Abstract
The features of the characteristic matrix used in linear intensity correlation reconstruction methods are directly related to the quality of ghost imaging. In order to suppress the noise caused by the off-diagonal elements in the characteristic matrix, we propose a reconstruction method for ghost imaging called scalar-matrix-structured ghost imaging (SMGI). The characteristic matrix is made to approximate a scalar matrix by modifying the measurement matrix. Experimental results show that SMGI improves the peak signal-to-noise ratio of the object reconstruction significantly compared with differential ghost imaging, even in the case of a nonzero two-arm longitudinal difference, which is a promising result for practical applications.of China (2013AA122901); National Natural Science Foundation of China (NSFC) (61571427); Youth Innovation Promotion Association of the Chinese Academy of Sciences (2013162).1. INTRODUCTION
Ghost imaging (GI), which is to a great extent different from conventional imaging methods, separates the detection and imaging processes. This feature has a unique advantage for imaging applications in complex environments, and therefore has promising prospects in varied applications. The GI technique initially exploited the quantum characteristics of light by involving spatially entangled photon pairs [1–3]. Subsequently, it was realized using pseudo-thermal light sources [4] and thermal light sources [5], significant progress from pure theory to practical applications.
Research in recent years has focused on fields such as biomedical imaging, optical security, ladar detection, 3D imaging, moving target detection, and so on [6–14]. However, for conventional pseudo-thermal light GI, limitations in visibility and resolution remain significant obstacles in many measurements. Many reconstruction methods have been developed to improve GI quality with fewer measurements, as in differential GI (DGI) [15,16], normalized GI [17], the th-order intensity correlation method [18], and compressive GI (CGI) [19,20]. Compared with the former three reconstruction methods, the reconstruction quality of CGI can be significantly enhanced using the same number of measurements, but it may rely on the prior character of the object, and the optimization procedure itself is time-consuming. Recently, from the viewpoint of matrix analysis, the reconstruction results obtained by the intensity correlation method can be predicted by some parameters extracted from a characteristic matrix and are robust to noise, which shows that GI quality can be improved by optimizing the random speckle patterns illuminating the object [21]. The results presented in Refs. [22,23] can also be explained in terms of the characteristic matrix. Some random speckle patterns, which are generated by a rotating ground glass disk and obey a negative exponential statistical distribution, are widely used in remote sensing because of its high damage threshold. However, the imaging quality is relatively mediocre when based on the analysis of the characteristic matrix [9,24]. It is natural to ask whether GI quality can be enhanced by adjusting the characteristic matrix in the image reconstruction process. Instead of optimizing the speckle pattern, pseudo-inverse GI, in which the characteristic matrix becomes a diagonal matrix, can obviously enhance both image visibility and the spatial transverse resolution when the pseudo-inverse method is used to optimize the property of the characteristic matrix [24,25]. Unfortunately, this approach lacks robustness in practical applications. It is therefore highly desirable to develop a robust and universal linear-matrix-based method for fast imaging. This study extends the matrix analysis and reports a matrix-based method named “scalar-matrix-structured ghost imaging” (SMGI) that involves making adjustments to the measurement matrix. Compared with DGI, SMGI can significantly improve the imaging signal-to-noise ratio. The influence of the two-arm longitudinal difference on SMGI and DGI is also discussed.
2. EXPERIMENTAL SETUP AND IMAGE RECONSTRUCTION
The experimental system is outlined in Fig. 1. A pseudo-thermal light source is produced by a laser beam (532 nm wavelength), expanded by lenses and , passing through a rotating ground glass to create a constantly changing speckle pattern. An aperture is used to adjust the speckle size. The laser beam is then split using a 50:50 beam splitter into two identical beams: a transmission beam for the “object” arm and a reflection beam for the “reference” arm. The transmission beam is modulated by the object with a transmission coefficient , and its total light intensity is collected by a bucket detector after passing through lens . The th measurement is recorded as . The reflection beam is detected with spatial intensity distributions by a charge-coupled device (CCD) (Stingray F-504, AVT, Germany), and the th measurement is recorded as . In conventional GI, the transmission coefficient is computed in terms of the correlation between and [19,25]: where and . By reconfiguring the elements of each speckle pattern acquired by the CCD (dimensions ) in the reference arm into a row vector of length to form one row of the matrix , we obtain the following matrix, based on measurements:
Sign up for Photonics Research TOC. Get the latest issue of Photonics Research delivered right to you!Sign up now
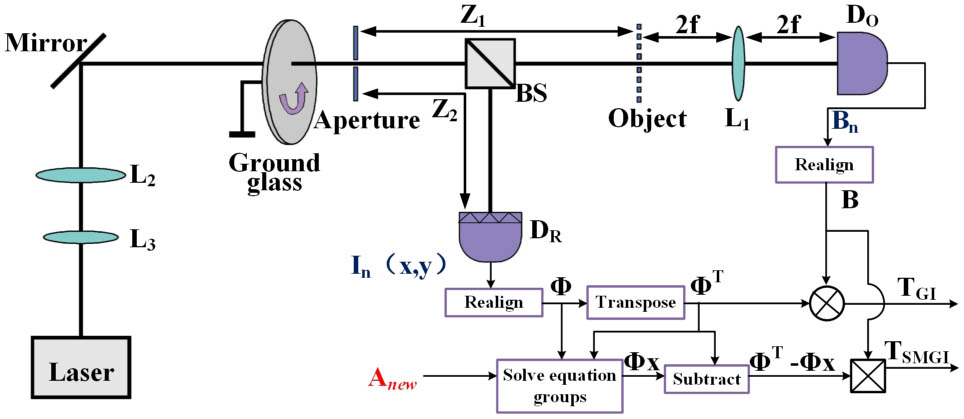
Figure 1.Experimental schematic of the GI and SMGI systems with a pseudo-thermal light source. In the “object” arm, is the distance between the aperture and the object. In the “reference” arm, is the distance between the aperture and the reference CCD. The distance is the focal length of lens .
