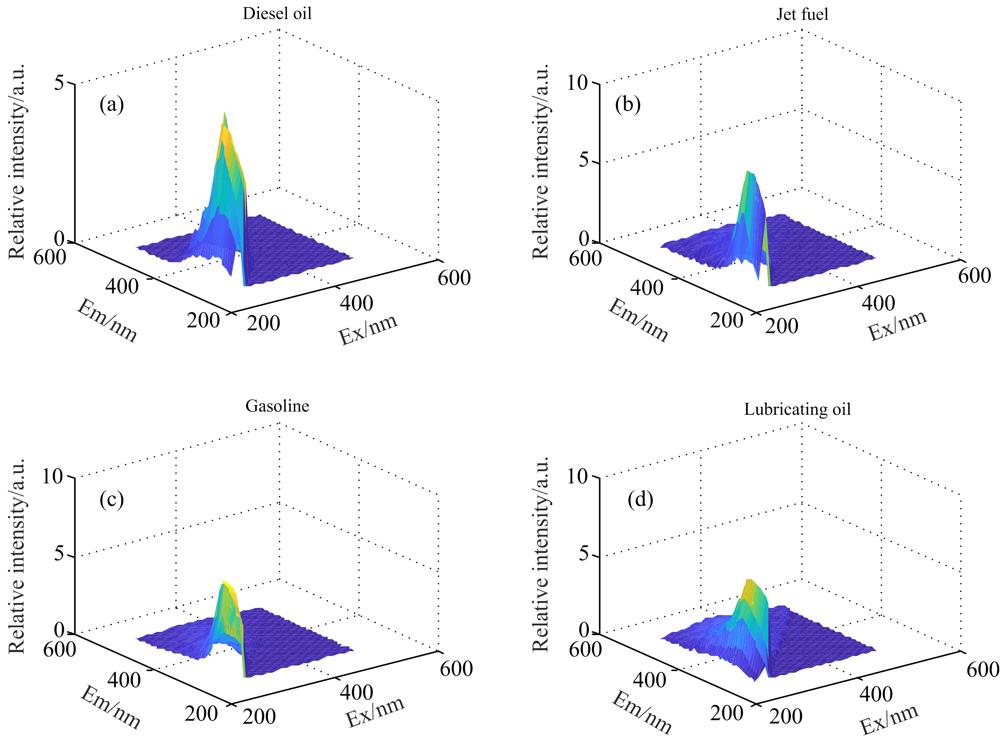
Set citation alerts for the article
Please enter your email address