
- Journal of Resources and Ecology
- Vol. 11, Issue 4, 394 (2020)
Abstract
1 Introduction
As a clean, safe, highly-efficient and economical form of energy, nuclear power is the only non-fossil fuel energy in the world which can completely replace the energy from fossil fuels to meet the needs of large-scale industrial development (
During decades of rapid economic development, China’s growing demand for electricity has been supported mainly by increasing the use of high-carbon fossil fuel energy (with coal accounting for approximately 75 percent of China’s energy). The country is now under great pressure to reduce greenhouse gas emissions (
If the total installed capacity of nuclear power reaches 150-200 million kW in 2030, then the total installed capacity of nuclear power is expected to reach 300-400 million kW in 2050. Without the introduction of fast reactors and MOX fuel, an estimated 27-36 thousand tons of natural uranium will be needed in 2030, and 54-72 thousand tons will be needed in 2050, which is obviously a considerable demand (
However, the access of commercial fast reactors to the nuclear power market depends on many factors, including the maturity of technology, economy, supply of uranium resources, etc., and different stakeholders have different priorities. Some nuclear fuel cycle schemes may be more advantageous than other schemes, but not all of the decision makers will be satisfied with them. Therefore, the establishment of evaluation indicators, methods and evaluation models is of great significance for the sustainable development of uranium resources in China (
2 Data sources and research methods
The nuclear fuel cycle includes the front end, the irradiation stage and the back end. The front end includes uranium mining, ore processing, uranium extraction, conversion, enrichment, fuel manufacturing, etc., while the irradiation stage is the reactor stage, and the back end includes the temporary storage, cooling, packaging, transportation, geological disposal and recovery of spent fuel, etc. If the recycling plan is selected correctly, it will not only improve the utilization rate of uranium resources, but will also greatly reduce the generation and toxicity of radioactive waste, and at the same time, it will alleviate the contradiction between the supply and demand of uranium resources.
Underlying the primary context and constraints specific to China is the crucial need to draft a framework for making informed decisions regarding the initiation of a sustainable development roadmap for uranium resources. Such a framework should (a) be capable of using an integrated system for evaluation and obtaining information about the potential benefits and costs of the potential fuel cycle options, (b) find a balance of benefits, (c) make appropriate decisions to achieve stability, and (d) be capable of providing policy guidance for planning nuclear energy in China.
Here we have formulated evaluation indicator metrics for China-specific sustainability associated with five reference scenarios of fuel cycle transition options. We then established a GAN analysis framework that is connected with the quantitative performance of the five candidate options for the fuel cycle. We evaluated and ranked each of these five fuel cycle options to model and evaluate the sustainability of China-specific uranium resources. We also further analyzed the roles of different representatives of stakeholders and their impacts on the overall ranking of the options from the perspective of weight, and found a balance point among the corresponding decisions to maintain stability.
The choice of nuclear fuel cycle plays an important role in the economy of uranium mining. Therefore, this paper sets five scenarios according to the different options for recycling uranium mine resources, and evaluates the influences of various index factors on the sustainable development of uranium mine resources through the five scenarios.
Scenario 1: Pressurized Heavy Water Reactors (PHWR) with direct disposal of spent nuclear fuel.
Scenario 2: Mixed Light Water Reactor (LWR) + Fast Reactor (FR) fuel cycle scheme with homogeneous multiple recycling of transuranic fuel.
Scenario 3: Mixed LWR+FR fuel cycle scheme with heterogeneous irradiation of Minor Actinide targets.
Scenario 4: Mixed Pressurized Water Reactor (PWR) + FR fuel cycle scheme with plutonium recycled directly and repeatedly in a closed fuel cycle.
Scenario 5: Sodium-cooled fast breeder reactor power plant with the resulting plutonium repeatedly recycled as Mixed Uranium-Plutonium Oxide (MOX) fuels.
Based on the sustainability of uranium in the future, we have classified and determined eight key evaluation indicators including uranium supply, demand, price, technological readiness, environmental impact, strategy, political impact and management (
There are always diverse stakeholders involved in the process of evaluating uranium resources. They each put forward individual preferences and opinions on the nuclear energy strategy which weigh the trade-offs and opinions within the strategic framework of decision-making in order to achieve a mutual compromise on the sustainable nuclear fuel cycle system in China. Considering the values (quantity) and preferences (quality) from diverse stakeholders, we further analyzed the role of different stakeholder representatives and their impacts on the overall ranking from the perspective of weight.