Likewise, the results from the bucket detector can be permutated into an column vector :
Similarly, the transmission coefficient of the object can be permutated as a column vector :
Equation (1) can thus be expressed in matrix form as where , , and , which corresponds to a constant. In addition, is an column vector whose elements are all 1. , representing the average of each column of , is a row vector. And measurement matrix is the transpose of . We therefore denote as the characteristic matrix. In theory, the closer is to being a scalar matrix (i.e., a matrix whose diagonal elements are nonzero constants and all off-diagonal elements are zero), the closer the image recovery by Eq. (5) is to the original object.
However, , being composed of speckle patterns acquired from practical experiments, cannot yield a perfect scalar characteristic matrix, as the resulting off-diagonal elements constitute the main source of noise in the reconstructed image. We therefore propose a new approach to SMGI involving the construction of a scalar matrix. This method first assumes the existence of an unknown matrix that can be used to amend , yielding a scalar matrix as follows: where is a strict scalar matrix. From Eq. (5), the SMGI reconstruction method can be expressed as
Acquiring is a key requirement. From Eq. (6), it can be obtained by matrix division:
The degree to which the characteristic matrix approaches a scalar matrix determines the reconstruction effects. is closer than to being a scalar matrix, and therefore the reconstructed image in SMGI is closer to the original object. An exact solution of would, in theory, yield a distortion-free reconstruction of the object.
3. EXPERIMENTAL RESULTS
To perform SMGI experimentally, we set up the system illustrated in Fig. 1, where , , the distance between and lens is 300 mm, and the pixel size of the CCD camera is approximately . is in fact an identical CCD to . The total intensity can be acquired by summing the entire intensity distribution, thereby effectively functioning as a bucket detector. We adjusted the aperture to acquire a proper speckle size. As a preliminary verification of the feasibility of our method, we first constructed and obtained , and then computed using Eq. (8) by matrix division. Results for and are shown in Fig. 2. Comparing Figs. 2(a) and 2(b), we see that the values of the off-diagonal elements in are significantly greater than those in , which indicates that is closer to being a scalar matrix and that the noise caused by its off-diagonal elements is less significant during reconstruction. These results constitute a preliminary proof of principle for our approach.
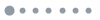
Figure 2.Calculated results for (a) and (b) . The axis represents the row coordinate of the matrices, and the axis represents the column coordinate.
For the experiments, we chose a transmission aperture of pixels, displaying the letters “GI” as the target object. We compared the reconstructions by SMGI, DGI, and GI after different measurement times. We quantified the performance in each case in terms of the peak signal-to-noise ratio (PSNR) to illustrate the reconstruction fidelity. The reconstructions and their PSNR curves are shown in Figs. 3(a) and 3(b), respectively.
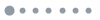
Figure 3.Comparisons of experimental results obtained by GI, DGI, and SMGI for object “GI” for a varying number of measurements. (a) Reconstruction results corresponding to 500, 1000, and 2000 measurements, (b) PSNR for the GI (black), DGI (blue), and SMGI (red) reconstructions, plotted as a function of the number of measurements (100–2000).
Figure 3(a) shows the enhancement of all the visual effects of the reconstructions with increasing measurement time. For a given number of measurements, GI performs poorly and yields very vague images, whereas the results for DGI are relatively improved. However, SMGI makes further progress in resolution. Further observations confirmed that the reconstruction of SMGI based on 500 measurements is as effective as DGI based on 2000 measurements, and significantly outperforms GI after 2000 measurements.
