GUO Li-qiang, MENG Qing-chao. Space Spectrum Classification Algorithm Based on Multi-label Shared Subspace Learning and Kernel Ridge Regression[J]. Acta Photonica Sinica, 2020, 49(5): 528001

Search by keywords or author
- Acta Photonica Sinica
- Vol. 49, Issue 5, 528001 (2020)
Abstract
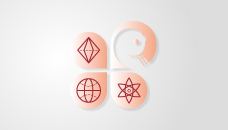
Set citation alerts for the article
Please enter your email address