Jie Xu, Hui Liu, Yue Shen, Guanxue Yang, Hao Zhou, Siyuan Wang. Point Clouds Classification and Segmentation for Nursery Trees Based on Improved PointNet++ Model[J]. Chinese Journal of Lasers, 2024, 51(8): 0810001

Search by keywords or author
- Chinese Journal of Lasers
- Vol. 51, Issue 8, 0810001 (2024)
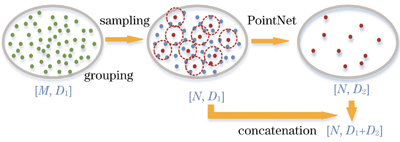
Fig. 1. Schematic diagram of feature fusion strategy
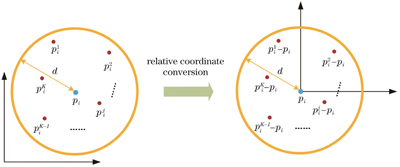
Fig. 2. Acquisition of relative coordinates of neighboring points for each local area
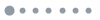
Fig. 3. Model combining coordinate attention mechanism and attentive pooling
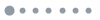
Fig. 4. Classification branch of improved model
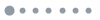
Fig. 5. Segmentation branch of improved model
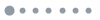
Fig. 6. Nursery for data collection. (a) Whole scene of nursery; (b) part of scene of nursery
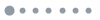
Fig. 7. Livox Horizon laser sensor and acquired point clouds. (a) Livox Horizon laser sensor; (b) acquired point clouds
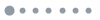
Fig. 8. Seven common kinds of landscape trees. (a) Osmanthus fragrans; (b) Malus halliana; (c) cherry plum; (d) Acer palmatum; (e) Chimonanthus praecox; (f) loquat tree; (g) Chinese holly
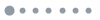
Fig. 9. Schematic diagram of segmented point clouds of seven tree species. (a) Osmanthus fragrans; (b) Malus halliana; (c) cherry plum; (d) Acer palmatum; (e) Chimonanthus praecox; (f) loquat tree; (g) Chinese holly
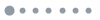
Fig. 10. Confusion matrixes. (a) PointNet; (b) PointNet++; (c) ours
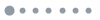
Fig. 11. Examples of visualization of segmentation results using PointNet, PointNet++, and proposed model (white boxes denote wrong predicted points). (a) Ground truth; (b) segmentation results of PointNet; (c) segmentation results of PointNet++; (d) segmentation results of proposed model
|
Table 1. Laser parameters
|
Table 2. Numbers of samples, point cloud groups, and groups in training and testing sets for different types of trees
|
Table 3. Software and hardware parameters for experiment
|
Table 4. Testing results of classification on self-made dataset
|
Table 5. Testing results of segmentation on self-made dataset
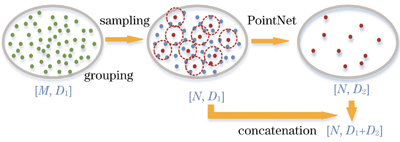
Set citation alerts for the article
Please enter your email address