Chun-fu HUANG, An LI, Fang-jun QIN, Zhi WANG. Temperature Error Modeling and Real-time Compensation of Fiber Optic Gyroscope Based on PSO-SVR[J]. Acta Photonica Sinica, 2019, 48(12): 1206002

Search by keywords or author
- Acta Photonica Sinica
- Vol. 48, Issue 12, 1206002 (2019)
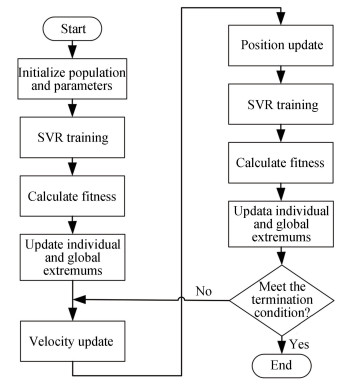
Fig. 1. PSO-SVR算法流程图PSO-SVR algorithm flowchart
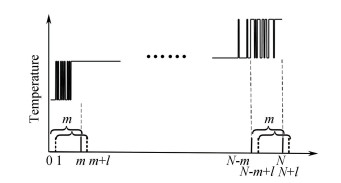
Fig. 2. 温度变化率实时获取Real-time acquisition of temperature change rate
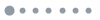
Fig. 3. FOG温度误差在线补偿FOG temperature error online compensation
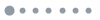
Fig. 4. 最小二乘补偿效果Compensation effect of least squares
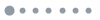
Fig. 5. RBF神经网络补偿效果Compensation effect of RBF neural networks
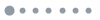
Fig. 6. PSO-SVR补偿效果Compensation effect of PSO-SVR
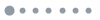
Fig. 7. 最小二乘法实时补偿效果Real-time compensation effect of least squares
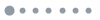
Fig. 8. RBF神经网络实时补偿效果Real-time compensation effect of RBF neural networks
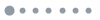
Fig. 9. PSO-SVR实时补偿效果Real-time compensation effect of PSO-SVR
|
Table 1. PSO parameters setting
|
Table 2. Best parameters for SVR obtained by PSO
|
Table 3. Comparison of results of threemodeling methods
|
Table 4. Comparison of real-time compensation results
|
Table 5. Operation time
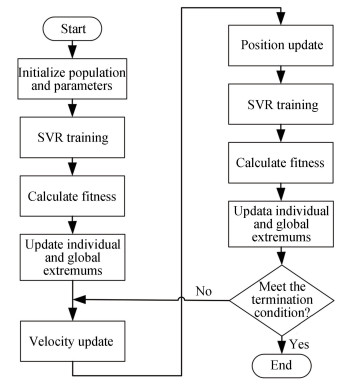
Set citation alerts for the article
Please enter your email address