Considering the quantitative results of Fig. 3(b), the PSNR for all three methods increases monotonically with the number of measurements. Compared with DGI and GI, SMGI shows higher PSNR values for a given number of measurements. The PSNR for SMGI exceeds that for DGI by as much as 3.8 dB (300 measurements). In the case of only 500 measurements, the PNSR for SMGI reaches approximately 13.9 dB, i.e., 1.1 dB higher than for DGI and 2.9 dB higher than for GI. These quantitative results are consistent with the visual impression, and further confirm the feasibility of our approach, highlighting its clear advantages for improving the quality of reconstructions.
In our experiments, each row of [Eq. (2)] corresponds to a row-by-row reconfiguration of the speckle pattern. A speckle typically has a certain size, extending over several CCD pixels, that produces correlations between some adjacent elements and elements separated by intervals of length (corresponding to adjacent elements in the column of the speckle pattern) in each row of matrix . Thus, consists of multiple parallel peaks with a certain width separated by , with the peak width and quantity related to the speckle size. The part containing the diagonal peaks of Fig. 2(a) is shown in Fig. 4. clearly displays a series of peaks of identical width, oriented diagonally. Therefore, the diagonal region of can break down into multiple approximate diagonal matrices, indicated with the yellow dashed boxes in Fig. 4(b). The element values of the off-diagonal part in each matrix are visibly large and fluctuate significantly.
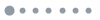
Figure 4.Calculated results for . (a) Part of the diagonal region of . (b) 2D grayscale image corresponding to (a). (c) Values of the matrix elements along the red dashed arrow lines in (a) and (b). The horizontal axis represents the sequence number of elements along the red dashed arrow line.
is constructed using . Considering the multiple-peak structure of , when building matrix , instead of making it a strict scalar matrix, it is better to reserve all the peaks in with a certain width and set other elements to zero. This is the basic principle underlying the construction of . is thus composed of many small matrices that are very close to being scalar matrices. Further, based on Eq. (8), we can calculate and obtain , as shown in Fig. 2(b). The region corresponding to Fig. 4 in Fig. 2(b) is shown in Fig. 5. The comparison of Figs. 4 and 5 suggests that it not only retains all the peaks corresponding to but also makes the values of elements other than the peak region fluctuate smoothly and close to zero in . In the same way, the diagonal region of is actually composed of a number of diagonal matrices ( pixels), which are much closer to the strict scalar matrices compared with .
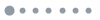
Figure 5.Calculated results for . (a) Part of the diagonal region of ; (b) 2D gray-scale image corresponding to (a); (c) Values of the matrix elements along the red dashed arrow lines in (a) and (b). The horizontal axis represents the sequence number of the elements along the red dashed arrow line.
Accuracy is an important criterion for assessing the practicability of a reconstruction method. All the above experiments were conducted with the same object arm and reference arm lengths (), so that the speckle patterns on the object plane could be acquired accurately by the CCD in the reference arm, which requires harsh experimental conditions and increases the difficulty of the experiment. Therefore, in order to further analyze the practicality of the SMGI method, we used the above “GI” image and another image (representing the Chinese character “zhong” surrounded by a partial ring) with the same number of pixels as the objects. We performed contrast experiments by SMGI and DGI with a two-arm longitudinal difference . In the results shown in Fig. 6, the speckle transverse size is approximately in the “GI” object, and in the “zhong” object.
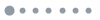
Figure 6.Comparison of SMGI and DGI with 2000 measurements under two-arm longitudinal deviation (μm) with fixed and variable . (a) Reconstructions of the object “GI”; (b) Reconstructions of “zhong”; (c) PSNR curves corresponding to (a); (d) PSNR curves corresponding to (b).
Figure 6 shows that the reconstructions for both methods are clearest when the two-arm longitudinal difference , but the results of SMGI are even better than those of DGI. As the longitudinal difference increases, the image quality obtained by both methods gradually declines. However, SMGI still yields better visual effects and quantitative results for a given . Further observation shows that SMGI performs even better when or 1250 μm than does DGI with . In the case of greater (i.e., 2000 or 2500 μm), SMGI still produces clear results with a PSNR close to or even better than DGI with . The dependence of the speckle-pattern accuracy in our method is not high, even lower than in DGI. It can therefore be concluded that our method is robust, easy to implement, and practicable.
4. CONCLUSION
In conclusion, we propose a new (to our knowledge) reconstruction method for GI, SMGI, and explain how it amends the characteristic matrix to yield a better approximation to a scalar matrix. Experimental results show that as compared with another reconstruction method, such as DGI, this method can effectively enhance both the PSNR and the visual impression of the reconstructed image, even with a two-arm longitudinal difference. This outcome shows much promise for future practical applications.