Based on extensive literature review and extensive consultation with experts, we used traditional questionnaire survey methods to provide stakeholders with a series of questionnaires. We conducted a weighting assumption analysis of the stakeholders to illustrate how the ranking differs according to their distinctive socio-political perspectives (
3 Establishment of the safety evaluation index of uranium resources in China
The core issue of the traditional definition of energy security is ensuring energy supply. As a non-renewable resource, the uranium mine determines that its mining is closely related to the resource environment. Traditional energy security ignores environmental protection and maintains ecological balance. Therefore, this paper adds environmental factors to the safety evaluation index system; that is, the safety evaluation of uranium resource sustainable development includes two aspects: uranium resource supply security and use safety. Supply security refers to the stability, economy and sustainability of the uranium resource supply (
3.1 Data reliability and validity analysis
The security of the uranium resource supply is a matter of politics, military, economy, resources, man-made and many other factors. Its safety evaluation is also a complex system problem, and it is necessary to build a comprehensive and perfect indicator system that reflects its safety status (
Key indicators | Sub-indicators | Cronbach α |
---|---|---|
Supply indicator | Resources (expected reserves, recovered reserves, recoverable reserves) ( | 0.802 |
Production volume (yield growth rate, storage-production ratio) ( | ||
Imported uranium mine (import concentration, import share, external dependence) ( | ||
Demand indicator | Population growth ( | 0.814 |
Lifestyle of residents ( | ||
Economic growth rate ( | ||
Technological advancement ( | ||
Production and consumption structure ( | ||
Alternative levels of other energy sources ( | ||
Supply and demand ratio of uranium resources ( | ||
Uranium resource consumption intensity ( | ||
Industrial structure ( | ||
Price indicator | Producer price ( | 0.898 |
International market price ( | ||
Production cost ( | ||
Marginal cost of mining technology ( | ||
Tax rate ( | ||
Technical indicator | Mining rate ( | 0.858 |
Uranium comprehensive utilization rate ( | ||
Science and technology contribution rate of uranium mining industry ( | ||
Scientific and technological achievements conversion rate of uranium mining industry ( | ||
Environment indicator | Nuclear waste (spent fuel post-processing) stock ( | 0.856 |
Uranium mine regional distribution ( | ||
Welfare loss ( | ||
Uranium mine depletion cost ( | ||
Environmental degradation cost ( | ||
Environmental pollution loss ( | ||
Strategic indicator | Control of domestic uranium mines ( | 0.842 |
Control of international uranium mines ( | ||
Strategic reserve for uranium mines ( | ||
Global development strategy ( | ||
Political indicator | External relationship stability ( | 0.752 |
Domestic political environment stability ( | ||
Uranium mining industry policy ( | ||
Consumption habits of nuclear power ( | ||
Management indicator | Human resources ( | 0.791 |
Equipment integrity rate ( | ||
Improvement rate of production safety system measures ( | ||
Safety of import transportation channel ( | ||
Influence control degree of import transportation channels ( | ||
Environmental safety ( | ||
Information identification and processing capabilities ( |
Table 1.
Confidence analysis of the overall indicator metrics for evaluating the resource safety of uranium in China
By calculating the Cronbach α value of the evaluation reliability for each latent variable, the table above shows that they are all greater than 0.750, indicating that the measurement has a high reliability level for each variable.
In terms of validity, firstly, since each variable measurement is derived from the relevant results and opinions of domestic scholars, the scale can be considered to have good content validity. Secondly, the KMO sample measurement and the Bartlett Test of Sphericity were used to determine whether the sample is suitable for factor analysis. The results of this analysis are shown in
Method | Variables | Value | |
---|---|---|---|
Kaiser-Meyer-Olkin | KMO value | 0.954 | |
Bartlett’s sphericity test | Approximate chi square | 2136.125 | |
df | 162 | ||
0.000 |
Table 2.
Factor analysis fitness test using KMO value and Bartlett’s spherical test
Using MATLAB software for exploratory factor analysis gives a KMO value of 0.954, which is very suitable for factor analysis; and the Bartlett hemispherical test value is 0.000, which is less than 0.001, supporting the exploratory factor analysis, and indicating that the data has good validity.
