KUANG Jin-jun, CHAI Yi, XIONG Qing-yu. Visual object tracking combined normal hedge and kernel sparse representation classification[J]. Optics and Precision Engineering, 2012, 20(11): 2540

Search by keywords or author
- Optics and Precision Engineering
- Vol. 20, Issue 11, 2540 (2012)
Abstract
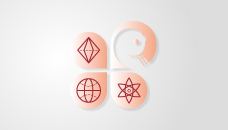
Set citation alerts for the article
Please enter your email address