References
[1] T. B. Pittman, Y. H. Shih, D. V. Strekalov, A. V. Sergienko. Optical imaging by means of two-photon quantum entanglement. Phys. Rev. A, 52, R3429-R3432(1995).
[2] D. V. Strekalov, A. V. Sergienko, D. N. Klyshko, Y. H. Shih. Observation of two-photon” ghost” interference and diffraction. Phys. Rev. Lett., 74, 3600-3603(1995).
[3] R. E. Meyers, K. S. Deacon. Quantum ghost imaging experiments at ARL. Proc. SPIE, 7815, 78150I(2010).
[4] R. S. Bennink, S. J. Bentley, R. W. Boyd. Two-photon coincidence imaging with a classical source. Phys. Rev. Lett., 89, 113601(2002).
[5] D. Zhang, Y. H. Zhai, L. A. Wu, X. H. Chen. Correlated two-photon imaging with true thermal light. Opt. Lett., 30, 2354-2356(2005).
[6] W. Gong, S. Han. Correlated imaging in scattering media. Opt. Lett., 36, 394-396(2011).
[7] N. Tian, Q. Guo, A. Wang, D. Xu, L. Fu. Fluorescence ghost imaging with pseudothermal light. Opt. Lett., 36, 3302-3304(2011).
[8] W. Chen, X. Chen. Marked ghost imaging. Appl. Phys. Lett., 104, 251109(2014).
[9] C. Zhao, W. Gong, M. Chen, E. Li, H. Wang, W. Xu, S. Han. Three-dimensional ghost imaging ladar via sparsity constraint. Appl. Phys. Lett., 101, 141123(2012).
[10] B. Sun, M. P. Edgar, R. Bowman, L. E. Vittert, S. Welsh, A. Bowman, M. J. Padgett. 3D computational imaging with single-pixel detectors. Science, 340, 844-847(2013).
[11] W. Gong, C. Zhao, H. Yu, M. Chen, W. Xu, S. Han. Three-dimensional ghost imaging lidar via sparsity constraint. Sci. Rep., 6, 26133(2016).
[12] X. Li, C. Deng, M. Chen, W. Gong, S. Han. Ghost imaging for an axially moving target with an unknown constant speed. Photon. Res., 3, 153-157(2015).
[13] Z. Li, J. Suo, X. Hu, Q. Dai. Content-adaptive ghost imaging of dynamic scenes. Opt. Express, 24, 7328-7336(2016).
[14] P. Ryczkowski, M. Barbier, A. T. Friberg, J. M. Dudley, G. Genty. Ghost imaging in the time domain. Nat. Photonics, 10, 167-170(2016).
[15] W. Gong, S. Han. A method to improve the visibility of ghost images obtained by thermal light. Phys. Lett. A, 374, 1005-1008(2010).
[16] F. Ferri, D. Magatti, L. A. Lugiato, A. Gatti. Differential ghost imaging. Phys. Rev. Lett., 104, 253603(2010).
[17] B. Sun, S. S. Welsh, M. P. Edgar, J. H. Shapiro, M. J. Padgett. Normalized ghost imaging. Opt. Express, 20, 16892-16901(2012).
[18] D. Cao, J. Xiong, S. Zhang, L. Lin, L. Gao, K. Wang. Enhancing visibility and resolution in Nth-order intensity correlation of thermal light. Appl. Phys. Lett., 92, 201102(2008).
[19] O. Katz, Y. Bromberg, Y. Silberberg. Compressive ghost imaging. Appl. Phys. Lett., 95, 131110(2009).
[20] W. Gong, S. Han. High-resolution far-field ghost imaging via sparsity constraint. Sci. Rep., 5, 9280(2015).
[21] C. Wang, W. Gong, X. Shao, S. Han. The influence of the property of random coded patterns on fluctuation-correlation ghost imaging. J. Opt., 18, 065703(2016).
[22] S. M. M. Khamoushi, Y. Nosrati, S. H. Tavassoli. Sinusoidal ghost imaging. Opt. Lett., 40, 3452-3455(2015).
[23] H. Ghanbari-Ghalehjoughi, S. Ahmadi-Kandjani, M. Eslami. High quality computational ghost imaging using multi-fluorescent screen. J. Opt. Soc. Am. A, 32, 323-328(2015).
[24] C. Zhang, S. Guo, J. Cao, J. Guan, F. Gao. Object reconstitution using pseudo-inverse for ghost imaging. Opt. Express, 22, 30063-30073(2014).
[25] W. Gong. High-resolution pseudo-inverse ghost imaging. Photon. Res., 3, 234-237(2015).