3.2 Path analysis of fitness between the index system and observation data
Given the above results, the following strategic indicators were used to measure the fit between the indicator system and the observation data: Chi-square value, significance value, adjusted goodness-of-fit index, goodness-of-fit index, root mean square error of approximation and CN value. The fitness of the initial model is shown in
Chi-square value | AGFI | GFI | RMSEA | CN | |
---|---|---|---|---|---|
197.740 | 0.000 | 0.846 | 0.886 | 0.175 | 187 |
Table 3.
Model fitness index
In the initial hypothesis model fitting indexes, AGFI<0.9, GFI<0.9, RMSEA<0.5, CN<200, and P=0.000<0.05, reach the level of significance, thereby rejecting the null hypothesis and assuming that the model does not fit the observation data. Thus, the model needs to be further revised. By referring to the correction index MI provided by AMOS, the covariation relationship between the error variables is increased. Following the correction, the adaptation degree of the model is shown in
Chi-square value | AGFI | GFI | RMSEA | CN | |
---|---|---|---|---|---|
138.682 | 0.076 | 0.832 | 0.938 | 0.029 | 194 |
Table 4.
Revised model fitness index
4 Construction of the evaluation index model for sustainable development of uranium resources in China
Analytic Hierarchy Process (AHP) is a system decision-making method that breaks down a problem into several levels of simpler problems which are then represented by a set of criteria or attributes. Relative to each sub-question, the AHP method is always applied to determine the relative weights of the evaluation indicators (coefficients), and the Decision Support System (DSS) is combined with the individual's point of view to obtain an overall relative weight option (priority) (
Since the adversarial neural network model of the sine function has the statistical characteristics of memory recovery, we first construct an adversarial neural network model with generalized input and output functions:
Among them, x and y respectively represent the neuron output values of X and Y in the sub-indicators in
Key | Sub-indicators | Technical department | Economic department | Social | Environmental department |
---|---|---|---|---|---|
Supply | Resources (expected reserves, recovered reserves, recoverable reserves) ( | 0.225 | 0.337 | 0.013 | 0.004 |
Production volume (yield growth rate, storage-production ratio) ( | |||||
Imported uranium mine (import concentration, import share, | |||||
Demand | Population growth ( | 0.007 | 0.122 | 0.006 | 0.003 |
Lifestyle of residents ( | |||||
Economic growth rate ( | |||||
Technological advancement ( | |||||
Production and consumption structure ( | |||||
Alternative level of other energy sources ( | |||||
Supply and demand ratio of uranium resources ( | |||||
Uranium resource consumption intensity ( | |||||
Industrial structure ( | |||||
Price | Producer price ( | 0.012 | 0.203 | 0.104 | 0.004 |
International market price ( | |||||
Production cost ( | |||||
Marginal cost of mining technology( | |||||
Tax rate ( | |||||
Technical | Mining rate ( | 0.414 | 0.107 | 0.013 | 0.329 |
Uranium comprehensive utilization rate ( | |||||
Science and technology contribution rate of uranium mining industry ( | |||||
Scientific and technological achievements conversion rate of uranium | |||||
Environmental indicator | Nuclear waste (spent fuel post-processing) stock ( | 0.148 | 0.006 | 0.512 | 0.474 |
Uranium mine regional distribution ( | |||||
Welfare loss ( | |||||
Uranium mine depletion cost ( | |||||
Environmental degradation cost ( | |||||
Environmental pollution loss ( | |||||
Strategic | Control of domestic uranium mines ( | 0.009 | 0.103 | 0.073 | 0.049 |
Control of international uranium mines ( | |||||
Strategic reserve for uranium mines ( | |||||
Global development strategy ( | |||||
Political | External relationship stability ( | 0.012 | 0.015 | 0.062 | 0.078 |
Domestic political environment stability ( | |||||
Uranium mining industry policy ( | |||||
Consumption habits of nuclear power ( | |||||
Management | Human resources ( | 0.173 | 0.107 | 0.217 | 0.059 |
Equipment integrity rate ( | |||||
Improvement rate of production safety system measures ( | |||||
Safety of import transportation channel ( | |||||
Influence control degree of import transportation channels ( | |||||
Environmental safety ( | |||||
Information identification and processing capabilities ( |
Table 5.
