Yu-ting XU, Hao-ran SUN, Xun GAO, Kai-min GUO, Jing-quan LIN. Identification of Pork Parts Based on LIBS Technology Combined With PCA-SVM Machine Learning[J]. Spectroscopy and Spectral Analysis, 2021, 41(11): 3572

Search by keywords or author
- Spectroscopy and Spectral Analysis
- Vol. 41, Issue 11, 3572 (2021)
Abstract
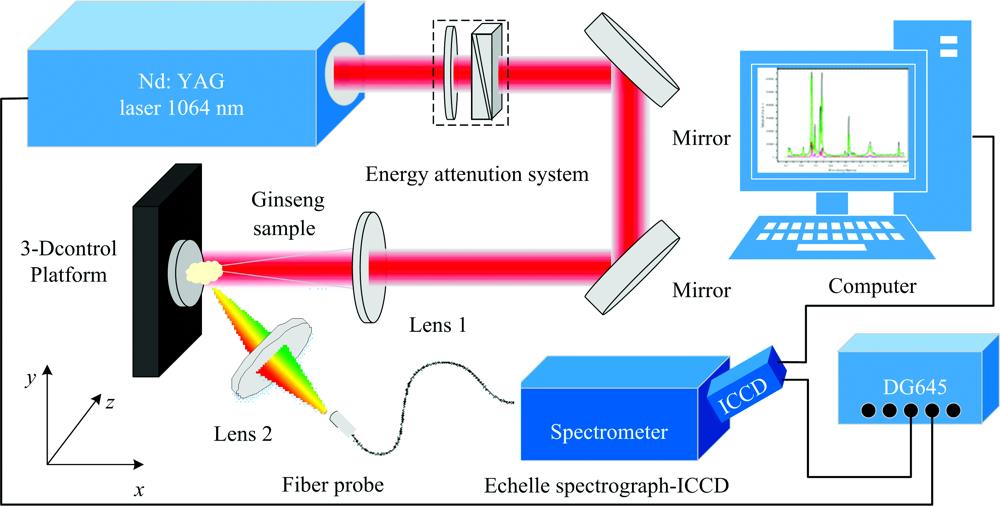
Set citation alerts for the article
Please enter your email address