Ying XIA, Junyao LI, Dongen GUO. Semi-supervised Scene Classification of Remote Sensing Images Based on GAN[J]. Acta Photonica Sinica, 2022, 51(3): 0310003

Search by keywords or author
- Acta Photonica Sinica
- Vol. 51, Issue 3, 0310003 (2022)
Abstract
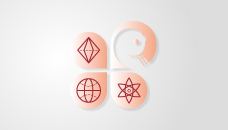
Set citation alerts for the article
Please enter your email address