Weight values of evaluation indicators from the stakeholders’ perspectives
Suppose h: Rn, since Rn is n-dimensional Euclidean space, the usual infinite norm assigned to Rn can be regarded as an iterative map or a neural network with continuous state,
Then a cycle occurs at$({{x}_{0}},{{\mu }_{0}})$, there is a curve$x(\mu )$in the vicinity of ${{\mu }_{0}}$, $x(\mu )$is a stable fixed point on one side of ${{\mu }_{0}}$, and an unstable fixed point after passing${{\mu }_{0}}$; and there is a smooth curve $\xi $ tangent to the line$\text{ }\!\!\{\!\!\text{ }{{\mu }_{0}}\text{ }\!\!\}\!\!\text{ }$at the point$({{x}_{0}},{{\mu }_{0}})$; and $\xi $ is a mapping iteration of $\mu $to $\theta (x)$ function about x. When$\beta >0$, the cycle is stable, and vice versa.
When the parameters are satisfied with$\rho >0,k>1,-1<$ ${{a}_{0}}-\frac{a}{\rho }<0$, and the parameter$\lambda $ changes, there must be $\lambda ={{\lambda }^{*}}$ in the interval$\left( 0,\frac{\rho }{k+1} \right),$so that the period at $\left( \frac{y(t)}{{{\lambda }^{*}}},{{\lambda }^{*}} \right)$is stable.
Vectorizing the model as:
Among them, $diag\{{{\rho }_{11}},{{\rho }_{22}},...,{{\rho }_{nn}}\}$is the diagonal matrix.
In addition:
$Y(\text{t)}=\left[
If P is a non-singular matrix, then:
Due to the nature of the sine function, when
At this stage, the existence and stability of the unique solution are judged by the input and output functions, which can be obtained from the GAN model:
In addition, μi(t) is the given weight of the i-th indicator. When we aggregate the global preference information considering total indicators, a preference function determines the relevant preference extent by using the ranking relation. However, the antagonistic preferences between two options can be independently presented by different preference functions. The function determines the appropriate graphical representations of the preference function in line with the various types of indicators. So, we derive the antagonism function of μi(t) by calculating
Then a stochastic gradient descent algorithm is used to evaluate the objective function in formula (8) based on the parameters of each tuple
As a result, there is a unique equilibrium solution
5 Evaluation index model of uranium resource safety in China
The above data were calculated by a model of neural network evaluation, which showed that the evaluation index model has good practicability. The evaluation results are in good agreement with the actual evolution of uranium supply security in China. In general, the uranium supply security situation is relatively poor in China, and it is currently being
transformed into a highly dangerous state, which is inconsistent with the process of building a well-off society in all aspects. Uranium resources in China have huge discrepancies in spatial and temporal distribution, the level of economic development varies from place to place, and consumers’ awareness of energy conservation is inconsistent, thus increasing the difficulty of achieving uranium supply safety.
Figure 1.Fig. 1
The results show that scenario 1 is the most unsustainable and highly confrontational scenario, with high demand for uranium resource supply, the least sustainability and high antagonism among the departments. In contrast, scenario 5 requires higher technology but produces less antagonism among departments, which substantially satisfies the basic requirements underlying the current definition of uranium sustainability and low antagonism.
Accordingly, the technology department is more inclined to choose scenario 5, while social residents are more inclined to manage the security because they lack a secure sense of the latest technology, so they will give up scenario 5 and choose scenario 4. The economic department will be more inclined to choose scenario 5 under the condition of appropriate price.
Figure 2.Fig. 2
The factors that have greater impacts on the safety of the uranium supply are mainly resource factors and import factors, as their cumulative weights are higher. Among the individual indicators, the strategic uranium reserve and storage-production ratio are the most important indicators, so they will mainly determine the utilization and benefit of uranium mine in China.
To perform a sensitivity analysis, we build (A) Fraction of precision and (B) Sensitivity performance, and analyze the sensitivity of the preference functions about three variables$\frac{y(t)}{{{\lambda }^{*}}}$, $\Phi \left( \frac{Y(t)}{\lambda } \right)$ and${{\mu }_{i}}(t)$, the x-axis represents the weight of three indicators, the y-axis of A shows the fraction of precision for the five scenarios and the y-axis of B as the sensitivity performance, then adopted a probabilistic approach through a simulation using the four departments. It simulated the iteration of the weight values of an individual extreme preference-oriented indicator in the five scenarios within an assigned uniform probability distribution. Here, the same principle of equal importance of all indicators was likewise applied to ensure that the sum of all the indicator weighting values at the same level equaled ‘1’.
shows the elimination of individual subjective bias for a defined inner range of distribution as 0.7835, with another line showing the outer limits of the distribution as 0.0583. The green line in the
The ultimate goal of the uranium mine index evaluation is not only to determine the current status of the mined uranium supply security in China, but also to determine the leading factors which affect the safety of the mined uranium supply based on the results of the evaluation, and then propose targeted countermeasures to ensure the safety of the mined uranium supply and, ultimately, sustained and healthy economic development in China.
