LEI Jun-feng, HE Rui, XIAO Jin-sheng. Driving obstacles prediction network merged with spatial attention[J]. Optics and Precision Engineering, 2020, 28(8): 1850

Search by keywords or author
- Optics and Precision Engineering
- Vol. 28, Issue 8, 1850 (2020)
Abstract
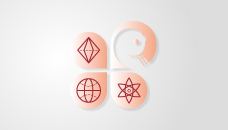
Set citation alerts for the article
Please enter your email address