Zhao Cong, Chen Xiaodong, Zhang Jiachen, Wang Yi, Jia Zhongwei, Chen Xiangzhi, Yu Daoyin. Coronary Lesion Detection Method Based on One-Class Support Vector Machine[J]. Chinese Journal of Lasers, 2017, 44(5): 504006

Search by keywords or author
- Chinese Journal of Lasers
- Vol. 44, Issue 5, 504006 (2017)
Abstract
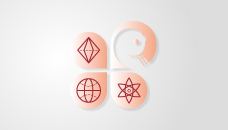
Set citation alerts for the article
Please enter your email address