Through the analysis of the status of uranium resource use globally and in China, and the international situation, the main influencing factors of the uranium resource safety system are obtained, safety evaluation indicators are constructed, the current situation of uranium resource utilization is sorted out, and the interests of the state and enterprises are taken into account. At the same time, from the political, economic, cultural, military, social and other perspectives, a multi-perspective approach used fuzzy mathematical analysis to construct China’s uranium resource safety evaluation index model. This model dynamically reflects the supply and demand of China’s uranium resources, the timely control of uranium supply and demand, and the realization of the coordinated development of the uranium resource industry chain.
6 Discussion and policy implications
The uranium resource safety evaluation system can not only ensure the healthy and sustainable development of the national economy, but also achieve harmony and tolerance between people and nature, and enrich and expand the application scope of the index evaluation. The use of grounded theory and more advanced mathematical methods to obtain a safety evaluation index system and a dynamic balance model is a new exploratory approach, and a beneficial attempt for the safe use of uranium resources. The findings revealed by this approach lead to the following policy recommendations:
(1) On the premise of ensuring the sustainable development of uranium resources in China, it is necessary to comprehensively strengthen the exploration and development of domestic fissile nuclear energy mineral resources, and to fully utilize existing production capacity. At the same time, a number of new uranium mines should be constructed and planned so as to achieve and maintain a certain scale of natural uranium production capacity in China.
(2) Promote to enrich the reserve of resources, including the reserves of mineral deposits and uranium products, the total amount of which is the sum of those required for the scale of three years after the development of nuclear power.
(3) Take overall strategic planning and overall coordination, including foreign exploration and development of uranium resources as a priority development area; assist foreign and political parties and military parties in the face of corporate behavior; and establish joint mining groups, financial groups, and others. The industry should be coupled to form an overseas competitive industrial chain with strong competitiveness. It should actively participate in the international mineral resource pricing and construction of financial trading markets, so that China can compete and cooperate with existing monopoly forces in overseas investment and trade.
(4) Promote scientific and technological progress, continuously improve the utilization rate of uranium resources, accelerate the technological upgrading of the mixing and leaching production line, and tackle the research and development of the proven large-scale uranium deposits; pay attention to the research and evaluation of unconventional uranium resources, and pay attention to the research and development of extraction technology for salt-lake uranium resources, as well as the exploration of sea uranium extraction technology; achieve gradual resolution of uranium tailing slag re-extraction and reuse; and focus on research on the environmentally-sound treatment of mine solid wastes.
References
[1] S Álvaro E de. Stakeholders’ manipulation of environmental impact assessment. Environmental Impact Assessment Review, 68, 10-18(2018).
[2] L An J. On basic stages, key areas and security in China’s nuclear energy development and utilization. Journal of University of South China (Social Science Edition), 17, 13-20(2016).
[6] Q Cai Y, H Yu, C Li X et al. Study on the technology of uranium resource prediction and evaluation with big data. Uranium Geology, 35, 321-329(2019).
[12] R Gorman M, A Dzombak D. A review of sustainable mining and resource management: Transitioning from the life cycle of the mine to the life cycle of the mineral. Resources, Conservation & Recycling, 137, 281-291(2018).
[13] Y Gu C. Research on risk evaluation of China’s overseas mining investment based on deep learning, PhD diss.. China University of Geosciences(2019).
[15] Experts discuss design options for future fusion power plant. Vienna: IAEA.(2019).
[16] How can the IAEA help make sure that nuclear energy is sustainable?. Vienna: IAEA.(2019).
[17] IAEA climate conference ends with call for major nuclear role. Vienna: IAEA.(2019).
[18] More newcomers eye nuclear power as UAE, Belarus set to start operating first nuclear power plants.. Vienna: IAEA.(2019).
[19] Spent fuel management: Four decades of research.. Vienna: IAEA.(2019).
[20] Y Li, S Chen Q, Y Liu Q et al. Safety assessment and situation analysis of China’s overseas mineral resources supply. Resource Science, 37, 900-907(2015).
