SHAO Yan-hao, ZHU Rong-gang, HE Jian-liang, KONG Fan-e. Evasive Decision-Making in Inescapable Areas Based on Deep Learning[J]. Electronics Optics & Control, 2019, 26(11): 60

Search by keywords or author
- Electronics Optics & Control
- Vol. 26, Issue 11, 60 (2019)
Abstract
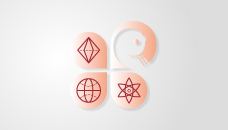
Set citation alerts for the article
Please enter your email address