YU Hailong, GAO Yujin, XIE Yunshuang, YANG Shuo, TANG Yuxuan, GAO Xun, LIN Jingquan. The effect of spectral preprocessing wavelet transform basis functions selection on the classification accuracy of aluminum alloys combining FIBS and machine learning[J]. Laser Journal, 2024, 45(6): 88

Search by keywords or author
- Laser Journal
- Vol. 45, Issue 6, 88 (2024)
Abstract
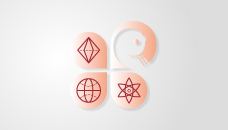
Set citation alerts for the article
Please enter your email address