[21] T Liu, F Liu Q. Status of global uranium resources and development trend of nuclear energy. Modern Mining, 125-127(2017).
[23] T Long, S Chen Q, J Yu W et al. Research on the new pattern of global energy supply and demand. China Mining Magazine, 28, 63-66(2019).
[24] M Peng Q. Increase the deliverability of strategic minerals, promote rapid development of emerging industry — Speech on the “analysis and discussion conference of supply and demand situations of strategic minerals”. Land and Resources Information, 41, 1-3(2017).
[25] Choi Sungyeol, Nam Hyo On, Ko Won Il. Nuclear power development can no longer be low-key. Energy, 1121, 836-851(2016).
[28] J Wang A, S Wang G, S Chen Q et al. The mineral resources demand theory and the prediction model. Acta Geoscientica Sinica,, 31, 137-147(2010).
[29] Q Wang Y. Research on the ecological compensation mechanism of mineral resources development for the whole life cycle. PhD diss., Beijing University of science and technology(2019).
[30] J Wen B, H Chen Y, S Wang G et al. China’s demand for energy and mineral resources by 2035. China Engineering Science, 21, 68-73(2019).
[31] Association World Nuclear. World nuclear performance report 2017.. England and Wales: WNA.(2017).
[32] Q Yan, J Wang A, S Wang G et al. Survey of uranium resources and demand forecast in 2030. Mining Magazine, 20, 1-5(2011).
[33] Y Yang, J Wang, M Xu. Thoughts on the development of sustainable nuclear energy system based on fast reactor in China. Chinese Engineering Science,, 20, 32-38(2018).
[34] T Zhang H, R Tang J, Y Chen R et al. Prelinimary discussion on the integrated management of mineral resources, assets and capitals. China Mining Magazine, 23, 45-51, 75(2014).
[35] Y Zhao, T Ju M, L Shen. Current situation and countermeasures of mineral resources security in China. Resources and Industry, 13, 79-83(2011).
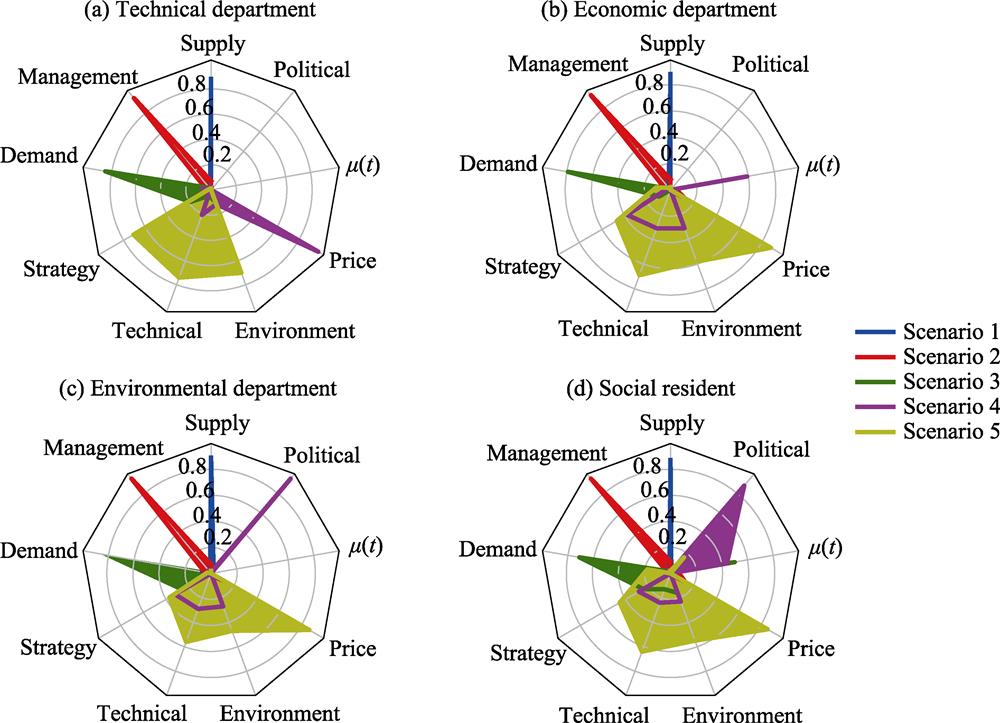
Set citation alerts for the article
Please enter your email address