Chunyan LI, Gengpeng LI, Jihong LIU, Dou LUO, Jiayi LIU. Analysis and Research on Spectral Confocal Displacement Measurement Method Based on GRNN[J]. Acta Photonica Sinica, 2022, 51(3): 0330001

Search by keywords or author
- Acta Photonica Sinica
- Vol. 51, Issue 3, 0330001 (2022)
Abstract
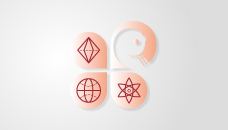
Set citation alerts for the article
Please enter your email address